How Generative AI and Data Portability Could Help Save Democracy
Chris Riley / Dec 9, 2024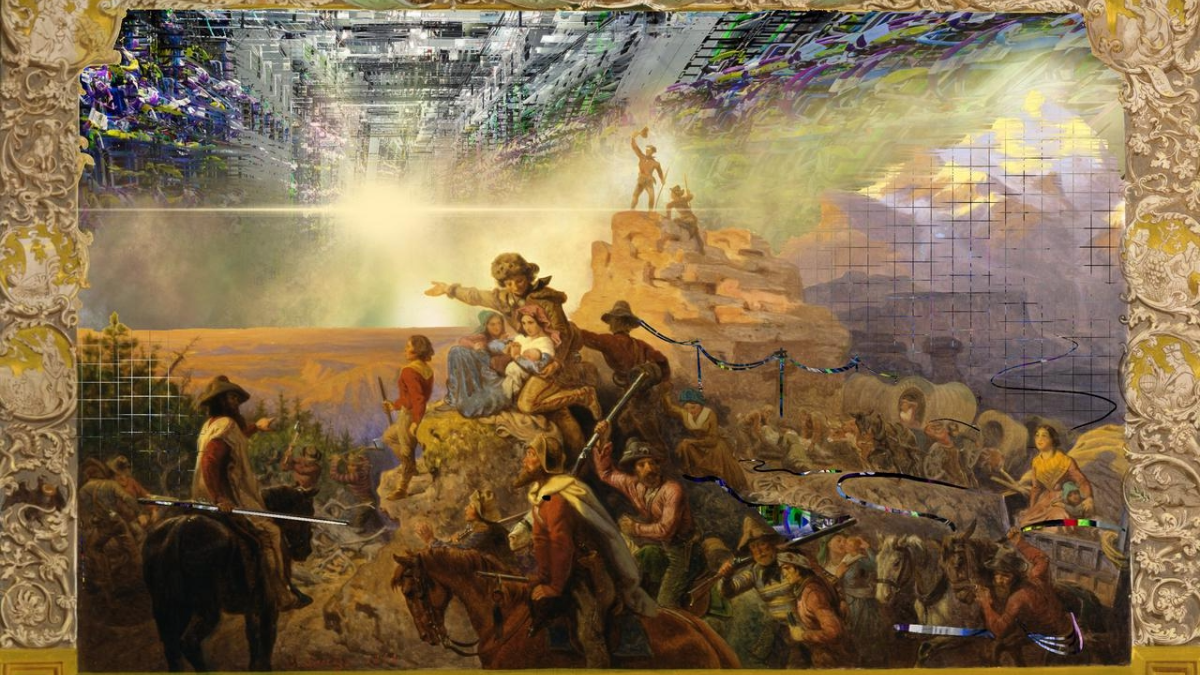
Hanna Barakat + AIxDESIGN & Archival Images of AI / Better Images of AI / Frontier Models 3 / CC-BY 4.0
On the heels of a dramatic Presidential election in the United States, I’ve read several different takes on what went wrong for the Democratic party. One element present in many of these is an information disorder. Most of what I saw acknowledged that fears of widespread misinformation and voter manipulation, exacerbated by the ease and quality of generative AI outputs and frictionless social media distribution, did not pan out. Or, at least, mis- and disinformation did not play the acute role that some feared.
However, the trajectory for our ability to ground a functional democracy in shared truth doesn’t look great. False stories about Haitian immigrants eating family pets and other similar claims were stubbornly persistent and, in some cases, produced harmful real-world outcomes, including violence. It seems quite possible that voters in 2028 will not be any better informed as to the contexts and consequences of their decisions.
I don’t think that is correct, though. My bet is that the average American voter will be better informed in future elections and that the basis of that information will be more consistent and more accurate compared to today. And that improvement in one of the core building blocks of our democracy will be due to generative AI.
Three assumptions drive this theory. The first is that people generally prefer low-friction sources of information. When someone has a question, they want an answer. They have lives, lives that are pretty darn hard in a lot of ways, lives that feel a lot harder now than they did in the 2010s as we all come to grips with the permanent consequences of inflation and the pandemic. We all sort of hoped those would be episodes (just as many did with the first Trump term) and that life at some point would return to “normal.” But as we know now, that is simply not the case. There is no normal.
