Artificial Intelligence Could Democratize Government
Luke Hogg / Mar 8, 2023Luke Hogg is the director of outreach at Lincoln Network, where his work focuses on the intersection of emerging technologies and public policy.
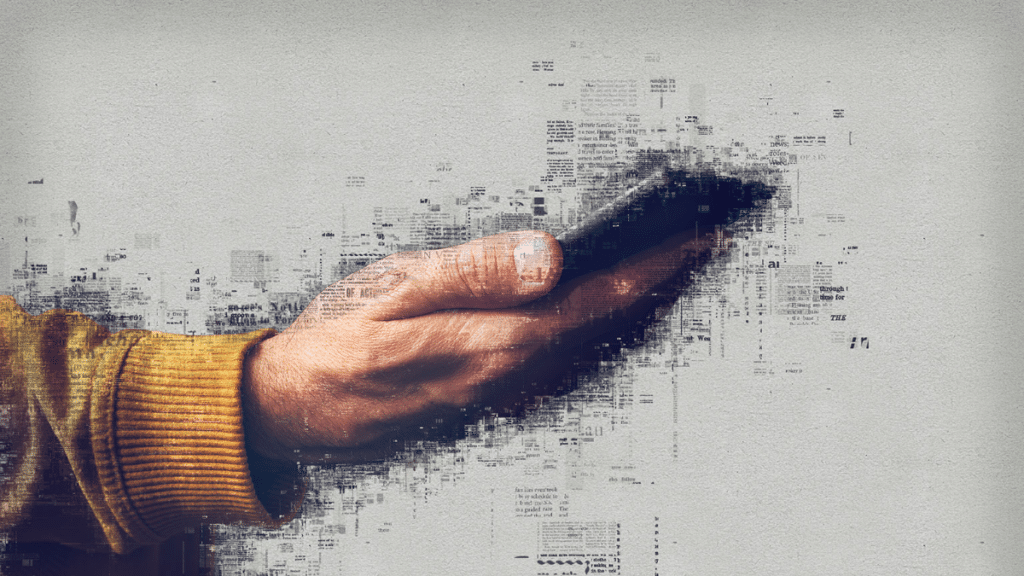
From education to media to medicine, the rapid development of artificial intelligence tools has already begun to upend long-established conventions. Our democratic institutions will be no exception. It’s therefore crucial that we think about how to build AI systems in a way that democratically distributes the benefits.
These tools could have a democratizing influence, making it easier for the average American to engage with policymakers—as long as they are built openly and not locked away inside walled gardens.
Late last year, ChatGPT—a chatbot built on top of OpenAI’s GPT-3 suite of large language AI models (LLMs)—took the online world by storm. As with other recent GPT-3 driven technologies, such as DALL-E and Whisper, many users have approached these tools in a lighthearted, playful way, enjoying silly conversations with ChatGPT and making DALL-E produce bizarre images. But what happens when the technology is applied, not to relatively frivolous uses, but by corporate America and the federal government?
Advances in AI are already causing anxiety in the business world, even among tech giants. Reports indicate that Google is at DEFCON 1, having brought in the original founders, Larry Page and Sergey Brin, to help top executives strategize a response to GPT-3. Going one step further, Paul Buchheit, an early Google employee and the mind behind Gmail and AdSense, recently claimed that advancements in AI could put Google “only a year or two away from total disruption.”
Such disruption won’t end with the corporate world. Many social scientists have begun to warn that AI will undermine democracy itself.
Researchers are already examining the ability of LLMs to perform the job of a corporate lobbyist. One study from John Nay of the Stanford Center for Legal Informatics found that the most recent version of GPT-3 successfully determined whether a bill is relevant to a specific company 75 percent of the time. While Nay concedes that we are still a long way from totally automated lobby shops, the idea that LLMs could begin to be deployed to influence lawmaking on any scale is, to some, troubling enough.
One concern is that AI systems will become tools of the elite to more efficiently wield influence over policymakers, making the U.S. less a democracy than a plutocracy. A recent piece by Nathan Sanders and Bruce Schneier in the New York Times, for example, opined that advances in AI risk “replacing humans in the democratic processes — not through voting, but through lobbying.” The authors conclude that “a future filled with A.I. lobbyists … will probably make the already influential and powerful even more so.” By improving the efficiency of traditional lobbying apparatuses and streamlining activism, the argument goes, AI could allow special interests to subvert the will of the people.
In a scenario imagined by Tyler Cowen of George Mason University, special interest groups and corporations could use AI systems to spoof public opinion and astroturf lobbying campaigns. As Cowen puts it, “interest groups will employ ChatGPT, and they will flood the political system with artificial but intelligent content,” making it more difficult to divine the public’s true opinion.
Such concerns are not unfounded. New technologies always present new challenges, and lawmakers should be cognizant of the risks AI might present and the methods for mitigating them.
