Challenging The Myths of Generative AI
Eryk Salvaggio / Aug 29, 2024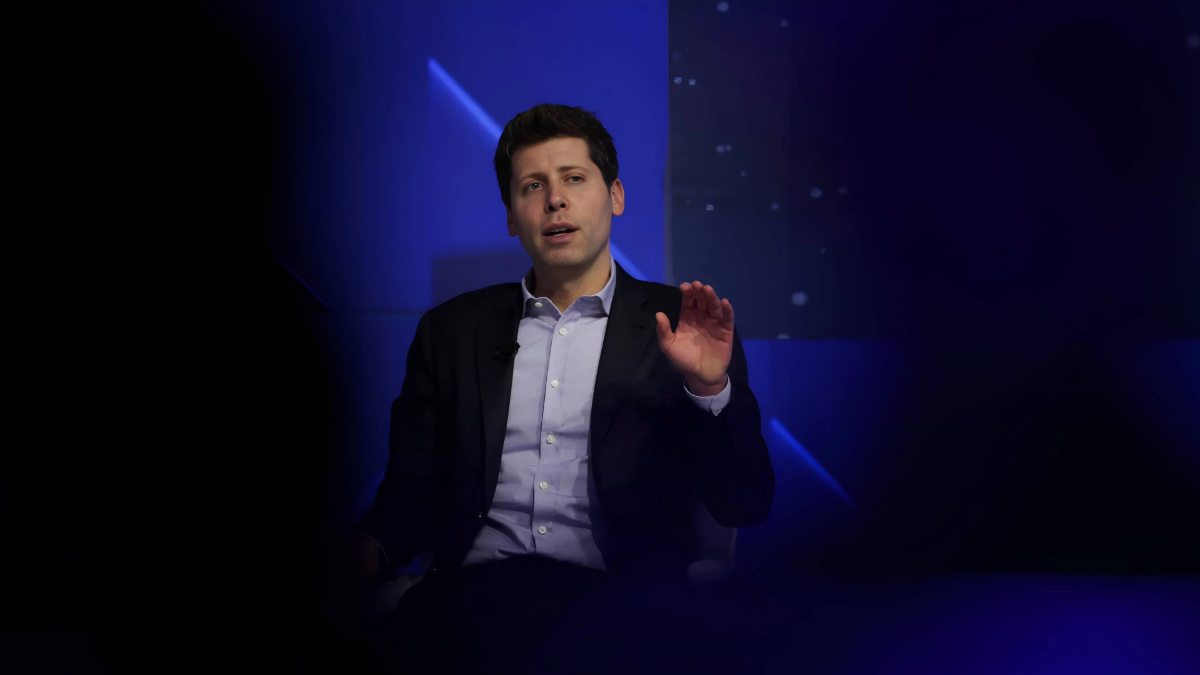
Sam Altman, CEO of OpenAI. Shutterstock
Aside from the physical heft of data centers seen from highways and the fiber optic cables crawling into homes and offices, the digital world mostly exists in our imagination. That imagination is shaped by the people selling services that rely on that infrastructure. This has given rise to a mythology of technology that aims for simple, graspable explanations at the expense of accuracy.
Companies marketing technology products and services wield enormous influence over our understanding of things. Marketing can lean into complexity to obscure the challenging aspects of these products or smooth out any rough edges through oversimplification, and the designers of marketing materials play a key role in this process. It isn’t always with ill intent. As a profession, marketing relies on myths to help us understand these technologies, and myths animate how designers imagine these systems.
There’s a competing set of interests at play: New technologies need simple metaphors to thrive, but simple metaphors aim to reduce complexity. Meanwhile, corporate boardrooms and founders believe in (or at least invest in) compelling myths and reward communications specialists for reinforcing these myths amongst consumers.
Given their origins, these myths inevitably skew to the techno side of techno-social equilibrium. They pollinate the social imagination with metaphors that lead to conclusions, and those conclusions shape a collective understanding. But if we want a socially oriented future for technology, we need myths that animate the social imagination of technology rather than overwrite it.
Why do these myths matter? Daniel Stone, Director of Diffusion.Au and a researcher at the Leverhulme Centre for Future Intelligence at the University of Cambridge, examines the frames we use when discussing AI and how it shapes our response.
“Myths and metaphors aren’t just rhetorical flourishes; they are about power. They tell us who has it, who should have it, how they should use it, and in the service of what goal,” he told me in an interview over email. “By actively choosing myths and metaphors that perpetuate a healthy, collaborative, ethical, and accountable understanding of AI, we can help ordinary people better understand how this technology works and how it should be used.”
