Finding the Humanity in an Automated World
Justin Hendrix / Jun 18, 2024Audio of this conversation is available via your favorite podcast service.
Madhumita Murgia, AI editor at the Financial Times, is the author of a new book called Code Dependent: Living in the Shadow of AI. The book combines reporting and research to provide a look at the role that AI and automated decision-making is playing in reshaping our lives, our politics, and our economies across the world.
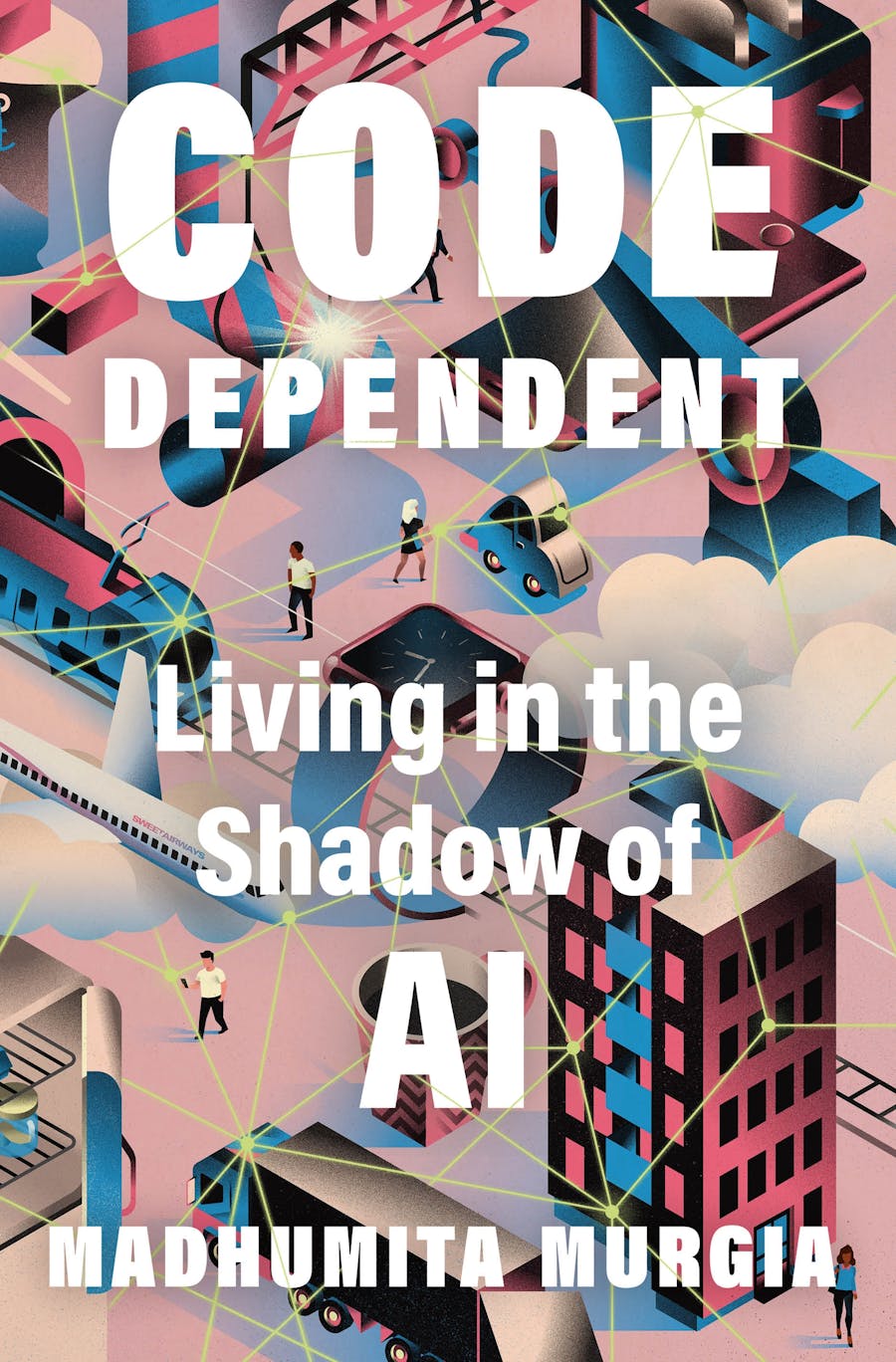
Code Dependent. Henry Holt & Co, June 18, 2024.
