Four Ways to Ensure Ethical Experimentation with AI in the Public Sector
Martelle Esposito, Genevieve Gaudet, Kira Leadholm / Apr 25, 2024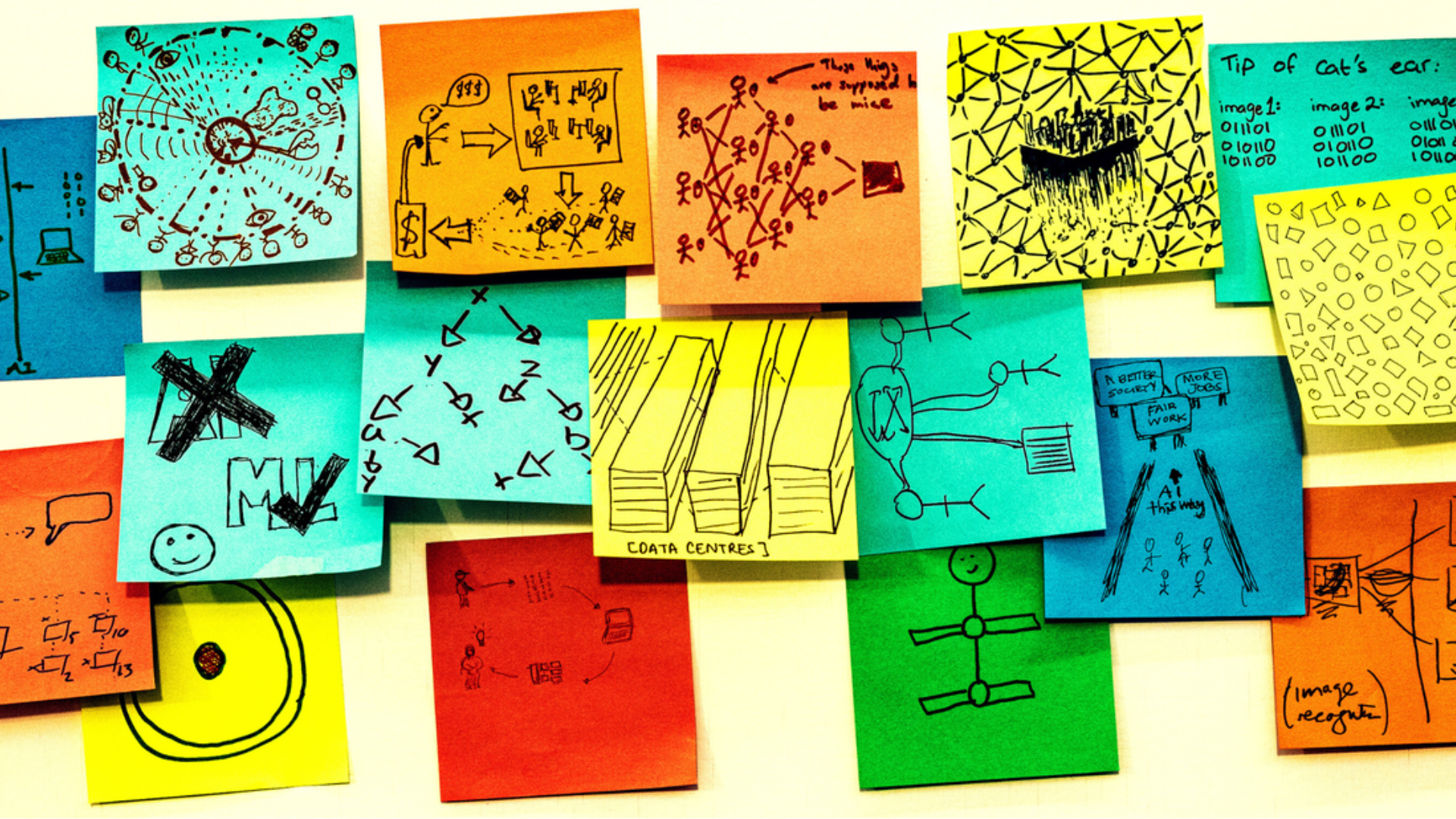
Rick Payne and team / Better Images of AI / Ai is... Banner / CC-BY 4.0
Applying for public benefits in the United States is a byzantine process that stymies even the most seasoned professionals. That’s partially why over 25 percent of people experiencing financial distress do not get help from a federally funded program. Artificial intelligence (AI) has the potential to demystify this process for applicants and for professionals who help people apply for benefits, often called navigators. However, AI must be deployed intentionally and ethically to avoid causing harm to vulnerable populations. In many cases, this means using AI to augment what navigators do, not using it to eliminate the human interactions that take place between navigators and the public.