Fortunately, innovators are already working on tools to counteract some of the potentially negative effects of AI. Even as schools and universities across the country worry about how to handle AI-enabled cheating, OpenAI has launched a new tool to detect AI-written text. As quickly as one startup can invent more realistic deep fake videos, another startup is hard at work upgrading deep fake detection software. These tools are notoriously difficult to build, but some of the best computer scientists in the world are working to solve these problems. In this technological arms race, content generators will always have the first-mover advantage, but those endeavoring to unmask fake content will not be far behind.
While these tools are imperfect—especially in the context of text detection—some have shown more promise than others. Generally, detection tools produced by adversarial third-parties such as researchers or other startups are better than those produced by the companies themselves. AI developers should take steps to ensure that their outputs are detectable to prevent misuse. For example, opening up AI tools would be beneficial as researchers and other developers are likely to be better able to build effective detection tools if the generative models are not locked behind walled gardens. By intentionally building tools that are open and detectable, AI developers can help prevent misuse.
The current discourse around LLMs and other AI systems is not the first time we have seen excessive hand-wringing over generated content’s impact on the democratic process. When the Federal Communications Commission (FCC) was in the process of repealing net neutrality rules in 2017, it received a record-breaking 22 million public comments on the proposed Restoring Internet Freedom Order. As it turned out, over 17 million of those comments were fake, nearly 8 million of which were generated by a single college student.
This and similar circumstances caused an uproar. Many pundits claimed that robocomments would be the death knell of democratic notice-and-comment rulemaking. The House Financial Services Committee went so far as to hold a hearing analyzing “how bad actors use astroturfing to manipulate regulators,” and the Senate Homeland Security and Government Affairs Committee investigated the issue. But, in the aftermath of all this, a report prepared for the Administrative Conference of the United States told a very different story.
While the report’s authors did recognize the challenges posed by fake comments and encouraged agencies to implement safeguards to prevent fake comments, they concluded that artificially generated comments do not “violate federal law and do not generally undermine the integrity of notice-and-comment rulemaking.” Furthermore, the report found no evidence of widespread substantive harm in either specific rulemakings or the rulemaking system overall.
Why not? As with legislating, notice-and-comment rulemaking is not a simple up-or-down vote based on the number of comments for or against. Instead, regulators examine the arguments in public comments and proceed according to a substantive, not quantitative, analysis of the record. Similarly, when deciding how to vote on a piece of legislation, lawmakers tend to consider their constituents’ substantive arguments, rather than simply counting the number of emails and phone calls received from each side.
Still, one can always argue that this time is different, and skeptics are right to insist that we think seriously about how we build technologies that help prevent the abuse of AI. To do so, we must ensure that these tools are built in ways that bolster the institutions of good governance. If they are built openly and honestly, AI systems could be a democratizing influence.
Historically, it has been difficult for an average citizen to meaningfully engage with their legislature. But, over time, technology has narrowed the gap between the people and their representatives in Washington. The telegraph, telephone, and Internet all came with growing pains, but all eventually made it easier for people across the country to participate more meaningfully in the policymaking process. AI is no different.
There are many ways that AI systems—and LLMs in particular—could make it easier for citizens to engage with their representatives in Congress. For example, Nay’s study cuts both ways: just as GPT-3 could determine the relevance of legislation to a company, it could also determine relevance for individuals. People who lack time to read through massive bills could have an AI tool do the time-intensive work for them and then contact their representative about the issues flagged by the AI.
AI’s democratizing influence isn’t a given, though; it depends on how the AI models are built. If AI systems are opaquely controlled by elites—be they governments or powerful corporations—then the risk of democratic backsliding will indeed become more substantial. To realize the promise of AI, we will need to build AI models and applications openly and make them widely available to the public. In other words, they should be open source wherever possible.
Within the context of AI, open source means that the code and data used to train and operate the AI system are available for anyone to view, use, and modify. Open-sourcing databases and software allows for greater transparency and encourages informed public discourse about the advantages and disadvantages of a particular model or application. It also increases the accessibility of software by ensuring everyone has equal access to the technology and allowing individuals to iterate upon existing systems. It should also make it easier for the forensics community to build detection tools.
Approached this way, open-sourcing AI tools could bring enormous benefits. Closed (particularly government-built) software is typically clunky and difficult to administer. Nimble, open-source AI systems, in contrast, could be rapidly customized and deployed to help detect and counter fabricated influence campaigns in ways that are difficult for top-down systems.
The democratizing value of open-source AI systems is even more significant for the public. As the public grows distrustful of centralized control by large tech companies, distributed open-source systems offer an alternative. Some large companies are already working to capitalize on the success of ChatGPT and other GPT-3 applications. Putting these potentially revolutionary technologies behind a walled garden would only make it more difficult for the average American to have their voice heard.