Before creating beneficial myths to direct our use of technologies, we first should understand the current myths surrounding artificial intelligence. Myths don’t have to be cynically deployed, though they aren’t always innocent. Many seem obvious, but they continue to shape our thinking around AI. They infiltrate our thinking through convenience, as reliable shorthand, and as commonly understood references.
I’ve sorted a handful of these myths into rough categories. There are certainly others. The goal is to provide a way of looking at technology that scratches against the spectacle of metaphor to think more clearly about what AI is and does.
Control Myths
Generative AI is in a constant state of tension between randomness and constraint. Randomness is a tough sell – people pay for reliable systems, not chance machines. Control myths explain these systems in ways that emphasize the ways users can influence them rather than acknowledging inconsistencies, such as so-called “hallucinations.”
The reasons are apparent. Marketers use control myths to propose the benefits of this technology, while designers use metaphors to make alien technologies seem more intuitive. These metaphors can simplify AI but also seed an understanding that gives rise to a flawed understanding of how they work and when and how to use them.
The Productivity Myth
The productivity myth is a high-level myth that links AI systems to saving time. For example, an advertisement for Salesforce’s Einstein shows an employee saving time using AI to generate marketing copy for a new fashion collection. A similar campaign shows a father using Google Gemini to automate writing a letter on behalf of his daughter to an inspiring Olympic athlete.
Each case suggests — and misses the mark — the role of effort and who controls how the technology is used. We might imagine an ad where the camera lingers on an employee, realizing they are about to be replaced by a tool that generates inaccurate sales copy. Or perhaps we could see that daughter telling her therapist about a father who never allowed her to express herself because he felt Google Gemini was better suited.
The productivity myth suggests that anything we spend time on is up for automation — that any time we spend can and should be freed up for the sake of having even more time for other activities or pursuits — which can also be automated. The importance and value of thinking about our work and why we do it is waved away as a distraction. The goal of writing, this myth suggests, is filling a page rather than the process of thought that a completed page represents.
The productivity myth sells AI products and should be assessed on its merits. Automation is often presented as a natural driver of productivity — but as MIT’s Daron Acemoglu and Boston University’s Pascual Restrepo have shown, it’s not universal. Some automation is mostly good at producing economic inequality, limiting benefits to the concentration of wealth. Meanwhile, an Upwork study has shown that “96% of C-suite leaders expect AI to boost worker productivity [while] 77% of employees report AI has increased their workload.”
Researchers Dagmar Monett and Bogdan Grigorescu describe a related myth as “the optimization fallacy,” the “thinking that optimizing complex processes and societies through their simplification and fragmentation is the best option for understanding and dealing with them.” We see this in the disturbing example of a father taking away his daughter’s agency in writing a letter as a beneficial use in the guise of freeing up time — a logic of “it can be done, and so it ought to be done” that misses the mark on what most people want to do with their time.
The Prompt Myth
The prompt myth is a technical myth at the heart of the LLM boom. It was a simple but brilliant design stroke: rather than a window where people paste text and allow the LLM to extend it, ChatGPT framed it as a chat window. We’re used to chat boxes, a window that waits for our messages and gets a (previously human) response in return. In truth, users provide words that dictate what we get back. With shadow prompting, a phenomenon I wrote about in Tech Policy Press last year, our words are altered before reaching the model. The prompt window suggests more control over these systems than we have.
Artists working with diffusion models and people working with LLMs acknowledge the prompt myth (and dispel the productivity myth) when they describe the hours they spend honing a prompt to get a specific result. If the prompt myth were accurate, it wouldn’t need this effort: the prompt would tell the model what we want, and it would understand it and respond in a way that meets our needs.
The prompt myth helps hide the control that the system exerts over the user by suggesting the user is in control of the system. Consider the findings of a recent (albeit limited) study that suggested the images from a diffusion model captured the imagination of those who used it for brainstorming. These users then “fixated” on the generated example.
Most concerning is the illusion that LLMs are retrieving information rather than constructing word associations within a broad corpus. LLM responses are statistically likely rather than factually accurate. Sometimes these things correspond, but often they do not. We are currently seeing a mass mobilization of these technologies around the prompt myth. The premise is that statistically likely word pairings will produce a reliable reference to the information users seek. More competent companies are hedging their bets, emphasizing citations in their rephrasings. Any business model or use of these systems otherwise is a leap into the mythology of the prompt window.
Intelligence Myths
Intelligence myths arise from the reliance on metaphors of thinking in building automated systems. These metaphors – learning, understanding, and dreaming – are helpful shorthand. But intelligence myths rely on hazy connections to human psychology. They often conflate AI systems inspired by models of human thought for a capacity to think. This matters in policymaking, as it frames an argument that laws and rights afforded to humans should also be applied to machines carrying out automated processes. For example, it suggests that a model should be free to learn from the images or texts of others because humans do.
