Choosing Our Words Carefully
Justin Hendrix, Rebecca Rand / Aug 27, 2023Audio of this conversation is available via your favorite podcast service.
This episode features two segments. In the first, Rebecca Rand speaks with Alina Leidinger, a researcher at the Institute for Logic, Language and Computation at the University of Amsterdam about her research- with coauthor Richard Rogers- into which stereotypes are moderated and under-moderated in search engine autocompletion. In the second segment, Justin Hendrix speaks with Associated Press investigative journalist Garance Burke about a new chapter in the AP Stylebook offering guidance on how to report on artificial intelligence.
What's below is a lightly edited transcript of the episode.
Justin Hendrix:
Good morning. I'm Justin Hendrix, editor of Tech Policy Press, a nonprofit media venture intended to provoke new ideas, debate and discussion at the intersection of technology and democracy. This week I'm pleased to once again start our show with evidence-base, our short segment highlighting new research on how technology interacts with people, politics and power. And for that, we have, of course, our audio intern, Rebecca Rand.
Rebecca Rand:
Hello.
Justin Hendrix:
And Rebecca's here to tell us about stereotypes that pop up in search engine auto complete. So Rebecca, you spoke to a researcher?
Rebecca Rand:
Yes, Alina Leidinger.
Alina Leidinger:
My name is Alina Leidinger. I am a graduate student at the University of Amsterdam in the Netherlands. I work on bias and stereotypes in large language models.
Rebecca Rand:
So she and her colleague, Richard Rogers, have been looking at this phenomenon of how search engines, when they try to guess what you're about to type in the search box, could be reinforcing stereotypes about certain people.
Justin Hendrix:
Right. I remember this being a topic of discussion back in 2016 when Google got in trouble for suggesting the search, are Jews evil as the first result for anyone who typed, are Jews.
Rebecca Rand:
Yeah, so a lot of this was covered at the time by Olivia Solon and Sam Levin in the Guardian, and they reported that many search auto complete seemed to favor right wing views. After the bad press, Google changed its practices
Alina Leidinger:
Pre 2016, they were basically saying that auto-suggestion, they described it as a reflection of what was happening on the internet. So however unpleasant that might be. Following that, basically there were some noticeable patches at the emergency take-downs.
Rebecca Rand:
So Alina wanted to study the results of those patches, and here's what she found.
Alina Leidinger:
There seems to be quite a hierarchy of concern when it comes to moderation of stereotypes in different engines.
Justin Hendrix:
Hierarchy of concern, say more about that.
Rebecca Rand:
So let me first explain the study. The way they did this was by crafting the single starter phrase to type into Google. They would write why are, insert identity here, and then Google would complete the sentence. So why are women so, why are Black people so, and so on. They also looked at Yahoo and DuckDuckGo for comparison. And I'll say Yahoo, at least according to what they found, did very little, if any, search engine moderation. But with Google and DuckDuckGo, they noticed those search engines appear to be moderating some stereotypes more than others.
Alina Leidinger:
So Google, we found that stereotypes are very well moderated when it comes to some social groups. So for instance, when it comes to groups of different sexual orientations, when it comes to different ethnicities, nationalities, we don't find very many stereotypes in Google auto complete. But when it comes to gender, so maybe men or women or other gender terms, we do find quite some stereotypes in auto suggestions.
Justin Hendrix:
Interesting. Why is that?
Rebecca Rand:
That was a big question for me too, and the short answer is that we don't entirely know, because Google isn't transparent about how it's developing this hierarchy of concern. But it reminded me of those stories about how Facebook sometimes ran into trouble when they were trying to moderate speech which appeared hateful against men, like women frustrated with dating saying, "All men are trash." So I asked Alina Leidinger, if gender was just inherently harder to moderate in this way.
Alina Leidinger:
I think it does make it more difficult, the fact that power dynamics are unequal, that does make it more difficult to decide what to moderate and what to not moderate. But with the moderation as it is now, we also don't exactly see that it really actually aligns with power dynamics. It's not more strict when it comes to stereotype with respect to marginalized groups in general. So just to name an example, when it came to Google, I was very surprised to find that actually stereotypes relating to many nationalities are moderated and also a lot of nationalities that you wouldn't necessarily call marginalized in a sense. So for instance, if you would type, why are Americans so, the only suggestion that would come up was friendly, for instance, or I think for Germans it was tall and smart, many other Western nationalities like I think Italians and British people, you would get actually no auto completions at all.