Open-source AI software does present some unique risks and challenges. Like all software, open-source software is vulnerable to cybersecurity attacks. Using open-source software typically requires a certain level of technical literacy. What’s more, the compute cost of training AI models and requirement for increasingly specialized human reinforcement needs could restrict AI’s democratizing influence. Builders who are working on developing AI systems designed to interface with Congress or government agencies should be aware of these risks and work to mitigate them wherever possible.
Apprehension about AI's political effects is reasonable, but every risk also presents opportunities if hedged properly. If built freely and openly, AI has the power to make Congress more accessible, efficient, and responsive. By making these systems open source and easily available to the public, we can better ensure that they are implemented responsibly within government and are a democratizing influence outside of government. If we take the steps now to encourage openness, then AI lobbying could ultimately lead to a more inclusive and representative democracy.
Authors
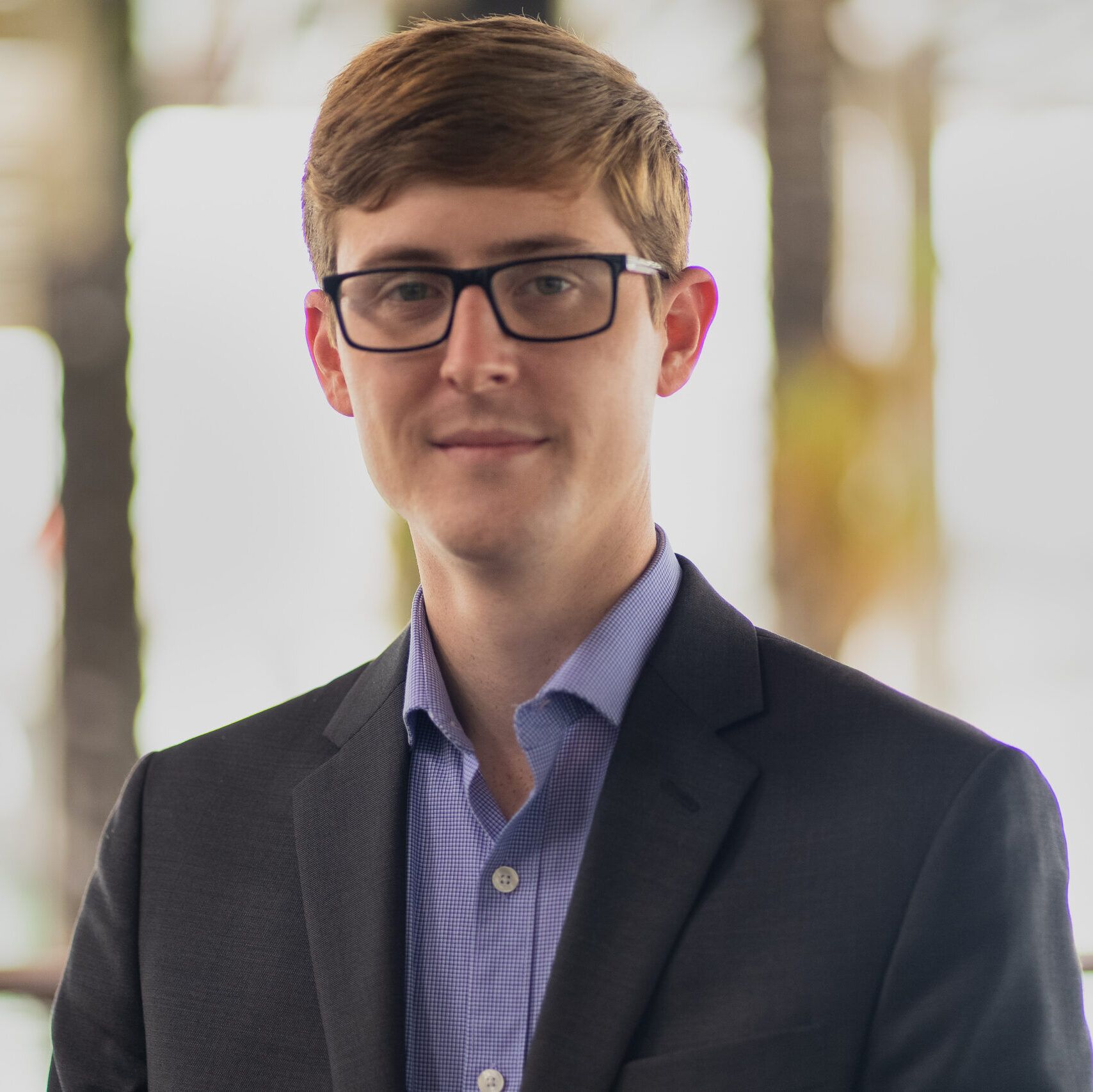