Assessing the risks of language model “deepfakes” to democracy
Cooper Raterink / May 21, 2021While some experts feared deepfake videos might play a role in disinformation campaigns targeting the 2020 election, the cycle ultimately saw very little impact of synthetic media. Perhaps the only notable incident was the use of a fake persona accompanied by a profile image generated with an generative adversarial network (GAN) in a disinformation campaign targeting Hunter Biden, President Joe Biden’s son. And while researchers are concerned about the future threat of systems like OpenAI’s GPT-3 language model, which debuted in June 2020, to automatically generate misleading text and voice content, this cycle instead saw verified political accounts and largely authentic grassroots behavior (such as well-intentioned and friend-of-a-friend misinformation) were most responsible for the spread of misleading narratives.
While synthetic text did not have an impact during this election, understanding the malicious potential of large language models such as GPT-3 or T5 is an active area of research. A major threat to election security posed by generative language models is the automation of astroturfing, which aims to camouflage a disinformation campaign as an authentic grassroots movement. A sufficiently powerful language model could generate misleading messages for a massive amount of bots, each spreading the same narrative and posing as a different individual expressing ‘personal’ beliefs. Alternatively, foreign content farms posing as legitimate American news outfits could use language models to accelerate content generation. Political actors might leverage the (inauthentic) support to manipulate public opinion.
Indeed, Renee DiResta, research manager at the Stanford Internet Observatory and one of the members of the Election Integrity Partnership, a group of researchers who monitored the largely human generation of disinformation in the 2020 cycle, worries synthetic text “could instead be used in bulk, to stitch a blanket of pervasive lies.” And a new report from the Center for Security and Emerging Technology on the threat of large language models concludes that “humans now have able help in mixing truth and lies in the service of disinformation.” But, initial indications suggest there are important limitations to the deployment of these models by disinformants. Understanding the potential of these technologies, as well as the challenges to putting them to use, is important to gauge the threat landscape.
Obstacles to deploying language models for disinformation
Disinformants likely need automated text-generation methods to be scalable to hundreds or thousands of messages per minute, imperceptible to human users and platform detection systems, and targeted to specific narratives. Text deepfakes were not a consideration during the 2020 US Presidential election likely because language models cannot yet meet disinformants’ needs.Several factors around access, detectability, and generation quality continue to limit how useful contemporary language models are to bad actors:
- There are significant barriers to accessing state-of-the-art models or creating them from scratch. In their decision to release GPT-2 in stages, OpenAI stated “analyses indicate minimal immediate risk of a fully-integrated malicious application” due to technical challenges. They describe state-backed operations and other organized, well-funded efforts as the most significant threat. In an effort to minimize risks, OpenAI made the more powerful models available only after observing how the smaller models were used. The University of Washington researchers behind GROVER, a language model trained specifically to generate fake news, explain that they released GROVER to help researchers model and address the threat of “Neural Fake News.” Although GPT-2 and GROVER were indeed made public, researchers and security consultants took proactive measures and encouraged adversarial research, making it difficult for disinformants to use these models to their advantage. As an alternative to using pre-trained models, the disinformant could train one from scratch, but the large costs and advanced technical experience required to do so are prohibitive.
- On average, generated text is detectable, and the content remains unreliable. Open AI’s GPT-3, the successor of GPT-2, showed that its outputs are difficult for humans to detect. Research has indicated, though, that when sampled in the way that most easily fools humans, generated text is less likely to fool automated detectors such as GLTR (and vice-versa). Often the most notorious examples of AI-generated text, which fuel public concerns about the matter, are not first attempts, and their quality depends heavily on sampling parameters. Additionally, neural language models are ungrounded, generating content based only on statistical trends discovered in the training data. Bender and Koller critique this ungroundedness, arguing that vastly different techniques are required to achieve truly human-analogous natural language understanding. In sum, disinformants would need to tweak model outputs carefully to guarantee their messages go undetected and are cogent and on-topic.
- Platforms invest heavily in harm prevention. Tech giants have acquired companies and developed cutting-edge machine learning methods to combat bots, spam, and disinformation on their platforms; monthly, Facebook removes hundreds of inauthentic accounts from its platforms. Techniques such as propagation-based detection rely more on platform dynamics than on message content, and so their performance would be unaffected by enhancements in machine-generated text. A blog post written by the CEO of HuggingFace, a popular language modeling library, states that “[bot] detection usually relies on non-conversational limitations like captchas or requests and account creation capping.” AI-powered astroturfing requires avoiding roadblocks that cannot be dodged by improving text quality alone.