This drive for immediacy is a human characteristic but not inherently a failing. Most people aren’t interested in all (or any) of the layers of answers, particularly given that reasoning and evidence build on other reasoning and evidence. (Anyone with a bright child will know that there is no end to a chain of recursive “why” questions in observing the patterns of the world.) Persistent normative judgments aside, it seems reasonable to assume that the lowest-friction sources of perceived truth will continue to rule the day.
My second assumption is that the lowest-friction source of truth is changing. Less and less will it be the digital spaces where people have historically gone for entertainment, news, and research, including social media, podcasts, and search. While those will still have a role, more and more, the immediate easiest source of answers to all of life’s questions will be ever-present generative AI systems.
I know what many of you are thinking. “The same generative AI that told us to eat rocks?” The outputs of the world’s most powerful generative AI tools are not perfect. And I am of the camp that thinks they’re reaching a ceiling. I don’t think generative AI is going to get significantly smarter than it is today, and to the extent large language models continue to train greedily on whatever data they can find, increasingly, that input will include earlier generations of AI output (which, after all, we did not successfully label as AI generated), undermining rather than improving the quality of the systems in the phenomena known as “model collapse.”
Nothing is certain. The analysis and prognostication around AI as a field have grown to the point where, much like in economics, you can find qualified professionals who have written extensive pieces supporting your position on any given issue, no matter what your position may be. But even if we do hit a plateau, the models we have today are incredibly smart and useful.
My daughter (age 9) was asked by her violin teacher to look into similarities between baroque architecture and the music of J.S. Bach. On a whim, I looked up the key characteristics of baroque architecture, which Architectural Digest identifies as monumentality, embodied experiences, and light. I asked the AI tool Pi how these apply to Bach’s music. Here’s what it told me:
1. Monumentality: Bach's compositions are known for their grandeur and complexity, even in pieces of shorter length. His fugues, for example, create a dense and intricate sound that feels monumental. The multiple independent melodic lines in his works add depth and richness, giving a sense of grandeur akin to the impressive façades of Baroque buildings.
2. Embodied experiences: Bach's music is known for its emotional depth and ability to evoke strong feelings in listeners. His compositions often feature dramatic contrasts, like shifts from major to minor keys, which mirror the theatrical forms and dynamic sequencing of spaces in Baroque architecture. A good example is the famous "Toccata and Fugue in D minor," which takes the listener on an emotional rollercoaster through its contrasting movements.
3. Use of light: In a more metaphorical sense, light can be represented in music through the use of ornamentation, trills, and other decorative elements that add sparkle and brightness to the sound. Bach's compositions are filled with such details, which could be likened to the reflective and glimmering surfaces found in Baroque interiors. A great example is the "Brandenburg Concerto No. 3," where the rapid violin passages create a shimmering, light-filled effect.
I’d say that’s pretty good. (No rocks are involved!) I'm not sure I’d call it PhD-level depth of understanding or anything particularly original, but as an exercise, it’s pretty good. And it illustrates my point about generative AI as a low-friction source of answers to life’s questions.
Not only are the tools useful, but the trajectory ahead is for them to have a greater presence in our lives. In the coming years, likely bolstered by fewer guardrails under a Trump administration (though one never knows), the massive amounts of money behind generative AI will push to see it embedded in more and more corners of the digital ecosystem. For better or (and?) for worse, these applications will be easier to access, in more ways, and for more purposes.
And that includes serving as a source of truth for, and on, the world at large. The collective generative AI outputs will continue displacing search engines, social media, podcasts, cable television, newspapers, and radio as the centerpiece (though never the sole source) of modern society’s shared knowledge base.
That will ring alarm bells. And there is plenty to be concerned about with a transition like this. But we should also acknowledge the possibility of benefits from technological change. My third and final assumption is that the shift to generative AI as a shared source of truth is an improvement on the status quo, quite possibly a major one.
Remember when conservatives tried to get xAI’s Grok to reinforce their beliefs, only to discover Grok declaring gender to be a social construct? Of course, with specific parameters and guidance, with built-in guardrails disabled in various ways, it’s easy to direct generative AI systems to produce unpleasant content in many forms. But by default and by design, they’re better than that.