What follows is a lightly edited transcript of the discussion.
Justin Hendrix:
Madhumita, it is so nice to speak to you about this book, which is getting lots of great reviews. The Times of India calls it "a globe-trotting work of reportage." Chris Stokel-Walker, who we just had on this podcast talking about his new book, calls it "essential reading." You are already on the list for Women's Non-Fiction Prize for the book. So I think it's going well even before it's on sale in the United States. How long did you work on this book?
Madhumita Murgia:
Thanks for having me, and thank you for the intro. I've been writing about AI for more than 10 years now, but I think the stories really morphed and changed. And the book itself took me about 16 months or so to write. But before that, I had conceived it through a proposal. So really it was a two-year project. And over that time, the story has dramatically evolved also, which made the writing of it pretty fun.
Justin Hendrix:
Tell me a little bit about your role at the Financial Times now. What is considered within the remit of being the first AI editor there?
Madhumita Murgia:
I took on this particular role of AI editor last January, in 2023. And yeah, I'm the first person to do this role. And really the goal was for us to have a cohesive global strategy of how we covered AI and really what we were going to write about. And this was because me and two or three others at the FT, Richard Waters, who was based on the West Coast, and John Thornhill, the three of us were always fascinated by AI and had written about it for many years.
And so when things started to heat up towards the end of 2022 and ChatGPT burst on the scene, we felt that... Actually just prior to that, we made this decision which was made by our editor Roula Khalaf, to say that there is going to be this huge mainstream interest in AI and we have the experience and the expertise of having covered it for a while so we can come in and be the rational voices here, that was the hope, to look globally and say what is actually worth covering? What are the important parts of this story? Can we explain some of the more complex underlying technology that sits beneath this story? And to join the dots, not just in the US, but look at it from this global angle, looking at China and Europe and the Middle East and India and elsewhere.
So yeah. So the remit is to write about the stories that most fascinate me in this area, but also to work with our correspondents around the world on what is worth doing in the AI space in their geography or in their industry so that we have this informed and cohesive view that comes through our coverage.
Justin Hendrix:
This book does take us around the world. And I thought I might just prompt you with a couple of place names and see if you can describe for us what you learned in those places as you went about writing this book and also in your reportage generally. Maybe let's start out in India. Let's start out in Maharashtra. What did you learn there at a doctor's clinic?
Madhumita Murgia:
The goal was to step outside, travel around the world and understand how AI was changing not just industries or jobs but really people's lives. So this is the story of ordinary individuals who could be you or me. And in Maharashtra, I spent time speaking with Dr. Ashita Singh. So she's a doctor, local doctor there who serves about her clinic, serves about a million people, mostly from an indigenous community in India there. And she is trialing out and working with an AI system that can diagnose tuberculosis just by looking at an image of a chest x-ray or a scan. And it's fascinating because you can just immediately see how this could bridge this gap that we have with access to healthcare.
And it doesn't just have to be in India. You see this in the United States, you see this here in the UK. You have huge swathes of the population communities that can't access quality healthcare. But if you could have an app like this that's cheap to scale up, you actually can filter very quickly those who need it the most and those who require medical care. So partly, it was to understand the potential of an AI diagnostic tool, but also to go beyond that. Because this whole book is about fighting against the black and white perception of AI, is it good or is it bad? It's such a polarized view.