Development teams can mitigate the risks associated with AI by building systems alongside those who will use the technology—often called human-centered design—and by testing new AI tools in low-risk environments, evaluating the results, iterating, and rinsing and repeating—also called agile development. This is consistent with some of the directives put forth by the Biden administration’s Executive Order on AI.
At Nava, a company that has helped some of the largest state and federal agencies solve highly scrutinized tech challenges, we agree with the Executive Order’s emphasis on using human-centered design and agile development to de-risk new technology. We’ve seen the repercussions of technology gone wrong, such as when we helped rebuild Healthcare.gov after its troubled launch. In that scenario, one website crashing meant that tens of millions of people couldn’t enroll in healthcare.
On the flip side, we’ve de-risked highly scrutinized government technology projects by practicing human-centered design and agile development. Based on our experiences, we believe that AI projects in the public sector should be approached no differently.
In partnership with Benefits Data Trust (BDT) and with funding from the Bill and Melinda Gates Foundation and Google.org, we’re creating, testing, and piloting an AI-powered tool that may help government agency staff and benefits navigators easily identify which families can enroll in key public benefit programs such as WIC, SNAP, and Medicaid. For this work, we’re exploring how the practices we’ve used in the past to successfully de-risk technology might enable us to responsibly implement AI models while improving outcomes for the public. In addition to providing immediate value for benefits navigators, we hope this project’s insights will inform AI policy and implementation.
We hypothesize that AI tools can decrease the amount of time that navigators of bureaucratic systems such as social workers, caseworkers, call center staff, or community outreach specialists spend on administrative tasks. This can free up their time to directly engage with families in need, reduce burnout, and even increase access to navigator professions. Our initial conversations with navigators and beneficiaries revealed that beneficiaries would rather invest the time required to meet with navigators in-person or virtually than apply for benefits remotely. This is because the time it takes to meet with a navigator is perceived to be less burdensome than the mental energy it takes to fill out an application. In short, having someone on-hand to tell you whether you’ve completed your application correctly is reassuring. These findings are consistent with those from the Biden Administration’s Having a Child and Early Childhood Life Experience project.
Despite the necessity of navigators, it’s hard to keep people in the profession due to low wages and long hours. One study found turnover among child welfare workers, who often act as navigators, to be as high as 35 percent. By reducing much of the manual labor required of navigators, AI has the potential to promote a better work-life balance and keep qualified people in the workforce longer. For example, an AI-powered chatbot might answer common questions about public benefits, enabling call center specialists to redirect their attention to cases that need more specific support. This would also help eliminate the hours-long wait times many people experience on hold with call centers.
What’s more, AI may actually create jobs in benefits navigation. The process of obtaining public benefits is notoriously complex, which means the barriers to entering navigator professions are quite high. This has contributed to a nationwide shortage of social workers—who often serve as navigators—despite the fact that more and more people need their services. If AI can help make sense of the system’s more convoluted aspects, navigators can focus on human interactions. This should also make it easier to train people who already interact with communities, such as staff at libraries or non-profits, on helping people access benefits.
We recognize this tool’s potential to improve outcomes for navigators and for the public, but we also acknowledge that there are many potential downsides to implementing generative AI in the public sector. That’s why we’ve taken steps to ensure this project is responsibly designed to mitigate harm while providing much-needed insights into the potential applications of AI in public benefits.
1. Iterating in a low-risk environment
One way to iterate in a low-risk environment is to test new AI models with people who are experts in the realm the tool will be implemented in. These experts can give feedback on the technology’s accuracy and recommend improvements. In our case, our end-users are navigators who also happen to be experts on the benefits application process. Given that, we will test our tool with navigators, allowing us to compare the tool’s outputs and recommendations against those of experienced specialists. Importantly, these tests will never affect vulnerable populations, and they’ll yield insights into how effective the tool is in reducing administrative burden for navigators.
The test-with-the-experts method has worked in other industries. Take for example AI in healthcare, another sector that’s extremely complex and high stakes. An AI model suggesting an erroneous diagnosis or treatment plans could kill a patient, but a lower risk application of the technology is using it to reduce the hours of paperwork medical professionals do daily. Like navigators, medical professionals experience high levels of burnout and turnover, in part due to the long hours they dedicate to patient paperwork. That’s why some doctors have been using generative AI that records patient notes for electronic health records and delivers after-visit summaries to patients. Importantly, doctors compare the AI’s output to what was said during the visit before passing the summary to the patient. This application of AI-powered tools has freed up nearly two hours per day for doctors who use it.
