An FDA for AI?
Justin Hendrix / Dec 24, 2023Audio of this conversation is available via your favorite podcast service.
If you’ve listened to some of the dialogue in hearings on Capitol Hill about how to regulate AI, you’ve heard various folks suggest the need for a regulatory agency to govern, in particular, general purpose AI systems that can be deployed across a wide range of applications. One existing agency is often mentioned as a potential model: the Food and Drug Administration (FDA). But how would applying the FDA work in practice? Where does the model break down when it comes to AI and related technologies, which are different in many ways from the types of things the FDA looks at day to day? To answer these questions, I spoke to Merlin Stein and Connor Dunlop, the authors of a new report published by the Ada Lovelace Institute titled Safe before sale: Learnings from the FDA’s model of life sciences oversight for foundation models.
What follows is a lightly edited transcript of the discussion.
Merlin Stein:
I am Merlin Stein. I'm a PhD candidate at the University of Oxford.
Connor Dunlop:
I'm Connor Dunlop. I'm the European policy lead at the Ada Lovelace Institute.
Justin Hendrix:
And I'm quite pleased to talk to you about this report that you've produced on whether FDA style approval and oversight could be used for AI governance. I want to talk about some of the basics of this, what you've looked at here. You're basically talking about foundation models, so we're talking about how to govern the use of these ... Well actually I want to ask you about this before we even start. It's controversy around even calling these things foundation models.
Merlin Stein:
So I think background on foundation models, why we chose foundation models, there are probably three terms to use, advanced AI foundation models or frontier AI. Frontier AI is the term that we don't think has any kind of specific grasp because it's moving all the time so that we can't really define what it means to be at different tier and similar. Advanced AI has the downside that we talk about all different forms of AI, no matter whether they're general purpose or not. And it could be an advanced AI that is like DeepMind style protein-folding, but why we use foundation works because it's so important that these are the foundation, I know that it's something of a framing and I know that it's driven and there's a lot of debate about that, but we chose that consciously.
Justin Hendrix:
Okay, so just acknowledging that there is a controversy even still about maybe the semantic choices we're making about how to describe these things, but what we're talking about here is systems that are capable of a range of general tasks, everything from texts to images and video and maybe even accomplishing more complicated things going forward. Why do you admire the FDA?
Merlin Stein:
The FDA is one model to look at when we think about AI governance. What the interesting thing about the FDA is that the FDA has been established since 1906 and has a long history where it looked into the oversight of novel and high-risk technologies, which is some inspiration for advanced AI.
Justin Hendrix:
You point out that, I found this interesting that FDA regulated products account for about 20 cents of every dollar spent by US consumers. I'm not sure if that's plotted for the FDA or an indictment of the cost of pharmaceuticals in the United States, but I'm interested in how you see the risk analysis for foundation models as similar to medicine. How does that analogy come together for you?
Merlin Stein:
We looked into it from four lenses. So I think first of all, it's important to see that foundational models and AI, is in many ways very different than medicine or drugs. But if we look a bit more broadly, we think about the novelty and that's this thing where technology is developing fast both on the medical side as well as foundational models. Secondly, we think about the risk and there is the, we specifically speak about Class III kind of risks of medical devices that the FDA regulates, that is similar to some part of the foundation models. Could be current foundation models, could be future foundation models, depends, because that's the third part. We don't know much about the risks, so we don't have clear certainty what the risks are nor where the risks originate and that's also something similar where we don't understand deeply how a drug works within the body, but we see the outcomes and we see the outputs and that's similar to foundation models again.
Connor Dunlop:
Just to build upon what Merlin was saying there, because I think that's really a core point that's really interesting for us. It's true that risk originates at any part of the AI value chain and I think that's what's really interesting and what we were taking from the FDA model that they covered the whole lifecycle, starting from discovery and development through reach of preclinical research and all the way through to post-market monitoring. So I think when you accept the premise that AI risk can originate and proliferate in the value chain, then I think this type of rigorous oversight covering that whole value chain like the FDA has, becomes a really interesting model to learn from.