The Learning Myth
Learning is a helpful metaphor for what LLMs and diffusion models do. However, there is a distinction between the metaphor of learning and the process we describe with that metaphor. For one, a model doesn’t exist until it has “learned,” which is quite different from what we do as people: a student exists, and then goes to school, and then learns, using a mind that was already there. We value a student’s ability to learn because people have intrinsic value.
By contrast, a Large Language Model is a statistical model of the language contained within pre-selected training data. The model is a result of that training. As such, it does not “learn” but is created through data analysis. A similar shorthand describes evolution through natural selection: we might say that moths have “learned” to develop specific patterns on their wings because of their environment. We are more resilient to that idea because we know that moths have not been collectively informed about the state of the world to decide the colors of their wings.
Like the moth, an AI model is better described as becoming optimized to a set of conditions in which specific patterns are reinforced. Many a joker will suggest that this is also what school was, but it’s fundamentally different. Humans can exercise choice and agency over their learning and even decide to reject it. Learning from natural selection is distinct from education: nobody ever says moths or AI systems were educated. Yet, AI advocates want to apply educational freedom (the “right to learn”) to the companies that build Diffusion and Large Language Models.
It is not the case that “AI gathers data from the Web and learns from it.” The reality is that AI companies gather data and then optimize models to reproduce representations of that data for profit. It’s worth preserving distinctions between the process behind building software systems and the social investments that aim to cultivate the human mind.
Human learning is based on a pro-social myth: individually and collectively, human minds hold more social value than any corporation’s product development cycle. The learning myth aims to promote the equivalence between computer systems and human thought and establish these activities on equal footing within the eyes of the law, policy, and consumers. It supports a fantastic representation of the AI system as having human-like capabilities and social value, essential to selling it to people to do human-like things. But AI products do not learn from our data — they cannot exist without it.
The learning myth downplays the role of data in developing these systems, perpetuating a related myth that data is abundant, cheap, and labor-free. These myths drive down the value of data while hiding the work of those who shape, define, and label that data. The existence of this labor can sully the tech industry’s myth of representing progress and justice through technology. The AI industry is a data industry. The less we can see the costs associated with hoarding that data, the easier for companies to justify it.
The Creativity Myth
There is a rather stubborn conflation of the creative process with creative outcomes tied to generative AI. This is seen in diffusion models — tools that generate images from prompts — but also from LLMs. In the creativity myth, the artist's role is to produce images, and the author’s role is to create text. This conflates the labor of writing with its product.
This is not to say that those who use AI tools cannot be creative with them — I am an artist who uses AI to critique AI, and my creativity is quite different from the creativity modeled within a diffusion process. A diffusion model is not creative in that it cannot stray from the process that has been assigned to it and cannot fuse or play with meanings beyond accidental collisions. A human using an AI tool can be creative. However, the tool is not creative, nor does the use of a tool infuse a person with creativity by automating the outcome of a creative process.
The creative myth redefines human creativity according to a strict process, defined by a series of steps that run like a computer program. This framing of the creative process reduces human creativity to a single production method. It conflates the product of creativity with the process of creativity. That confusion has legal benefits for AI companies.
Like the learning myth, the creativity myth is prominently featured in policy and legal arguments about whether training data on scraped images should be protected. The goal of this argument is, quite literally, to suggest that the model should have the same rights as humans by diminishing distinctions between an AI product’s optimization algorithms and human decision-making. Along the way, this myth simplifies the diversity of human imagination into a single, routine process. That is neither the purpose nor value of creative expression.
Futurist Myths
Futurist myths point to the path of improvement in AI systems and assume a sustainable pace of innovation and problem-solving. Futurist myths also speculate about the impacts of systems where pressing problems have been solved to sidestep conversations about the challenges of AI systems being deployed today. Ironically, the future is sometimes employed to label discussion of the problems as counterproductive or Luddite while simultaneously asserting that the problems will inevitably be solved.
The Scaling Myth
One argument proposes that many problems with AI will be fixed with more data or better training. We also see that more data means more problems. For example, current regimes based on scraping create biased datasets that produce biased results. These will not be fixed by scraping larger, equally poorly vetted datasets from the same biased sources — unfortunately, hate scales.