But the reality is the entire gray area in between. It's good intentions that go wrong. It's broken AI systems with broken human systems that fail people rather than the technology itself. So in that chapter in India, I was also looking at how this might go wrong, how this might impact the agency of human doctors, how does it change the way humans do their jobs, and how we as patients rely on other humans, which is doctors and nurses versus AI systems. And asking this question of whether we might create a two class system where you have those who can afford human care, which might become the premium, and those who are left with AI care, which might be the more flawed, general purpose tool.
So it was trying to look at what reality we're already living in and what to be aware of when it comes to automating healthcare.
Justin Hendrix:
I want to fly across the world and ask you about another place and another person, Ziad Obermeyer in California.
Madhumita Murgia:
I went right across and spent time with Ziad in Berkeley. And he's really interesting because he's both an emergency room physician trained at Harvard in Boston, but working out in Berkeley. But he's also a machine learning scientist, so an AI researcher. So he really understands both sides of this coin when it comes to AI and healthcare. And what was really interesting to me about Ziad's work was that he uses his work in AI to actually plug gaps that we have today in healthcare to solve medical mysteries or biases that we see today. And so an example of that is, he used machine learning, which is a subset of AI, to actually understand pain, this feeling that is so universal to all of us but that's so subjective. Each of us feels pain differently. It's very hard to measure it, to standardize it.
And in particular in the United States, often African-Americans report higher levels of pain than what doctors seem to see in their scans or through their medical notes. And so you have this gap of understanding where people often attribute it to social issues like racism in the system or other sort of nonmedical issues. But really what he found, he used AI on a set of self annotated scans of a knee which the patient themselves had annotated pain levels, and he managed to train an AI system that was much better at predicting pain in African-Americans compared to human doctors.
And so now, they can go and try and figure out what is it that's biologically different about the knee tissues of people of this ethnicity compared to Caucasian patients, rather than just try and explain away the differences. So I thought that was really interesting example of using AI, flipping it, not just to replace humans or do what humans do, but actually go beyond what we've been able to find or beyond what our biases prevent us from discovering and helping to solve these unsolved problems and mysteries.
Justin Hendrix:
Let's go to one more location. Let's go back to Kenya. You take us to a busy cafe in the Kibera neighborhood of Nairobi and you talk about outsource firm that we've both talked about on this podcast and also I had former employees on in past. Sama.
Madhumita Murgia:
I opened my book in Kenya. And for me, the reason to start there was to... The goal, the impetus for this book is to peel back the magic around AI. And we have this sense that it's this automated technology, this black box that it's very difficult to get inside of and that it's only really in the purview of the select few experts, and it's very difficult for the rest of us whether we are regulators and policymakers or people in the workforce or even doctors to understand what's inside it. So we are very locked out of the conversation. So for me, the goal of bringing human stories to the fore and talking to ordinary people about how their lives were changed was to say, "This is your story too, and this can be your conversation."
And the reason I started in Kenya with data labelers, who I call the data laborers, was actually to start at the beginning of the pipeline of AI where AI really starts being built, and to explain that this isn't a self-learning technology that you just set loose on some data, but there are actually hundreds of thousands of humans who sit in factories and label data so that AI systems can perceive them, can analyze them, right? Whether that's the self-driving mechanism of the Tesla car or whether that's e-commerce, categorization systems. All of these companies and products are customers and clients of outsourcing firms like Sama, like you mentioned, but others too in the Philippines and India and elsewhere.
And without these laborers, we wouldn't have the so-called self-learning AI systems, deep learning systems that we rely on today or that we are all using. So I started out in Kenya to, again, there, it was to push back against just the pure dichotomy of it's bad to outsource and they're being underpaid and ill-treated. I wanted to understand what changes these people experienced to spend some time in their homes and to really understand the impact of such a job on their lives. And I think, for me, the takeaway was that, yes, there have been some positive changes in these communities. People who were previously doing manual labor or domestic work were able to do this kind of digital job with a lot more dignity, with minimum wage, with sick pay and so on, able to put their children through school and pay for their parents' healthcare.