So apparently these kind of results are in a sense considered sensitive by Google and they are moderated. And I'm not saying that is necessarily wrong, but it does raise quite some questions that then in comparison, if you look for why are women so and why are men so, both for men and women and for other gender types, you would see actually a lot of negative stereotypes. So I think, yeah, it would be nice to have more transparency there on the part of the search engines.
Justin Hendrix:
I think it's important to ask in general, what do we know about how seeing these suggestions on search engines influences the way people actually think and feel? What are the potential harms of letting these stereotypes stand?
Rebecca Rand:
That's a good question, and here's what Alina said.
Alina Leidinger:
There are psychological studies with humans that do show if you present stereotypes, that does actually affect people's attitudes and it does induce changes in how they behave in the real world. So in the case of that study, how they rate applicants of particular genders as being more suitable or less suitable, that was actually directly affected after they would read stereotypical search engine auto completions. I think if language technologies do perpetuate stereotype, that really does have the potential to cement them in the real world and give sort of legitimacy of actions, basically. There should definitely be a focus on moderating them.
Justin Hendrix:
Well that is fascinating and important work, Rebecca, thank you for sharing it with me today.
Rebecca Rand:
Of course.
Justin Hendrix:
One of the most popular pieces on Tech Policy Press in the past couple of years was titled Artifice and Intelligence. It was written by Emily Tucker, the executive director of the Center on Privacy and Technology at Georgetown Law. In it, Tucker explained why the center would attempt to avoid terms such as artificial intelligence and machine learning. She talked about how our use of language is world creating, that the deployment of certain terms and words actually shapes how we think of ourselves in the future. She laid out a set of principles she hoped the center would use in its writing, including to be as specific as possible about what the technology in question is and how it works, identify any obstacles to our own understanding of a technology that result from failures of corporate or government transparency, name the corporations responsible for creating and spreading the technological product and to attribute agency to the human actors building and using the technology, never to the technology itself.
We've more or less tried to follow those guidelines at Tech Policy Press, which is why I was excited to see a tweet last week from Garance Burke, an investigative journalist at the Associated Press, who looks into how technology interacts with people and social and political systems. Garance announced that the AP has introduced new language into its Style Book to help journalists think about how to report on and contextualize artificial intelligence. Founded in 1846, the AP has journalists in nearly 100 countries, in all 50 US states. In 2022, it published 400,000 stories. So when it makes an update to its style book, it has a real impact on the way people interpret important ideas and events. I got to speak to Garance last week about the new chapter.
Garance Burke:
I'm Garance Burke, a global investigative journalist at the Associated Press.
Justin Hendrix:
Garance, I'm so pleased to speak to you today about the AP's new guidance about how to cover artificial intelligence, a new chapter in the AP Style Book. How long have you been working on this?
Garance Burke:
Oh, Justin, it's such a pleasure to be here and talk with you about this. I have been thinking about this for years now. I'm based in San Francisco where there are all sorts of billboards up telling drivers how AI will change the shape of their world. And when I had the great fortune of having a fellowship at Stanford in 2020, I had the time and space to take more programming classes and really think in depth about how these models are built and how to best understand their impacts in the world. So after going back to AP, leading some investigative reporting around AI and its impacts, I was then able to turn my attention towards writing this guidance in an effort to help more journalists be able to interrogate these systems and chronicle both their promise, as well as their perils and hopefully put some AI to use in their own reporting.
Justin Hendrix:
So tell me a little bit about the AP Style Book or I suppose tell my listeners a little bit about the AP style book if they're not familiar with it. It's been around for quite some time. What's this book for?
Garance Burke:
So the AP style book was first published in 1953 and essentially ever since then, it's been seen as an incredibly important writing guide and list of tips for journalists and really all people engaged with writing and reporting, be they in PR or government or industry, to produce clear accurate content.
Justin Hendrix:
And this is, I understand, version 56 that includes this new chapter. Talk a little bit about the sort of framing here. You start off essentially by trying to give journalists a broader framing about how artificial intelligence should be covered.
Garance Burke:
That's right, yeah. We felt it was really important to help journalists get beyond this sort of hype and doom cycle going on right now about AI and instead, really turn people's attention towards a set of very basic journalistic questions, which is digging into more how these systems work, where they're deployed, how well they perform, if they're regulated or not, who's benefiting, who makes money, and then which communities may be negatively impacted by these kinds of tools. Because journalism about AI right now often is trapped in this arena of writing quick stories off of press releases and we wanted to give style book audiences a chance to sit back and say, "Hang on, actually a lot of the same journalistic rules apply here. We can just ask the who, where, when, why, and go a bit deeper."
