Data Scraping Makes AI Systems Possible, but at Whose Expense?
Hanlin Li / Jul 20, 2023Hanlin Li, Ph.D., a Postdoctoral Scholar from UC Berkeley’s Center for Long-Term Cybersecurity (CLTC) and an incoming assistant professor at UT Austin, issues a call for regulatory support for data stewardship.
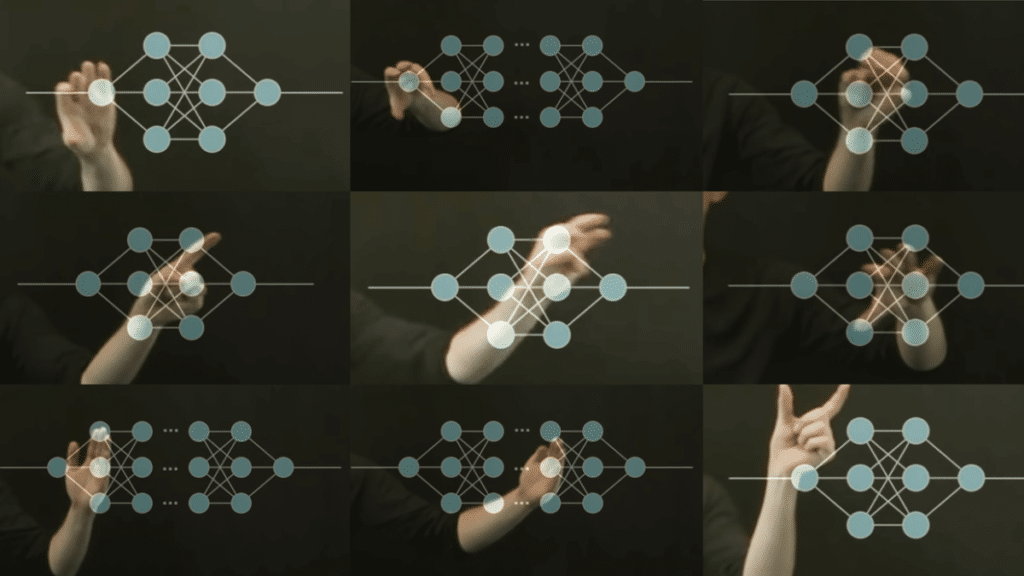
Prominent AI technologies, from ChatGPT to Midjourney, are possible thanks to data scraping — a data collection technique that involves downloading vast swathes of information on the web, from images to articles to code. It usually involves a computer program that scans web pages and stores the information in a structured format. This technique is not new, but it has become immensely popular among technology companies developing AI systems. Advanced AI systems, such as large language models, require a massive amount of training data to be successful. For example, the Common Crawl dataset, the data source for training GPT-4, is made of data scraped from billions of web pages.
The breadth of today’s data scraping practices means that every content producer is a data worker, whether they like it or not.
While data scraping has proven to serve companies well, its limitations and unintended consequences to society are becoming more evident than ever. Currently, anyone with the right tools and resources can scrape, store and use data with little to no oversight. Data scrapers can freely traverse the web and collect any public information for their own purposes, from surveilling the public with facial recognition technologies to generating images mimicking an artist’s work. For those of us who produce data as users and content creators, there is no way to prevent companies from scraping and using our data. The lack of consent, copyright protection, and privacy considerations are hugely controversial from users' and content creators’ perspectives. For example, recently, upon learning that their content has been scraped by AI companies, fan fiction writers revolted by deleting their writings and flooding their pages with irrelevant content.
At the heart of the tensions around data scraping lies a lack of data stewardship. Data producers are forced into a binary choice: stop sharing their content and data with the public altogether, or let companies run wild with this valuable resource. But it doesn’t have to be this way.
Researchers have been brainstorming and investigating feasible ways to equip data producers with more control (e.g. Glaze, a tool that prevents generative AI models from learning artists’ individual styles and attaching license clauses to datasets), helping to pave the way for public policies to promote and enforce responsible data use so that the outcome of data abides by data producers’ values and wishes.
While these technical solutions offer short-term solutions to data stewardship, structural changes are necessary to resolve the tension between data scraping and data producers. Researchers and regulators must prepare for the long run in establishing comprehensive, meaningful data stewardship mechanisms for all. A key long-term goal is to establish a meaningful feedback mechanism between data producers and data scrapers. Data scraping obscures the people who produce original data and provides opportunities for companies to reuse data without consent. This technique has been reflected in some high-profile cases. Recently, eight unnamed plaintiffs alleged Google illegally used their content, photos, and copyrighted work to develop AI products. Earlier this year, a trio of artists brought lawsuits against AI firms for using their artwork without consent. Last spring, Clearview AI, was forced to delete data about UK citizens by the country’s Information Commissioner’s Office, following controversies about the company collecting facial data without people’s consent.