Claims of widespread benefits from scaling AI are still based on theories. Of course, we have seen improvements in LLMs and Diffusion models through scaling. The question is, what is the nature of those improvements? In practice, scaling is primarily useful for broad, generalized “foundation” models — and only to a point. The idea that this is a path to a “general” intelligence — a system that can perform nearly any task at or beyond a human level — is still highly speculative. As for size, tremendous benefits can still be found in smaller models trained on more carefully curated datasets. Depending on the problems to be solved, smaller data can be better data. However, building smaller models on top of foundation models risks overwhelming smaller, carefully designed datasets with various unexpected biases.
The function of the scaling myth is multi-fold. For one, it frames data rights in oppositional terms. Companies that want to dominate the AI market claim they need more data. Policies that protect data interfere with their ability to scale these systems. The scaling myth resists such policies, often by pointing to geopolitical rivals – most often China. The scaling myth is also used to justify massive investments in data centers to investors. More scaling means more data centers, which draw on resources such as energy, land, and water. Notably, the larger the datasets become, the more difficult it will be to assess the content of those datasets and the ways they influence the output, complicating regulation and transparency efforts.
The Emergence Myth
Another animating myth of the AI moment is that of emergent behavior. Behavior can emerge in any complex system. Even a motor engine can display emergent behavior through inevitable wear and tear on its parts. The emergent property myth of Large Language Models — and even video-generation models — assumes the ability of the system to “learn” skills or derive new abilities through pure exposure to data.
Google Researchers Jason Wei and Yi Tay, in research that supports this view published in 2022, defined emergent properties as "abilities that are not present in smaller-scale models but are present in large-scale models; thus, they cannot be predicted by simply extrapolating the performance improvements on smaller-scale models."
But the case for emergence is far from settled. Dr. Anna Rogers of the University of Copenhagen has curated an excellent list outlining the challenges of assessing emergence and proposing several alternative explanations. Most rationally, emergent properties result from how these properties are measured. Likewise, many examples exist because the datasets were too large for researchers to know what they contained. With more training data locked behind proprietary walls, there is no way for skeptical researchers to evaluate such claims.
The emergence myth is a companion of the scaling myth. The scaling myth suggests more data will solve current problems and move on to accepting a set of premises. The emergence myth runs from benign to dystopian. For example, the emergence myth has suggested that LLMs may be closer to artificial general intelligence than they are — a great boon to investors.
This is also fuel for a host of doomsday fears about AI, encouraging limits to competition in the name of “AI safety.” Of course, countless problems can emerge from careless interactions between parts of complex systems. But we must be careful about articulating where this emergence happens: is it collecting large sums of writing that somehow leads to a superintelligence? Or is it more likely to emerge in the rush to integrate various AI systems into decision-making tasks they are wholly unsuited to tackle? What is more likely: a compression algorithm that suddenly comes to understand the world or one that we mistake for understanding the world — and then come to depend on? The practical risks of AI are not that they become super capable thinking machines. It is building complex systems around machines we falsely assume are capable of greater discernment and logic than they possess.
More Rigorous Myths
“When we are trying to understand large, complex, and highly abstract topics, such as the economy, migration, climate change, or AI, we depend on metaphoric thinking. Metaphoric thinking helps us convert these highly abstract concepts into something more tangible that we can understand,” Stone says. “Metaphors make up to 20% of typical speech and 40% of political speech. But only 3% of people even notice they’re being used. This means their power is significant but often unrecognized.”
The onus is on journalists, researchers, policymakers, and artists to stand up to these myths and demand more robust evidence whenever they arise. It can feel risky to challenge the myths: one risks appearing as if they misunderstand the technology. Challenging these myths, however, shows a healthy and skeptical orientation toward some very bold claims. In a time where adopting AI is taken for granted as progress, it can be hard to challenge the assumptions about how this progress is being defined.
We’re not entirely adrift. Organizations such as the Washington Center for Equitable Growth focus on diversifying points of view in research — and connecting those researchers to policymakers. Meanwhile, Aspen Digital has compiled a rich set of emerging tech primers for journalists covering artificial intelligence, empowering them to push back on the vocabularies of big tech. And researchers such as Daniel Stone are challenging the frames we’ve come to rely on in these conversations.
Finally, such myths can adversely impact well-meaning work. For example, there has been an encroaching ennui with the flood of ethics and values statements associated with AI. This is a bit of deja vu: it’s been nearly five years since Anna Jobin, Marcello Ienca, and Effy Vayena published an assessment of 84 AI Ethics Guidelines across industry and policy spaces. Since then, the generative artificial intelligence boom has presented a widespread re-evaluation and thinking-through of what principles should be applied to AI deployment.