Justin Hendrix:
So the more risky and more novel a product, the more tests, evaluation processes and monitoring it will undergo you say. How would you maybe characterize in more detail what FDA style approval and oversight means? So what would be the kind of specific processes that you would see coming over from medical device approval or pharmaceutical approval that would apply for AI?
Merlin Stein:
We see five dimensions that we characterize as FDA style oversight and we are of course aware there's a huge debate around the FDA and how it's overseeing. There're lots of institutional aspects of it, but we focus more on the process design of it and that means first risk and novelty driven oversight that have different classes and based on how novel or how risky a new product is, the oversight is stronger. Second is a continuous and direct engagement of the developer with the FDA throughout the whole life cycle as Connor currently mentioned. Third is a wide-ranging information access. So we spoke with some medical oddities who are partly scared of the FDA and see the FDA because of this wide information access as the regulator with tooth.
Fourth, it's the burden of proof that is actually on the developer and it's not just a transparency regime. And finally, and maybe most importantly, fifth is somewhat of a balance that's of course highly discussed, but a balance between innovation with efficacy and safety and not being a regulator that is very strictly banning everything but balancing these powers.
Connor Dunlop:
I think last time I spoke to you Justin, we talked a lot about the challenge of information asymmetries and especially in my work in the EU, that's been something that regulators now have been grappling with. How do we reduce these information asymmetries between developers and regulators and the wider ecosystem? So I think you mentioned the approval process that the FDA uses, the pre-market approval process. I think that's a really helpful way where you can start to reduce those information asymmetries because it creates a logical point before risk proliferates where a regulator can get access to high value information and then with this sort of engagement at the approval gate, this is how the FDA really upskilled and learned over time.
And I think I maybe mentioned it there, but just to reiterate, I think the interesting thing to take from that approval yet, is how the burden of proof is put on the developer to prove safety and efficacy. I think when I look at how AI is being regulated across jurisdictions right now, the challenge is that the burden of proof is often not on the developer, it's on a regulator. Even in the UK with the AI Safety Institute, for example, right now, they have agreed to get early access to foundation models, but it's just for the AI Safety Institute to prove and scrutinize to find any risks, but that puts the burden proof on the regulator. I think flipping up burden of proof, getting the developer to show the regulator that the system is safe, I think that's a very helpful learning from the FDA.
Justin Hendrix:
Right now you've got basically this mode of operating that seems to be, develop a foundation model, put it out into the wild, see what goes wrong, fix it after the fact. That's basically the approach that most firms are taking at this point and it seems like there's hastening that process because of competitive pressures. If you look at just this week, and I don't know if either of you have looked at it, the sort of preparedness framework that OpenAI has put out, really talking about its technical and procedural safety infrastructure under the premise that its systems are getting closer and closer to artificial general intelligence, this idea of being able to put things out into the world and then explore the safety and risk phenomena that come back once it's out there. Wouldn't this sort of, I don't know, just radically change the way industry's going about things?
Connor Dunlop:
It basically would very heavily run against what we've seen so far. I think that's one of the main reasons for scoping this project, and you alluded to it, the experimental nature of the technology and OpenAI does explicitly say that they put this out into the world and they learn and iterate and go from there. We think if the scale of the risk that OpenAI indeed itself would say the technology could pose, we think that's not the ideal model for going about this.
We think that if there is a risk to life, if there is wide scale pervasive, systemic risk from these models, which we believe that there could be, then it makes much more sense to focus on safety and efficacy before going to market, slower rollout. You can have early access for researchers for example, and this is a good way to learn iteratively without doing sort of wide scale testing on the general public because it's very hard to roll that back if something goes wrong.
Justin Hendrix:
Folks who would be critical of the FDA would say high cost of compliance, enormous amounts of money spent, especially in the upfront process of even getting a drug or a medical device into the pipeline for consideration requires so much capital to get going. You're really harming innovation. That's the great cost of this model, right? I don't know, how do you answer that kind of critique?