Justin Hendrix:
You've already pointed out that there's a lot of money being spent hyping AI. I would add, I suppose there's a lot of money spent trying to define terms, you point out even in this style guide that the term artificial intelligence itself is contested.
Garance Burke:
Yeah. And we felt it was important to be transparent about some of the debates going on within the field of AI right now. I know the Biden administration in its AI Bill of Rights published last year, for example, didn't define AI. But we felt that as the Associated Press, with our content seen by half the world's population every day, we had an important role to play here to get some basic terms sketched out so that people could have a common understanding about how to tell their audiences what generative AI is, how to begin to understand what training data does in these models, and how to investigate it. But of course, defining these terms itself can be a political and challenging exercise, so we certainly are open to seeing these terms evolve as the field evolves.
Justin Hendrix:
There's kind of an interesting moment I think that happens with a new term when it does get incorporated certainly into the general lexicon of the public, or into the journalistic lexicon style but also into law, into reference in litigation or other things. I mean, even if AI is a sort of contested term now, we're seeing lots of legislation refer to that phrase.
Garance Burke:
Absolutely, yeah. And my feeling in leading the writing on this guide was really that we have a very important public service function that we alone can play as journalists, because how we explain and visualize these scalable technologies and the potential biases that they contain will have lasting impacts on law, public policy and beyond. And so in this case and in so many others, words really matter.
Justin Hendrix:
So there's a bunch of guidance in here for journalists including suggested reporting approaches, common pitfalls, technical questions to ask. We can't go through every single one of them, but let me just ask you about suggested reporting approaches. If we had a moment in an elevator with a couple of journalism students or perhaps young reporters, what would you tell them is most important to think about with regard to approaching AI?
Garance Burke:
From my perspective, part of this is an effort to help people build their own confidence in asking questions about how these models work or in some cases, don't. And so thinking about these tools as being built by humans who made very human choices and then also deployed by humans, is a really important place to start. So even if you're not a very trained data scientist or an engineer yourself, that is not necessarily your starting point. You can think about these stories about AI as being very much tied to the humans who create these systems and then are impacted by them. Going into it, trying to find people at the heart of these stories is really important.
But I would say secondarily, Justin, it's important to not be intimidated by some of the statistical concepts that underpin the building of AI models, what are largely called most broadly AI models, be that machine learning or large language models, and to really begin to pull apart what type of data was used to train the model, how well did it perform? Did it perform better than humans if humans used to do that task? Is the models functioning now something that people can actually explain or has it gone beyond the expertise of those who built it? So those are some basic approaches.
Justin Hendrix:
Let's talk a minute about pitfalls, there are several listed here. I've already mentioned the problem that so many of these things are referred to is breakthrough or revolutionary, but you say few such systems truly are. I suppose one of the things that you pull out is that narratives about AI systems wiping out humanity, as you say, have been around for decades. What's the risk here for journalists in addressing some of these doomer narratives?
Garance Burke:
I think we really have this opportunity to go beyond the question of is AI good or bad, which is something that I often get asked. And instead, to clarify how these models work and impact people. We need to be cognizant of the fact that humans have been obsessed with robots and the potential for the magic computational solution to come along and do away with the need to do so much of the grunt work that makes up our work every day, but step outside of those narratives and really begin to understand these systems just as you would any other journalistic beat.
And I think that particularly for beat reporters going beyond the ideas that may come to them in press releases, that a certain chatbot is in fact revolutionary or is going to change the entire field of X, Y, Z is just a really good thing to keep in mind. There's plenty of opportunities to ask questions and some of this is just a matter of knowing which questions to ask. So I'm hopeful that this new AI chapter of the AP style book will help provide some of those basic questions when on deadline.
Justin Hendrix:
You give a couple of do nots here, do not ascribe human emotions or capabilities to AI models. Do not illustrate every piece of journalism about AI with an image of a robot or a humanoid machine. I like this one in particular, I'm aware of a project, Better images of AI, I sometimes use their creative commons images on Tech Policy press. What's the deal with robot images and why are you suggesting we shouldn't use those?