It turns out that the bulk of human communication as chewed up and digested by generative AI systems is … pretty OK.
In their plug-and-play form, generative AI systems can serve today as a low-friction distillate of human wisdom, customizable with specific bodies of knowledge to allow them to be effective, shared sources of truth in specific contexts and communities.
Is this what truth means? I don’t want to fall too far down the philosophical abyss, but I’ll dip my toes in. I’m enough of a scientist/mathematician to believe certain things are objectively true. And I’m enough of a Christian to believe that God is, and is real and true, without infallible evidence to support it. But also, the victors write the history books, and in many ways, I would say shared truth is a social construct. Generative AI distills and presents that socially constructed shared truth, drawing on the reflections of, largely, humanity in the first 30 years of the digital era.
What does that mean going forward? When model collapse kicks in, the quality will decline. (Quite likely, it already is.) But LLMs can be calibrated to their optimal state, even rewound if necessary. The core understandings of generative AI systems, their baseline intelligence of the world, will stabilize a bit. That will make it harder for them to be gamified.
And, when generative AI systems are asked what they “think” of something happening in the news, something that occurred after their core training, where will they derive their “knowledge” to answer the question? From partnerships with established news outlets, which they’re already striking.
The lowest-friction source of truth is on a collision course with reputable news. As people seek answers, what they will find will be a digest of quality information. Certainly it will be an improvement on asking the same questions of modern-day social media.
An unintended consequence of the desires of venture capitalists and the technology industry to make generative AI pervasive will be a greater overlap in shared knowledge and understanding of the world at large among the voters in democratic countries -- and thus, a healthier democracy. The incentives seem to align here among all stakeholders involved:
- News organizations who want to find ways to have their work valued and consumed in the modern world,
- Technology companies who want products that are desirable to users and produce economic returns for their investments,
- Democracy advocates (and at least some in politics and government) who want to improve shared understanding of the world and resist misinformation and manipulation, and
- Everyday people who want to get about their lives without burden, but also, from time to time, have questions and want answers.
This isn’t a perfect plan. There are still some questions and conversations that just shouldn’t happen on generative AI. I wouldn’t necessarily consult it for, say, questions of legal ethics. And precise characterizations of a specific person’s past words or positions can be complicated. Also, it would be great if we, as humans, took the output of generative AI with a grain of salt. Reflexively assuming truth in anything digital is problematic.
Another major challenge is that the foundational knowledge base for these systems—the 30-year span of socially constructed shared truths—is deeply influenced by various forms of bias and discrimination, including those related to gender, race, and a predominantly Western perspective. The problems of bias in AI are felt far beyond the generative context, and targeted interventions will be needed wherever the nature of embedded AI perpetuates history’s many discriminatory harms. We won’t have much of a democracy if only some of us are able to be fully part of it.
Within these contextual caveats, though, I continue to believe there is room for hope here.
My assumptions could be wrong. Feel free to pick whichever one you wish to disagree with. (It’s probably the third, but do consider the baseline. Or maybe the second, on the grounds that generative AI will never really prove to be economically worth the electricity and compute cost—but the smaller form, more efficient, device-level models will become more of the focus when the top-level power plateaus.)
Nothing is certain in the modern era. Maybe the models will still be susceptible to gamification. Maybe enough people will become convinced that generative AI is the work of the Devil or something akin, and resist allowing them to become present in their lives, no matter the work of technology companies to make them easy and pleasant to use and omnipresent. But I see hope here, that advances in technology will end up contributing to good outcomes for society.
Where does that leave us? For me, it means that one of the most important things we need to focus on is preventing the concentration of power in individual producers of LLM technologies. Every bit of accountability that regulators or users seek to get from service providers will be easier if generative AI core technologies can be substituted on-the-fly without excessive cost or disruption.
Or, to look at that technology paradigm from the flip side, we get to the same outcome (more or less) if users and third-party service providers building downstream of the generative AI core technologies can port their customizations, personalizations, and history from one model to another. This doesn’t mean model weights or anything else proprietary to or embedded within the generative AI tool itself – just the pieces downstream of that.