But at the same time, there was a ceiling that they hit beyond which they didn't have agency to question the work they were doing. There was opacity. They didn't know, in many cases, who the client was that they were working for. Many had to sign NDA, so they were never allowed to share with their family, friends or even lawyers who they were working for. And then of course, the economic impact, which is, yes, they were being paid the local minimum wage, but in no way was their lot in life moving up, right? They were not seeing the same economic wealth and the huge upside of AI technologies that we have been promised and that we promise everybody around us.
We're saying this is a technology that's going to create billions in wealth, that's going to make all our lives easier and productivity is going to grow, but for the people helping to build it today in the developing world largely, their income is still capped at $4 an hour, whatever that is. And they're not seeing the huge upside of AI technologies as yet. So that was the story that I was trying to understand better and look at how people are fighting back as well and finding their own voices and their own agency to fight for their own rights within this labor context.
Justin Hendrix:
So the book takes us to many of the places in the world and to many other individual stories, Amsterdam, where we learn about algorithmic lists and predictive policing, onto Argentina where we learn about the interaction between AI and public services, public health. Is there a particular place that you went to that rings out in your mind when you think about the process of putting this book together? Is there a mental image in your brain that you keep coming back to?
Madhumita Murgia:
What's fascinating is that you would expect that it's some of the more unusual, unexpected places that stick out because you don't necessarily expect to have AI technology in Salta, which is a small town in Argentina, or in rural India, for example. But I think for me, what sticks out is how universal many of these stories are. It's not specific to Salta or to China or to Pittsburgh. When I go and talk to the people here, you can pull out the strands from each of these stories and it applies to us globally. And I think for me, the theme that came up again and again was how techno-solutionism is failing us, right? This optimistic idea that technology can solve any human problem. It can fix healthcare, it can fix jobs, it can fix education.
There's just this optimism that's been seeded either by tech companies, but also throughout society because we are looking for solutions to these complex issues, energy, climate change. But really when we apply technology in these very messy human scenarios like trying to predict crime or trying to decide who should get public services or benefits from the government, it falls over again and again because we lose the humanity at the heart of it. We expect the computer to spit out the correct answer. We tend to trust it more than we should because of the tendency to be biased towards computers over humans.
And that's how this harms those who are at the sharp end of the technology because these systems do make mistakes. They're predictive statistical engines, right? They're never going to be perfect. They're not calculators. And when they do make mistakes, there's nobody there to catch it. And so those errors start to multiply and scale up in a way that they wouldn't if it was human. And those who are often getting hurt in all of these scenarios around the world are those who are already vulnerable and marginalized, as you would expect with any technology or new policy that's rolled out.
Justin Hendrix:
I do want to ask you about your own view of technology. You talk about your transformation from the time you were a wired reporter, perhaps almost institutionally programs to look for more optimistic takes on tech being born of Silicon Valley and its particular portrayal of the future. What's your relationship now to techno optimism? How do you think about Silicon Valley's version of the future? That's something we've talked about on this podcast quite a lot. The extent to which that's a constraint that so many across the world are working within. It's very hard to see outside of.
Madhumita Murgia:
Yeah. No, it is really interesting. Because I have evolved, I think, in my thinking, which is, I was training as a scientist prior to being a journalist. So I was an immunologist, a graduate student. And so I was naturally prone to believing in the power of cutting edge science and technology to solve problems and to bring solutions. And as you say, wired sees the world or did at the time through very rose colored spectacles when it comes to amazing new innovations and technological magic. So I was prone to seeing things that way.
But I think where I am today is much more of a realist when it comes to AI. So I wouldn't say that I've gone over to the side of the pessimism and saying that this is terrible for the world yet, but I feel very realistic about the opportunity than also about the harms. And I think that a big part of it is obviously the tech itself and how much hype there is around it and how quickly we are rolling it out. But as you say, it's also this question of the optimism that we see coming out of the creators of this technology who often aren't seeing the very real world harms that it's already causing.