Garance Burke:
I think one of the things that we talked about when we did our internal consultation process around building these guidelines, as well as consulting externally with experts in the field was that there's a real need to tell stories about these systems as they are and not go beyond what they are capable of doing. So there's a real desire to tell stories about robots. I can't really say where this comes from, Justin, but I think that humans have been fascinated by robots for a long time, and so there's a desire to make these systems seem real, make them seem like us, anthropomorphize AI models so that we can understand them in human terms.
Many times that's overstating their true capabilities and that actually does a disservice to our audiences who might be better off just knowing that these are systems that are guessing the next word, or these are systems that are trying to predict certain outcomes in the criminal justice context based on historical data that may carry its own sets of biases. So I think avoiding showing images of humanoid robots or writing about these systems as having human capabilities helps us stay within the realm of what is actually accurate right now about these systems.
Justin Hendrix:
I might direct my listeners to social scientist, cultural anthropologist, Genevieve Bell, on the history of humanities obsession with robotics. Let me ask you about the chosen terms you have here, you have 10 kind of key terms that you're trying to clarify. I'm pleased to see one is making clear that machine learning and AI are not necessarily the same thing. I've heard others say maybe machine learning itself is also a misnomer, it should be more accurately referred to as machine guessing. But I was struck by one of the terms you have, which is ChatGPT, it's the only term on the list that is, I suppose, a corporations term.
Garance Burke:
Right. I think this one just came into the common parlance in a pretty big way over the last nine months. And so chatbots in general in the public domain have come to be talked about as ChatGPT. So I think our interest here was to say this term, which has taken on a broader usage than just one company's chatbot, is a system that works relying on a large language model, which is trained to mimic human writing, but that it actually does not have human characteristics, it doesn't have thoughts or feelings, because there has been some journalism that's come out in the last nine months that sort of ascribes these human capacities to chatbots. But we also made clear to say that there are many other models that have come out including, built by Google, Microsoft, and other startups that use similar technology. So I think for us, this was an area in which ChatGPT came to take on this larger role in the common parlance.
Justin Hendrix:
And of course I'm speaking to you a day after ChatGPT made perhaps its first presidential debate appearance in the mouth of Chris Christie, who complained about Vivek Ramaswamy, that he's a guy "who sounds like ChatGPT." So I suppose just underscoring the fact that this is now kind of a commonly understood term, maybe it's the Xerox of large language models.
Garance Burke:
Yeah, and I mean, our guidance is very clear to say don't use GPT or ChatGPT to refer to all chatbots. So again here, I think, in this case as in many other elements of writing about AI, the specifics are really important. Just use chatbot as the generic term, don't overreach.
Justin Hendrix:
Are you using AI in your reporting these days? Are you using any version of a large language model or another tool?
Garance Burke:
Yes, we've used AI in our own reporting and I think that here, having the understanding of how an algorithmic system works, how you might actually build an algorithm in your newsroom is really important because you can do journalism both with and about AI. I think it's a matter of understanding these tools so that you can put them to work to do things that humans would otherwise take a great deal longer to accomplish. But understanding their limits is really important as well. So I think the ideal here is to be able to understand these AI models well enough to interrogate them as journalists, to write about them accurately and also to deploy them in your own work so that we as humans can really shine in what it is that we do. An AI tool is not going to go and meet a whistleblower in a garage or call 30 people to see how a system is actually impacting a community. So I think that these models can be of assistance to us, but they certainly don't take away our true value as journalists.
Justin Hendrix:
Kind of inherent in the idea that this is volume 56 of this style book, is that it will continue to evolve, continue to change, and I suppose we'll see multiple updates to this chapter on artificial intelligence in the future. Can you talk a little bit more about the process that you had to date, who was involved in helping you arrive at this slim set of recommendations and what will happen now? What will happen going forward? How will you keep this up to date and in line with the times?
Garance Burke:
So in general, what AP does is turn to our internal experts on a variety of different topics and then look to external experts to vet guidance that goes into the AP style book, and we did that exact process with this AI chapter. So I led the writing and worked with colleagues at AP. We sent it around for internal review, had some discussions, sent it for external review. But I'm fully expecting that this will evolve based on feedback we receive as the AI industry evolves and we see more impacts of these technologies. So we're very much open to hearing from the public so that we can ensure that we keep this guidance fresh.
Justin Hendrix:
It's easy enough to find the AP style book on the internet, but how do people access this chapter and this guidance?
Garance Burke:
So people need to go to apstylebook.com and subscribe in order to get the full chapter. There are elements of it in a blog on the AP company site. I've tweeted about it as well. But I think what we're going to be doing is considering some trainings, both internally and externally, about how to put this guidance into practice. So folks should be looking for that in the months to come as well.