- Findings touting language models’ potential to generate disinformation are rarely contextualized. While there exists research demonstrating the misuse potential of contemporary language models, it does not fully probe that potential in the context of the internet platform. For example, researchers at the Center on Terrorism, Extremism, and Counterterrorism (CTEC) investigated the ability for GPT-2 to generate extremist texts. This work is essential, but there is also a need for work evaluating how the synthetic extremist content performs in action on social media platforms and in conjunction with evolving hateful narratives. Findings about machine-generated text in isolation need to be followed up with case studies and interdisciplinary research exploring how the same text would perform in context on platforms.
Even if researchers develop remarkable fake news generators using advanced language models, and disinformants gain access to them, it might not be worth it to deploy them in practice. Bad actors would need to make expensive and risky investments to build systems to maintain fake accounts amplifying synthetically generated messages. Given, as the Election Integrity Partnership reported, the accounts of verified political influencers and media figures contributed the vast majority of misleading content around the 2020 election, malicious actors’ money might be better spent on talking heads than on talking bots.
What the Future May Bring
Consulting the literature for an answer as to when or how a tipping point in synthetic quality will be reached is chasing after the wrong idea. The future of text deepfakes is not a story of ticking time bomb, but of cat and mouse. Diresta put it best in Wired: “The war between fakers and authenticators will continue in perpetuity.” The analogy of the cat-and-mouse game invites deeper consideration of how the players and the playing field are changing:
- Tech platforms and regulators are raising barriers for the use of deepfakes. Alongside investments in innovative detection systems, tech companies have made their policies about synthetic media more transparent and aggressive. Also, the United States Congress is becoming increasingly interested in regulating deepfakes and AI more broadly. As the Center for Security and Emerging Technology noted in its report on language models and disinformation, “The best mitigation for automated content generation in disinformation thus is not to focus on the content itself, but on the infrastructure that distributes that content.”
- For disinformants, access to larger language models and the resources to train and deploy them is expanding, but can be checked. The financial costs involved in training a language model to a given level of performance are consistently dropping, and models that are large by contemporary standards will soon become accessible to the general public. However, if it is true that models useful for astroturfing require application-specific research, such as GROVER, proactive ethical agreements between researchers in the space may be successful in making access difficult for malicious actors for some period of time.
- The problem of disinformation is inherently socio-political, and fostering deepfake media literacy is a promising prevention mechanism. Britt Paris and Joan Donovan explain that “any solution must take into account both the history of evidence and the ‘social processes that produce truth’ so that the power of expertise does not lie only in the hands of a few.” Power dynamics shape what a society believes to be true -- consider political influencers disseminating misleading narratives during the election. This observation points to the importance of social solutions to the problem, such as media literacy. Given time and educational materials, people can learn simple heuristics that will protect them against manipulation by synthetic text. For example, in the HuggingFace blog post mentioned before, the CEO points out that language model chatbots can often be exposed through simple means, such as by asking math or reasoning questions like “What is the day after Monday?”
- A motivating example: non-English social media communities. In domains where content is less likely to be moderated by tech platforms, such as in non-English speaking online communities, automatically generated content may go undetected. There may also be reduced awareness about synthetic text in these communities, meaning text deepfakes could spread more quickly therein. At the same time, improvements in language modeling and machine translation may improve platforms’ abilities to conquer such content, and growing synthetic media literacy will help these communities conquer it themselves.
With proactive countermeasures, even substantial innovations in language modeling may not change the nature of the game. Over time, the mice will multiply and become more elusive; to continue the chase, the cats must adapt. Technological innovations, such as synthetic text detection systems like GLTR or open-sourced fake news bots like GROVER, will accelerate this adaptation.
But perhaps even more important is spreading proper awareness about the issue at hand. For now, like language models themselves, popular concern about the current nature of the threat of automated disinformation is largely ungrounded. And there may be bigger issues to worry about with regard to their development—such as whether “racist, sexist, and abusive ideas are embedded” in the models, as MIT Technology Review’s Karen Hao points out in a report on the efforts underway to address such flaws. Fostering a measured public understanding of text deepfakes is a necessary step toward creating a society of minds resilient to them. Even if the 2020 US Presidential election was not overrun with deepfakes, it highlighted the profound danger of the spread of disinformation and lies in a democracy. The time to prepare for the next cycle is now.
Authors
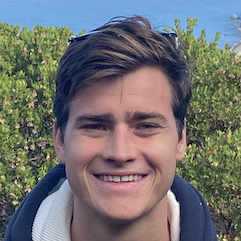