2. Engaging end-users in research
When incorporating AI models into public services, it’s also crucial to understand the needs of those who will eventually use the tool. Failing to do so could result in a product that doesn’t center the people who will use it, contributing to negative public impacts like harmful biases. One of the best ways to go about this is to conduct user research, which can help build trust in new technology and in public institutions. In our case, we’re continuously engaging with navigators and industry experts, such as representatives from The American Public Human Services Association (APHSA) and the Center on Budget and Policy Priorities (CBPP), through a formal advisory group. We are also engaging program beneficiaries to understand their experiences with benefits navigators and applying for benefits on their own. We’re doing so because ultimately, the tool should improve experiences for navigators and for the beneficiaries they work with.
The UK’s Government Digital Service (GDS) is also leveraging user research for building AI tools as it pilots GOV.UK Chat, a generative AI-powered chatbot that answers questions about anything on GOV.UK, the UK’s one-stop-shop for government information. GOV.UK contains over 700,000 pages on everything from visas to national holidays to legal processes, so it can be hard for people to find the information they need. Enter the chatbot.
GOV.UK Chat culls published information on GOV.UK to help people find the answers they need. GDS is taking a phased approach to building the chat bot, meaning they build a prototype, test it, evaluate the results, and then prototype again. The first three phases of development were internal, which enabled GDS to de-risk the chatbot before it interacted with the public. Next, they tested it with a group of twelve people, and then 1,000. This user testing resulted in a 65 percent satisfaction rate with the chatbot and several insights GDS will incorporate into their next round of iteration.
3. Training AI with diverse datasets
It’s well-documented that AI models can reinforce racism, sexism, and other harmful biases if trained incorrectly. That’s why it’s important for developers working on public sector projects to train models with diverse datasets to prevent bias. When choosing training datasets, developers should ask if certain groups are over-represented or under-represented. Additionally, developers must consider how they’ll maintain data privacy if they’re dealing with personal identifiable information (PII).