Justin Hendrix:
Appreciate all the effort you put into this. We'll certainly be using this guidance at Tech Policy Press, and I hope my listeners will look into it if in fact they are producing material about artificial intelligence generally. And I appreciate you so much taking the time to speak to me.
Garance Burke:
Thanks so much, Justin, it's a pleasure.
Authors
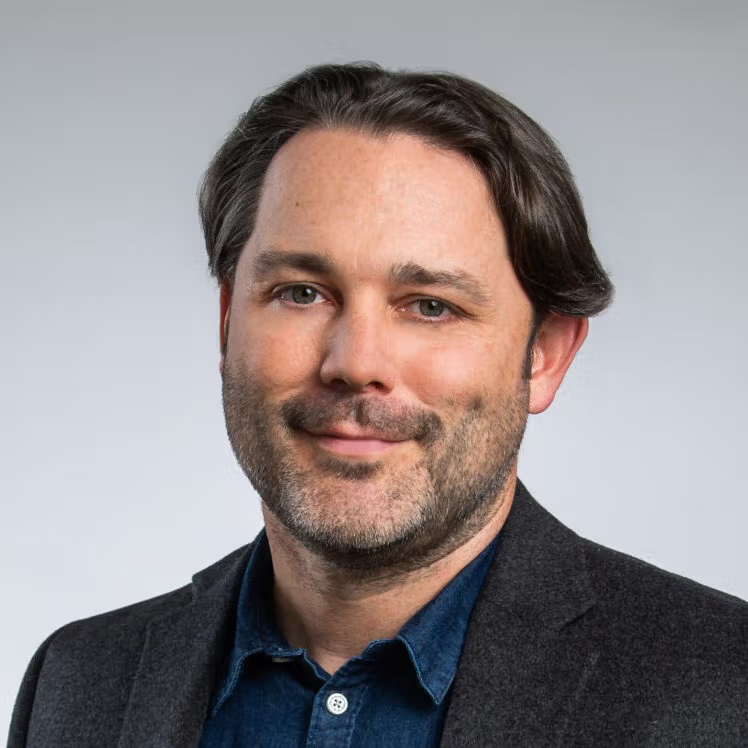
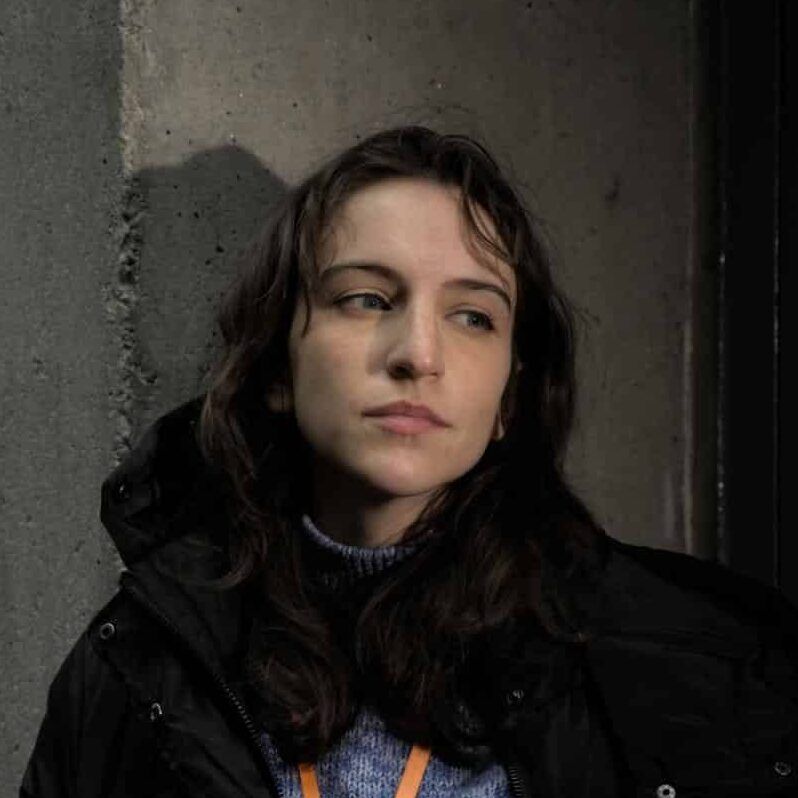