Compared to that 2020 paper, there hasn’t been much of a change in the vocabulary of values. Those authors summarized a common set of values, which included transparency; justice, fairness & equity; Non-maleficence (i.e., do no harm); responsibility and accountability (“rarely defined,” the authors note); privacy; beneficence (i.e., do good); freedom and autonomy; trust; sustainability; and solidarity.
There is still value in values. Given the vast number of communities affected by AI systems, conversations about each of those systems, for each of those communities, are warranted.
Values help articulate fears and desires for artificial intelligence. However, if critiques and values statements implicitly buy into the premise of these generative AI myths, they are counterproductive. Relying on these myths undermines the importance of grounded, realistic conversations about the impacts of artificial intelligence on vital institutions related to education, criminal justice, and a healthy commons. We must work together to create a more rigorous understanding of what these technologies do (and don’t do) rather than developing value statements (and laws) that buy into corporate fiction.
Authors
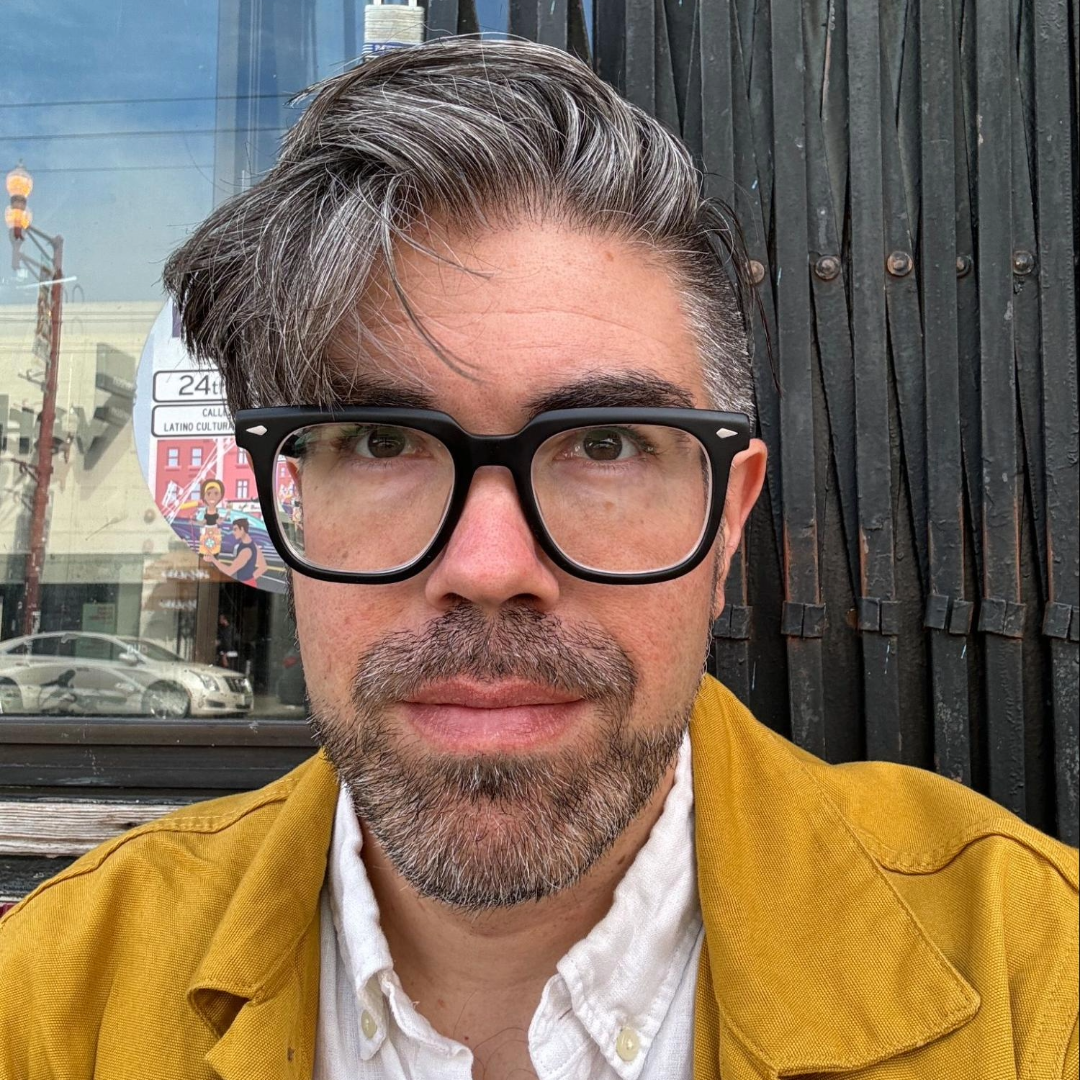