Instead, we're having a conversation about a far future where AI might wipe out humanity. And we seem to be much more comfortable having conversations about existential risk and long-term AI safety to humans than looking at the reality of what's happening today. How is AI already failing today? Who is it harming? How can we fix the basic accountability issues around it? We're avoiding that conversation. And that's largely because of this tendency, I think, of technological creators to overlook those messy human issues and problems and to want to look beyond.
So I do think that we need to... That's why we need more people in the conversation. You need sociologists, you need philosophers, but you also need teachers and doctors, those whose lives and jobs are going to be changed through AI, to have a voice in saying how they want this to be shaped and how they want it to shape their work too.
Justin Hendrix:
So at the end of the book, with some humility, you say these are not quite as grand as the Rome Call or the Bletchley Declaration, but you offer 10 simple questions that you say are carved out from my interactions with people who think deeply about AI, questions that may help protests simply understand AI technology better. Just describe. We don't have to go through each one, but can you describe these questions and this framework that you've now created, and are you using it in your work?
Madhumita Murgia:
The goal was... It was actually inspired by... My husband is a quantum physicist, or was. And he would talk to me about the DiVincenzo criteria. It was just a simple set of... I think it was seven criteria that had to be fulfilled in order to build a quantum computer. And this was done decades ago, much before there was ever even the idea of a practical quantum computer. But the point was that when you did build one that you could scale up, it needs to satisfy these specific criteria. Very simple, but still in use today. And that's what I wanted to crystallize out of my reporting, which was to say, "Here are 10 simple things that if we ask of ourselves and of one another and of those in charge, we can start to actually create a world where we can live alongside AI in a way that we're happy, where we are not harming those who are most vulnerable."
So this includes things like what value we should put on to the data labeling industry, for example. What should they be paid? What's a fair wage globally for people who are participating in this as that will continue to grow? But also slightly different but related question of what is the value of the data we have created, whether that's as writers or podcasters, actors, musicians? This is the data, this is our creativity that is now being used to train the new generation AI systems largely for free, right? It's been scraped off the internet. So the question then is, how do we value that data? How do we compensate the humans who've contributed to the building of these AI systems rather than cut them out of the process?
But then there's also questions of what happens when you implement these systems? Who's in charge when AI systems start making decisions about who should get public benefit, who should get extra care in a hospital scenario? Who should get bail instead of jail? Who is overseeing the outcomes of these systems? Who holds the pen at the end of the day so if things go wrong, you can appeal? And also the question of, should we have a human alternative? Is there a human that I can appeal to if I don't feel that this automated outcome is correct?
So a lot of it is really thinking through how to maintain the dignity of human beings within a world that's going to increasingly start to get automated around us without actually pushing away the technology and turning a blind eye to it or pretending it doesn't exist. So how can we include it in our society, but in a way that's acceptable to all of us from a ethical, purely ethical and moral standpoint? What do we think is a fair and dignified way in which to live alongside these? So it sits above the question of regulation and policy, but I think should help inform that as well.
Justin Hendrix:
I want to ask you about just the concept of unintended consequences. Because it seems to me like one of the things we're dealing with so many of the products that are being rolled out from big companies, even Google, OpenAI, et cetera, is this idea that we should just accept that there will be some unintended consequences. There are going to be some racist material that will come out of your generative AI system, or there's going to be some bias built into this thing. At what point do we, I don't know, have to regard unintended consequences as certainties and really look at the executives who continue to hide behind that idea of unintended consequences as disingenuous. It's apparent these systems will produce these consequences. So if you roll them out, they're not unintended at all, are they?
Madhumita Murgia:
Yeah. It's a really good... And this is actually one of my 10 questions, which is, can you know for sure that this isn't breaking any rules that you would have for other devices or other systems that you've rolled out in your work or whatever context? And if not, then why are we rolling this out at all? If you don't know for sure that this diagnostic AI system isn't going to kill people and if you can't be sure, then why are we putting it out to begin with or what are the guardrails in place? But yeah, as you say, we know that there will be unintended consequences, and that's partly because we don't actually have a full sense of how these systems work when we talk about a black box. That is partly true. Right? We understand what data inputs go in. We can adjust the weights of the system so that variables are weighted differently. And you can see what comes out the other end. So you have some way of manipulating and maybe a little bit of transparency.