These incidents from members of the public highlight the need for a formulated avenue to voice their grievances and influence how companies reuse data. Such a feedback system between data producers and data users may take the form of a shareholder meeting or town hall gathering. Researchers have proposed other various approaches to implement such feedback channels, including establishing third-party entities that broker data with data users such as data intermediaries, data cooperatives, and data stations. All these types of interventions can help companies currently with a strong reliance on data scraping to collect feedback from data producers about what are acceptable use cases for their data.
The mission for data stewardship is not just one of agreement, but also one of representation and rigor. When we think about whose data is included in training and whose perspectives are represented, we see that data scraping is likely to lead to biased datasets. For example, the BookCorpus, a widely used dataset in large language models at Google and OpenAI, contains a significant number of romance novels scraped from the internet. The stereotypical language used in such text datasets is one of the causes for large language models to perpetuate social biases concerning gender. Such representation harms do not seem to be going away any time soon, as seen in a recent case. Data stewardship will require continued research and regulatory support to help tease out and mitigate explicit and implicit biases in aggregated datasets.
As data scraping enables AI firms to harvest free labor and data, policymakers and researchers must double down on their efforts to establish data stewardship so that the future of AI is built upon consent, equity, and rigor. For all the praise AI systems receive, data producers who made these systems possible should have a say in the matter.
Authors
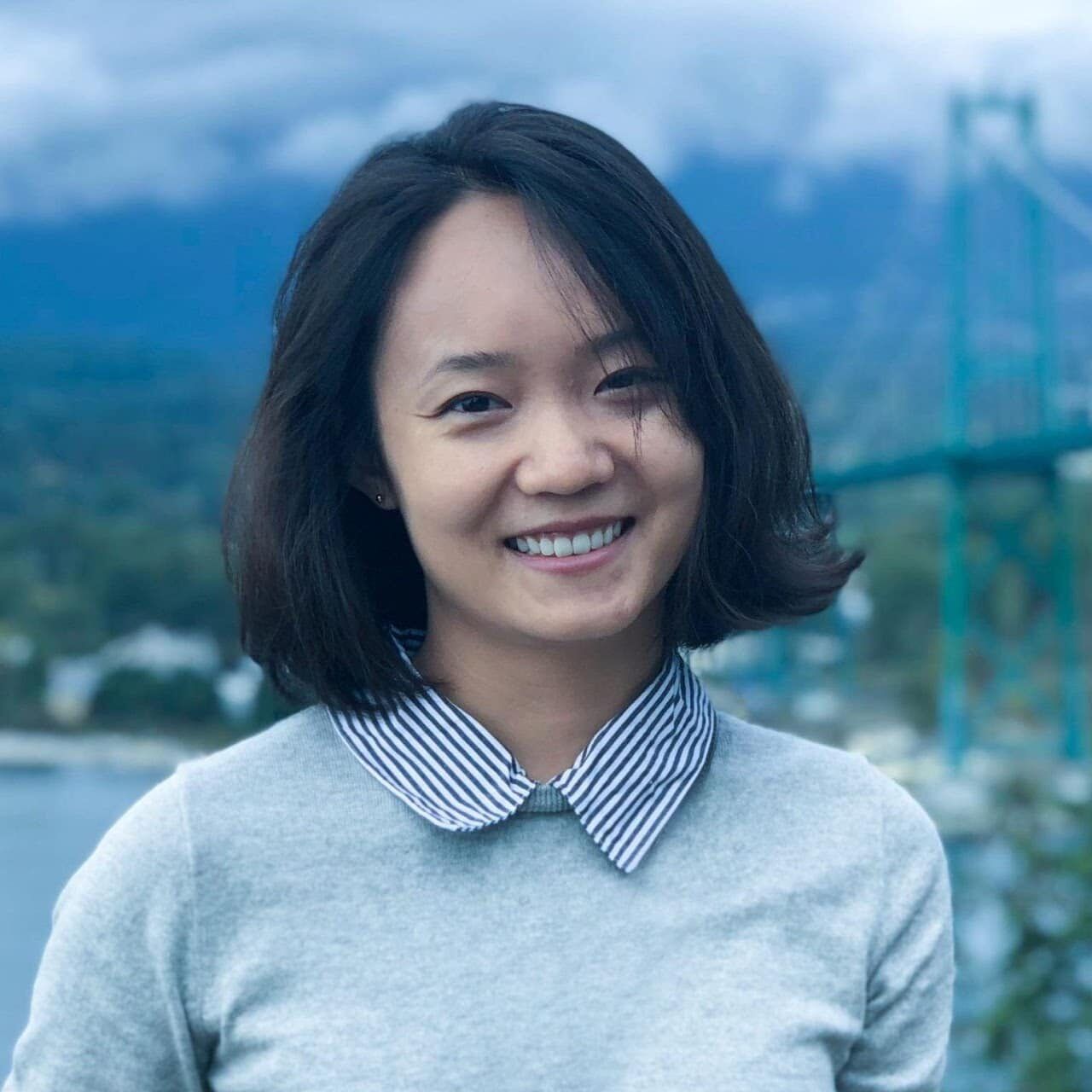