We will leverage datasets from BDT’s Benefits Centers, or call centers, which have helped more than a million people apply for benefits in states across the country. This will help us train our model to recognize who might be eligible for benefits based on underlying factors, to capture the empathetic tone that navigators use, to understand what questions navigators ask about uncommon cases, and to conduct targeted outreach efforts. We won’t have access to PII, so our project will allow us to explore how we might build intelligence while maintaining data privacy.
4. Defining how to measure success
Even if a project has ticked all the above boxes, it’s impossible to prevent every negative outcome of a new tool. Teams experimenting with AI tools must be able to gauge which negative outcomes are permissible and which are not. This will maximize positive outcomes and minimize negative ones. For example, it would be a major issue if our tool was incorrectly suggesting people are not eligible for benefits when they are. On the flip side, it’s not as big of an issue if our tool falsely suggests someone is eligible for benefits. In this scenario, we’ll vet those deemed ineligible with caseworkers to prevent erroneous determinations of ineligibility.
In order to navigate these tradeoffs, teams must define success metrics and decide how they’ll measure them. For our project, we’re measuring the following outcomes by engaging subject matter experts and beneficiaries.
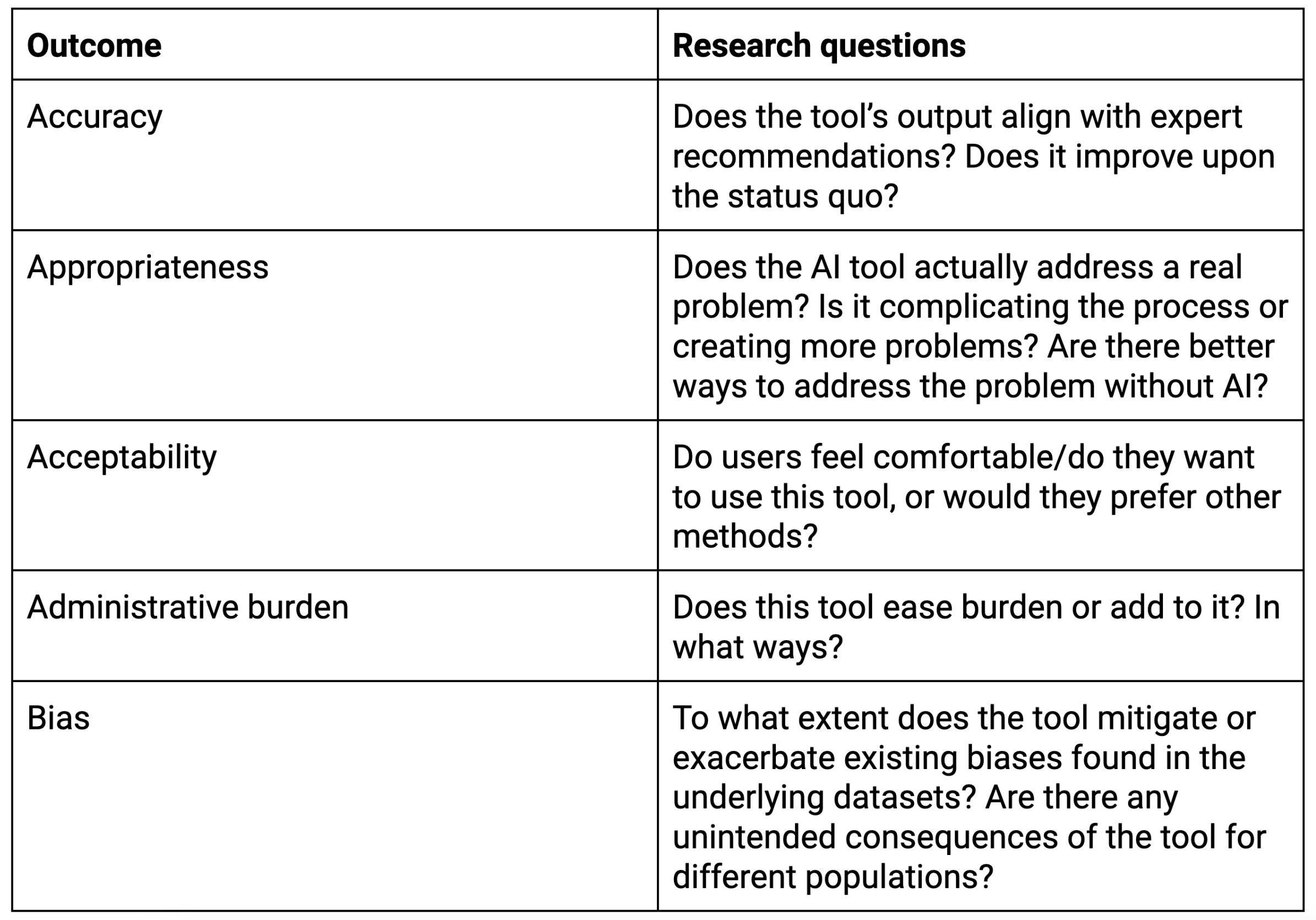
Desired outcomes and research questions. (Administrative burden as defined here.)
Conclusion
Depending on how they are built, AI tools have the potential to revolutionize public benefits delivery or harm millions of people. If an AI model augments the work of navigators, as the AI scribe supplemented what doctors do, we anticipate that we can address the shortage of navigators while helping eligible people acquire public benefits. Developers must also get feedback from the people who will use the service and iterate based on that feedback, as GDS did with its chatbot. Any AI tool for the public sector must be trained with diverse datasets to prevent harmful biases. And finally, teams can navigate difficult tradeoffs by defining how they’ll measure success. By following these practices, we can ensure that the output of an AI model does not adversely affect vulnerable populations, but empowers them.
Authors
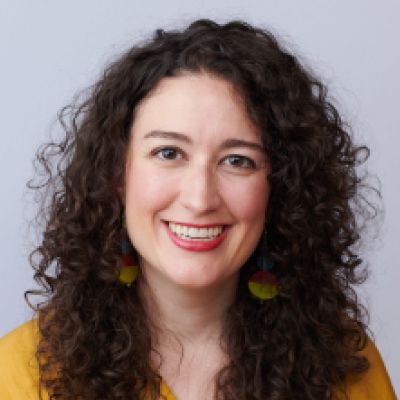
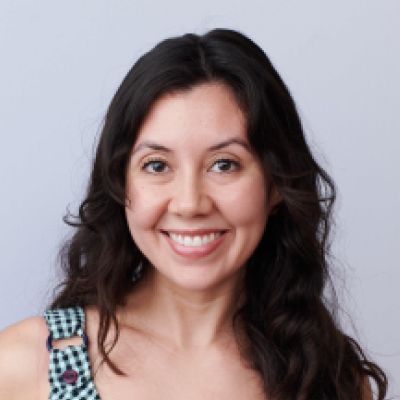
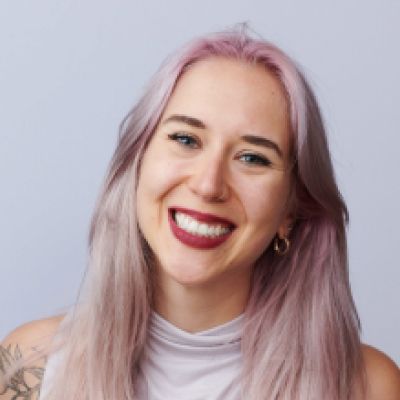