But at its core, inherently, deep learning technology is finding patterns and predictions in a way that humans can't. That's why they work well. So we are never going to be able to say, "X. This is how we get from X to Y, and here's an equation for it." Which is why I think the context of where they use matters so much. If the outcome can change somebody's life and affect the job they do or their health or something very crucial to a human life, then we have to be far more careful than if this is a tool we're using at work to help brainstorm ideas or summarize a meeting that we did or whatever it is. So the context matters.
And I do think that it's not so much letting the tech companies hide behind it. I think there's a lot... We just need to be much more educated, more broadly as a society. And that includes CEOs and boards of other big corporations that are starting to rapidly roll out these tools to say, "This isn't a search engine. This isn't a question answer engine. And the context to use this is where the downside is low. So you should use it where you can brainstorm or come up with ideas or trends. But if this is something that's crucial to the working of your company or is going to deeply affect an individual, then until you know for sure how to audit the systems, it shouldn't be touching those areas."
And I think, yeah, you are right about the fact that we don't know how to audit the outcomes. And we allow tech companies to say this over and over again, and it's, as if that's good enough. But I think that there needs to be a lot more investment into even the science of auditing it, which I think now there's a bit more of that being done by regulators in the US and the UK, for example, where they're saying we need to figure out how to evaluate these systems, how to tell when they're going wrong, how to minimize the errors. And without that, they can't just touch every job and children and every part of the population.
It's tough though because it feels like the genie's out of the bottle when it comes to adoption. This is being rolled out through Microsoft, Google Meta, which touches billions of people already through their products. But I think it's the more we talk about how this is hard to evaluate, hard to audit, the better we are educated and armed when things go wrong, which they will.
Justin Hendrix:
Almost feels like to me, to some extent, even responsible actors are almost in a prisoner's dilemma when it comes to artificial intelligence. It's, "I have to experiment with this technology and roll out applications before someone more irresponsible than me does. Because that's what I'm being told essentially, that I'll be trampled over if in fact I'm not the person who figures out how to apply this technology. Whatever I'm doing, someone will do faster, cheaper, and possibly maybe at some better threshold than I'm able to do." I don't know. That seems like a false choice to me, but seems to be where we're at.
Madhumita Murgia:
Yeah, there's definitely a race dynamic, particularly in the last two years. I think ChatGPT kicked it off, again, an unintended consequence, I think, of that system. Because my sense was that, when it was rolled out, the technology already existed, but not in that consumer wrapper. And even the people building it really couldn't predict how popular it would become with the average consumer and how they would take it and run off with it and come up with all these different users for it. But that was the impetus for all of the other companies in this space who had been building these systems for years and years to say, "Oh, it looks like the public is ready for this. So let's all start rolling out our versions of these products."
And as you say, this also a interoperation race dynamic has definitely emerged between Microsoft, Google, OpenAI and Google, Apple coming in with their view of things, Amazon will join soon enough. And it's very difficult to keep rationality and let's go slow with this and at the heart of that when so much money is riding on it. So I think it's not necessarily one company or even one person, but there is definitely a race dynamic that's emerging. And this has now gone from being just a Silicon Valley race dynamic to also becoming a geopolitical issue with China and the US and we need to get there quicker than they do, which again pushes forward the frontier on this stuff. Yeah.
So I think we are trapped now in a situation where there's a lot of private money riding on this and a lot of expectation and hope from what these products will bring.
Justin Hendrix:
I don't want to try to get you to tell us the moral of the story, but you do end up in an interesting place. At the very end of this book, you take us to the steps of the Vatican, to the Pope's residence, and you're walking along with a rabbi who's concerned with tech issues. Why did you end the book there? Should I take a message from that?