The market for generative AI is still very early. It’s not too late to demand portability and interoperability as generative AI tools become more and more widely used. The first and most straightforward step? Make sure an individual generative AI user’s personal conversation history is portable, preferably transferable directly between services through an interoperable format. In the post-GDPR world, this is the least we should be asking for.
Related Reading
Authors
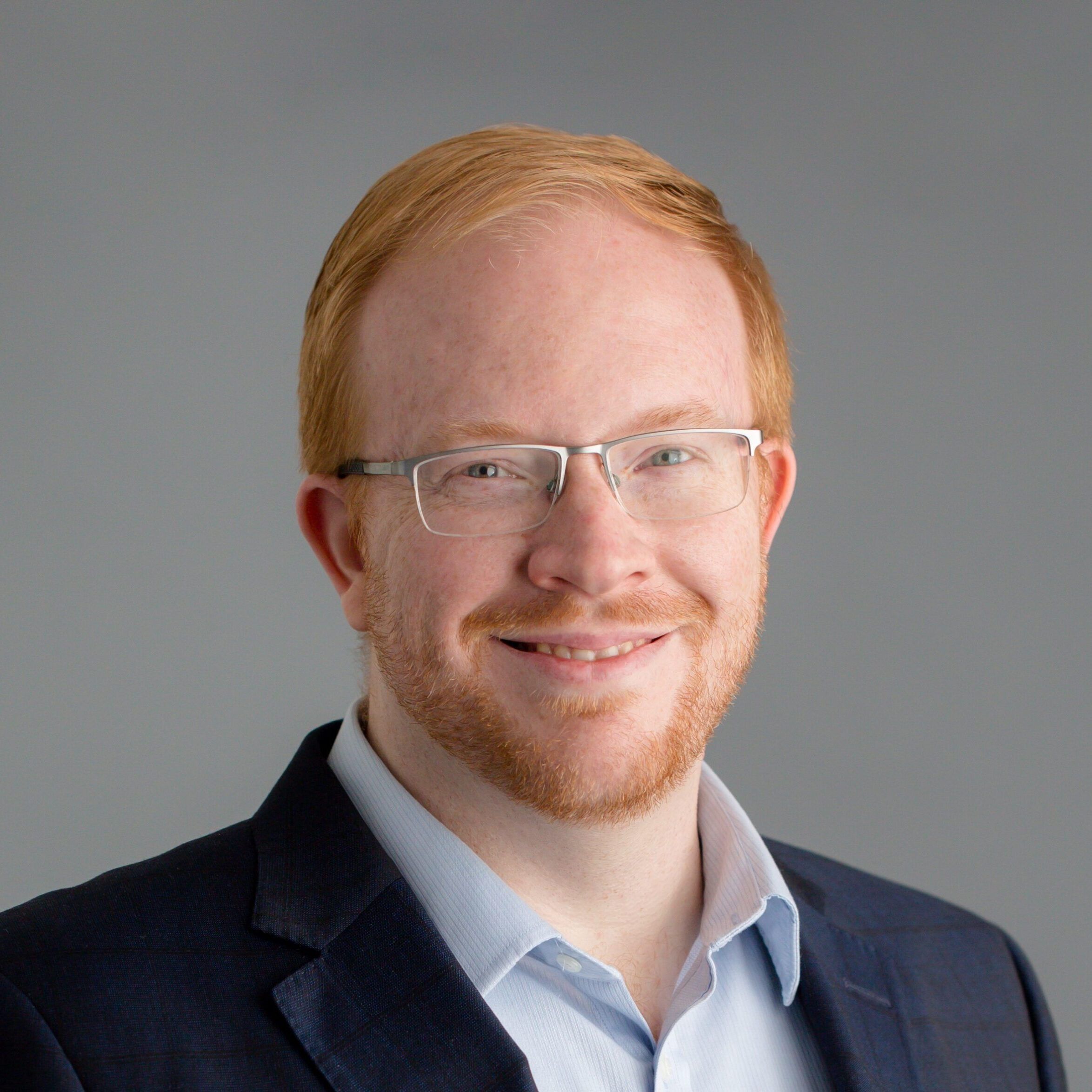