Merlin Stein:
We would even go beyond that and say limitation's not just with the cost of compliance but also that the FDA model doesn't cover all of the risks. It's mainly for risks that are clearly discoverable in clinical trials and clinical trials being some sort of a proxy for what will happen later on. Of course there are monitoring regimes and similar that we can learn from, but there are limitations also to the kinds of risks that the FDA model works for and there are limitations when the FDA works very closely with industry, how independent the FDA can be, specifically in niche areas. On the cost of compliance we are very much with you that it's very important to think through, "Okay, how can we make this model as lean as possible while reducing the risk?"
And that's why there are probably two perspectives to take. Number one is this model, the FDA model as we see it today is nothing that grows from zero to 100%, but it was a hundred-year history that slowly built up and what we are advocating for starting this build up and we don't have a hundred years for AI, so we need to start it now and start it fast. And number two, is if we specifically look into the numbers and for the EU AI Act, there's been The Future Society who checked what would be the compliance costs for example, and they calculated that for the models with systemic risks, the ones with the pedagogues regulations that are still below our suggestion of the FDA model, but that's less than 2% and that means that there is a cost of compliance that is aligned with the cost to society because of the risks of these models.
Connor Dunlop:
If I could also jump in on the cost of compliance, I think this is a very interesting question because when you look at foundation models, this sort of ecosystem as it currently exists, I think that it's not actually just the cost of compliance that would tend to lean towards possible oligopolies or monopolies. It's really looking at that sort of the structural layer behind foundation models like the sort of data and compute needed to actually get in the game. Just for example, how expensive the large training run is, hundreds of millions potentially. So I think it's possible that there is a tendency towards oligopoly and monopolies already and then if that's the case, maybe then you can be more open to having very strong scrutiny of those players. But then again, I think it's not certain that's the way we do, but it's possible given the cost of getting into the game, that this is what we would see.
And yeah, maybe just one extra point on that. I do think there are some learnings already from other jurisdictions on how we can prevent some of that tendency towards oligopoly. The European Medicines Agency for example, which is like the European equivalent of the FDA, they have an SME office that would provide regulatory assistance and potentially reduced fees for compliance. So I think there's some elements around that which could be explored as well to try and reduce the risk of oligopolies and monopolies. But yeah, it's definitely something to think hard about.
Justin Hendrix:
One of the things I like about this report is that I feel like I learned about how the Food and Drug Administration works from reading it, which shows that you didn't just do a kind of cursory look at the FDA as a kind comparative model, but there's all sorts of things here that you lay out as possible mechanisms for regulation or the evaluation of AI systems that are drawn from what you call FDA inspiration. So everything from third party efficacy evidence, adverse events reporting, to the way that clinical trials work, pre-specified change control plans, lots of different specific programs that the FDA uses. Are there examples from that long list of FDA inspiration that you would offer the listener as things that you really think AI developers should be using?
Merlin Stein:
The interesting part is that lots of the suggestions of what is currently discussed in the area of governance debate, they are very close to where we already have a very clear process defined and I would say to lay out two of them. One is the clinical trial style almost, not popular necessarily, but most important part of the FDA, which is very similar to sandboxing for AI to really have a deep visibility into the model before release. And I think that's a specific thing that is, for example, currently being tested in Spain and that is a specific thing. I think that's very important.
And the second part is if you think in terms of post monitoring and adversarial reporting, we talked with 20 experts that were partly FDA cheap concepts who developed these programs, but also on the auditee side in this day and what their mindset is because of the post monitoring reporting part, is that they need to report anything where they see, "Okay, an adversary had some access or similar." And I think that mindset change through that kind of adversarial reporting process that we see of the FDA, I think that would be very important for AI governance.
Justin Hendrix:
So let me ask you about whether you think that this FDA style process or FDA style oversight needs to be run by a singular FDA style entity. A lot of folks are thinking about whether it's best to look at these problems from some singular regulatory entity or whether it's best to devolve those types of oversight to agencies that have specific sectoral expertise. Is implicitness the idea that you think there should be, a centralized agency?