Madhumita Murgia:
It's funny. I've seen a few reviews here where people are like, "Eh, that is not where we expected to end up." At the end of this book, traveling around the world and talking about AI, I went to the Vatican to witness this inter-religious, interdisciplinary summit, which brought together leaders from the Jewish community, the Pope, of course, and the Catholic community, and also the Islamic community, and alongside tech companies and governments. So it's just really interesting cross-cultural and cross-disciplinary meeting of minds. And the goal was really to figure out what are the moral lines and the limits we want to draw around the technology.
And the reason I went there rather than just neatly summarizing everything from the book is, I did think it was an interesting metaphor about what has happened with religion. And in fact, the rabbi says this to me at the end that we have made huge mistakes as religious leaders and communities over the years, and much of that is because of this question of power. Power is what corrupts. It's what leads us to hurt and bloodshed and violence and war. And the feeling was that this is where we are with some of these corporations today where there's such a concentration of power. And yes, individually, people might be trying to do good things. Nobody's evil or trying to hurt any individual community or whatever.
But because of this huge, not just concentration but also inequity of power, where even governments struggle to reign in what tech companies are doing, where countries all over the world are dependent on a very small pocket of the world for these systems, for much of their infrastructure, there's a worry about the follies of that power and what might happen if you don't step back, have some humility, and consider this to be a societal issue and not just a tech product that you're going to roll out and implement.
And so for me, it was just taking a step just out of this whole magnifying glass of AI and thinking about it more broadly is what happens if we implement a very powerful technology built by very few without the voices of other communities and people with other types of expertise reflected in the building of it, especially because the technology is, it does reflect the values of our society because of how it uses data that's trained... it's trained using data produced by us, and it's affecting decisions made by us in everywhere from employment and education to healthcare. So it does actually have values baked into it and biases and so on. It's even more crucial for us to see this from just outside of the tech bubble.
Justin Hendrix:
This book is called Code Dependent: Living in the Shadow of AI. Madhumita, thank you so much for speaking to me today.
Madhumita Murgia:
Thanks for having me.
Authors
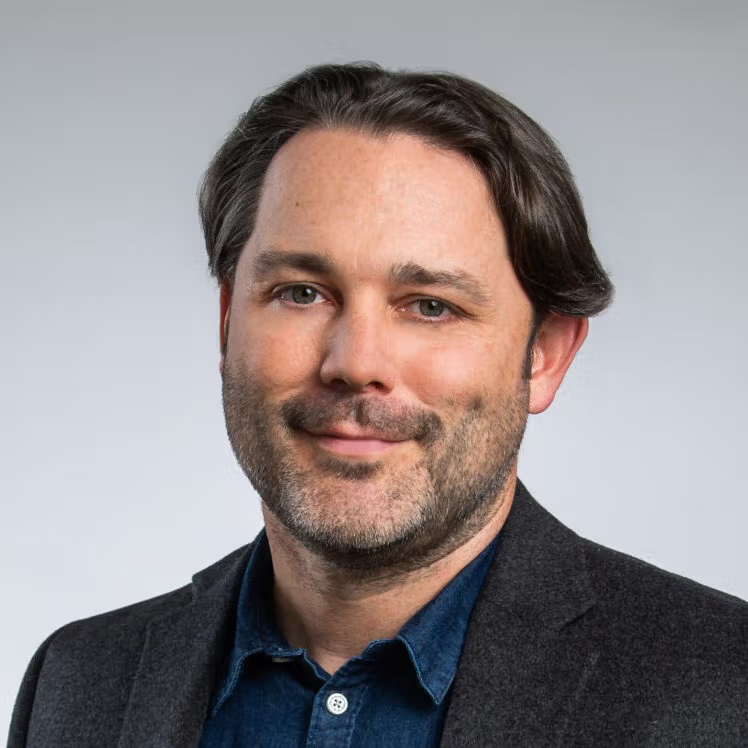