Connor Dunlop:
I think first of all, it depends which jurisdiction you're thinking about. I think the reason why you might need a standalone FDA style regulator specifically for foundation models is that there is solitaire territories in some sense because there is no established state of the ARCs and not really established standards on what safety and advocacy looks like for foundation models specifically. I think also given like you mentioned, it's not just in that they're very general purpose, can be adapted to a wide range of contexts. I think that might be a reason to say that you need some centralized capacity to look specifically at this type of AI model. I think there's been recognition of that in the UK because that will be the scoping of the AI Safety Institute. Also in the EU they're setting up an AI office and it'll have specific remit to look at foundation models or general purpose AI.
So I think that's a recognition that we do need some additional centralized expertise to grapple with those models specifically. I do think then, taking a holistic approach of the whole value chain, I think we do have then more sector specific capacity and expertise and then this could be how we would envision it in our models. This could be what a pre-market approval process would look like for specific applications that build on top of or adopt the foundation models. That's where I think you could tap into existing regulator capacity. I think it could be quite a holistic way to govern these models.
Merlin Stein:
We can't apply the FDA model one-to-one and just copy the 18,000 people to set a big central agency. I don't think that's actually needed. The important thing is to think what is being regulated already on a sector specific site with sector specific regulators, but where does it make sense to look at foundation models that are definitely later use in many different sectors centrally and creating that kind of central capacity.
Justin Hendrix:
I recognize that the metaphor between medicine and AI doesn't always hold up. One of the places I'm interested in your perspective on where that tension might really spring up is around maybe some of the recommendations you have around ideas like post-market safety monitoring. For instance, one of your recommendations is around the idea that developers should enable detection mechanisms for outputs of generative foundation models. That is certainly something that I suppose we would hope that most model developers would do, that they would make it possible for us to track the providence of material or code or images or whatever that's produced by the system. But how realistic is that? We see a lot of folks arguing essentially that in just a few years time it will be even more difficult to necessarily track the outputs of generative foundation models as they get better and as the outputs get more and more complex. Is that a kind of challenge to this way of thinking?
Connor Dunlop:
Yeah, I think that is definitely something to consider. I think being able to detect outputs is something to aspire to. I think in the context of the EU as well, that they have at least added a requirement for someone producing a deepfake to label it. But I think the challenge of any of these types of regulatory approaches is you can set rules and guidance for companies, but also this is always based on the premise that you're regulating a company or someone who's an actor with goodwill, a goodwill actors. Going beyond that with regulation, I think we should have that basically as a safe works, but then when it gets into the territory of bad actors, they're not going to label their deepfakes for example. This is where you need an ecosystem approach and that's where I think the post market monitoring learnings of the FDA becomes very relevant.
It's not just on the regulator, it's on the wider ecosystem to report adverse events like mentioned. I think that's the type of thing where, if you project forward a little bit, we might need similar trusted flagging mechanisms in social media, all these types of things. Yeah, it's going to take an ecosystem approach and we're not there yet, but it's good to start having these conversations now, actually.
Justin Hendrix:
Yeah, and I guess if you really do take the metaphor forward, if folks are cooking up pharmaceuticals in their basement, we have other mechanisms to police that in society. I wonder if we would take that metaphor in that direction that we would see, what's the word unsanctioned or unapproved sort of use or development of foundation models as something that needs to be monitored in the same way that we would use monitor, like folks making meth.
Connor Dunlop:
Yeah, it's a very interesting question. I think one interesting thing that I've observed from a far, at least with the foundation model developers are the ecosystems exists today. There seems to be more willingness for them to commit to, like the White House Voluntary Commits is one example. It seems like there is some appetite for them to try and do the right thing, at least. I think left for their own devices that will not always be the case. And I think then what we haven't really seen in, and this is to your question, what happens when people defect in this kind of ecosystem? Normally there's been some pressure to sign up to voluntary commits and things like this, but if one company was to say, "Yeah, we're going to train a large model, not do any risk assessment, any risk mitigation, put it out into the world." Yeah, we haven't seen that yet. But it's a very interesting question what a regulator does.
Justin Hendrix:
I know that you, in the report suggest that international consideration isn't something you've put an enormous amount of effort into here. You haven't thought about how this would impact international governance of artificial intelligence, but I wonder if there were perhaps some ideas about that, maybe ended up on the cutting room floor that you could mention now. How would, if different kind of regulatory blocks, maybe the US, EEU, others, if they had these FDA style approaches, how do you think that they would knit together or work together across geographies? Is there already a kind of membrane between, for instance, the European Agency that you mentioned, Connor and the FDA, do they share information?
Merlin Stein:
There are two things we could learn on the international side. Number one is that there is lots of joint standard setting and information sharing. And that already happens of course with the ESO standards and similar on the AI side too.
But number two, that there is a jurisdictional certification approach that basically means there's an international organization that's building on top of, within jurisdiction FDA style regulators. Meaning that if the European regulator has certain standards that are very much in line with the American regulator, then there's an automatic acceptance of the approval from the other side. And that very much helped for there to be a kind of natural competition of countries to reach the same level as let's say a European or US MAI regulator. If you are a country that is then, for example, India, that was the case of medicine that they then very much increase their regulatory standards through the European or US level to then have the mutual acceptance.
Justin Hendrix:
One of the things I like about this paper is that at the end you pose a bunch of different types of questions that perhaps you would've spent more time on if you'd had it or others should spend more time on. One that I quite liked was the idea that maybe the FDA, maybe it's not the best model, maybe there are other non-health regulators that are also looking at very serious technical matter that could be a better model. You point to the US Federal Aviation Administration, the National Highway Traffic Safety Administration. Have you thought about that at all? Are there other agencies that perhaps will be report number two or report number three where we might draw inspiration?
Merlin Stein:
There's so many possible agencies you can look at. The three criteria again are, there's a very high risk of public safety and these risks are very uncertain because AI, the area of unknown unknowns. And then the third one is that there are processes in place that already work very well for general purpose technologies. And that's why if you think about them, a specific kind of set of risks where the FDA lacks, for example, you think about systemic redistribution parts and systemic risks, I'm currently thinking a bit about how to apply banking regulation there and how if you think about the third party auditing system, what can we learn from regimes that are happening and maybe also failed as part of the financial crisis?
And what can we learn for specifically third party auditing there? And there will be a report in the next month from me on that side. But I also think, yeah, Connor, you might have more to share from the Ada Lovelace institute side who's also looking in lots of case studies among different regimes currently.
Connor Dunlop:
Yeah, we're looking at certain angles. I think one maybe to highlight regarding this third party auditing and this having a very strong ecosystem supporting a regulator is looking at automotive and specifically where we're looking at how Germany has built up this sort of automotive ecosystem based on trust and safety and they really became a global leader in that sphere, but leaning very much on this third party ecosystem of inspection. So I think that's an interesting angle because especially in the European context, there's a lot of conversations around EU competitiveness and how as for the EU can catch up with the US and I think there might be some learnings there to say, "Okay, you can catch up by leaning into trust in CFD, leaning into a third party ecosystems. Europe has done that before in automotives and in Germany. So I think that's one angle we're going to look at next to see if there's other interesting learnings.
Justin Hendrix:
And Connor, that's really my last question, which is we last talked about the EU AI Act before the final trial log, before political approval just a couple of weeks ago now. What do you see in the AI Act? Does it in any way, I suppose, contain within it the seeds of some of the ideas that you advance in this report?
Connor Dunlop:
Yeah, there's a lot of thoughts on that, but yeah, I think there is definitely some elements that could map onto the FDA model. So it seems like, for a foundation model specifically, they are taking a less, what some people have called prescriptive approach to foundation model governance. So they've left it quite loose in terms of how some of these models will be governed.
They say they need to do some systemic risk assessment, systemic risk mitigation, but precisely saying how that looks is a little bit TBD. How they're going to do that, I think it's related to how the FDA has a sort of co-regulatory approach. So if we take the example of approval gates with the FDA, they develop on the regulator, co-develop what EFD and efficacy endpoints will look like for pre-market approval. But with this co-development approach, and I think what the EU is leaning into is to say, "Okay, we're not doing excellent data requirements very heavily on foundation model, but what we're going to do is what they call a code of practice." So that means basically the developers and the regulator will get around the table and try and work together on what safety and efficacy should look like. I think there's a lot of issues around codes of practice in terms of you don't really want developers drafting the law, so to speak.
And then there's also a big challenge there around the fact that they are not initially binding, so it doesn't really have teeth. But I think if those can be mitigated, for example, by ensuring civil society has a strong say by ensuring that they did cover, become binding within a certain timeframe, I think this cool development approach, similar to the FDA can potentially become interesting and it seems like the EU is leaning into that. And maybe one quick other one, I think they are also looking very heavily at the governance, basically the governance piece. So they're setting up the AI Office to specifically take this arm on foundational governance. So it's a little bit, kicking the can down the road a little bit, but putting in that framework to say, "Okay, if and when they need stronger intervention, they can hopefully do that."
Justin Hendrix:
Merlin, any final thoughts? You're completing your PhD at Oxford. How will this fit into your dissertation and what's next for you?
Merlin Stein:
For me, it's very much about understanding the kind of trickiest risks where we don't have much existing models that work well, which is the kind of systemic over-reliance risk side of using foundation models too much, of them being too much integrated in society. And there's lots of research questions, how to address these, how to identify these, and I'm super curious to see what will come on this side and to be super open for collaborations on that side.
Justin Hendrix:
I suppose you and Connor and perhaps myself will be in business thinking about these things for quite some time. I'm grateful to you for taking a moment out just before the holidays to talk to me about it today. So Connor and Merlin, thank you so much and happy New Year.
Connor Dunlop:
It was a pleasure. Thanks a lot Justin, and hope to talk in 2024.
Merlin Stein:
Thank you Justin. See you soon.
Authors
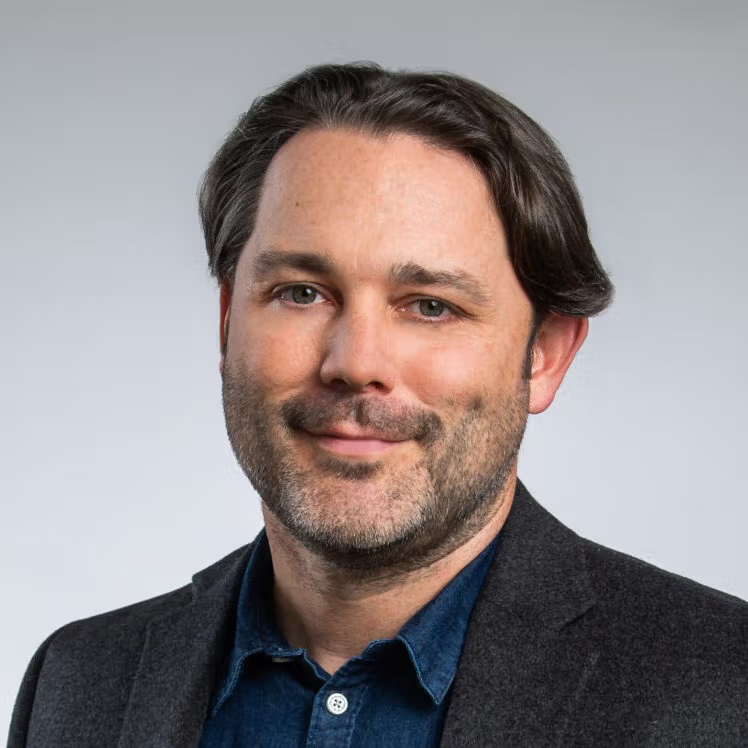