AI Won’t Replace Doctors, But It Can Help Them Improve End-of-life Medical Care
Meha Ahluwalia / Jan 4, 2022Meha Ahluwalia is the Project Manager of the Aspen Tech Policy Hub.
In Leo Tolstoy’s 1886 novel The Death of Ivan Ilyich, after falling from a stepladder, Ivan is visited by progressively expensive doctors who torment him with one unsuccessful treatment after another. As he grows increasingly feeble, Ivan realizes his death is imminent and pleads with his doctors to stop their relentless poking and prodding. More than anything, he wishes to be consoled and petted. Puzzled over his condition, the doctors dismiss Ivan’s requests and fail to provide him the comfort he longs for before he dies.
But if Ivan were injured today, he might be more satisfied with his healthcare. As artificial intelligence (AI) capabilities in medicine advance, physicians could gain help in identifying patients who would benefit from conversations about end-of-life care. Policymakers must move quickly to develop frameworks to govern such applications.
AI in medicine
Today’s term for what Ivan sought is palliative care. When a patient’s health declines and curative treatment is no longer possible, the healthcare calculus changes. Instead of exhausting every attempt to extend their time, patients often prioritize comfort and preserving autonomy. Palliative care specialists, armed with medical knowledge and experience, provide treatment that emphasizes quality of life according to patients’ wishes.
Yet while most people hope to have such care for themselves and their loved ones, only 14% of those needing palliative counseling receive it. Further, while 80% of patients would prefer to die in their own homes, only 20% do. These discrepancies occur in part because physicians have difficulty predicting death. Dr. Nicholas Christakis at Yale University found that in a study of 343 physician’s survival predictions for 468 terminally ill patients, only 20% were accurate. Many physicians expected patients to live five times longer than they ultimately did.
Some scientists believe AI can help. Researchers at Stanford University developed a program to predict the likelihood of a patient dying within 12 months. By utilizing supervised deep-learning techniques, the algorithm derives insights from vast electronic health data sets of previous patients. It then assesses a given patient’s electronic health record from the past year to generate a projection of the patient’s mortality. A team at the University of Nottingham created a similar program to predict the fates of chronically ill patients, achieving a higher rate of accuracy than physicians’ own estimates. By using these programs to highlight patients who may be sicker than we think, physicians can help more critically-ill patients die on their own terms.
AI’s potential in medical diagnosis largely stems from its ability to pattern-match more efficiently and accurately than humans. Research suggests that because AI can collect, synthesize, and derive insights from massive amounts of data, it can improve the diagnostic process significantly, particularly for medical specialties heavy on categorization. While physicians can view a few hundred images per hour, AI can process millions of images almost instantly. This contrast in scale can make a meaningful difference.
Take aneurysms. Without corrective surgery, aneurysms become fatal extremely quickly. Of the roughly 30,000 ruptured brain aneurysms in the United States each year, 40% are fatal, and 15% of patients die before reaching the hospital. Of those who do survive, 66% are left with debilitating cognitive disabilities. Detecting an aneurysm requires scouring over 300 images for minute details – a labor- and time-intensive task while corrective surgery becomes harder with each passing minute. The difficulty is that many aneurysms hardly present symptoms until right before they rupture; subtle signs barely register as a blimp in the movie-like succession of images.
AI can handle this task more nimbly. In 2019, a team of researchers at Stanford presented an AI tool called HeadXNet to take on aneurysm diagnosis. The algorithm powering the tool was trained on a data set of 611 head scans. For each voxel (the term for a 3D pixel) of every scan, the scientists painstakingly tagged whether the contents were part of an aneurysm. After learning from these scans, the program demonstrated detecting 6% more aneurysms than human radiologists. The potential advantage that this signals cannot be overstated – a missed aneurysm nearly always results in death.
Other research shows that algorithms can outperform radiologists at detecting cancer. A 2015 study found that pathologists often disagree on how to interpret breast biopsies, resulting in misdiagnosis of one in six patients with a particular type of non-invasive breast cancer. Researchers at the University of Washington and the University of California, Los Angeles developed an AI program that diagnosed breast cancer biopsies correctly 89% of the time, compared to a 70% success rate of pathologists. Similarly, a Google algorithm detected lung cancer with 94% accuracy, reporting far fewer false positives and false negatives than physicians did. In both examples, the researchers attributed the AI’s success to spotting minuscule differences in images that humans are unable to discern. Certain details that were hidden or unremarkable to radiologists constellated patterns when the algorithms compared millions of similar images.
Ensuring equity, justice, and humanity in AI decision making
AI won’t remove the need for humans in diagnosis and treatment, but it will alter how they carry out their tasks. When AI is applied in this context, it’s considered “software as a medical device” (SaMD). Even with these additional devices, doctors will still need to review AI recommendations, evaluate their applicability to the situation, and decide how to proceed. And patients should always have the right to appeal the determination to a human.
Specialties like palliative care highlight the potential for AI in healthcare especially well. Developing care plans requires more than knowledge of biology and statistics, but the interpretation of these factors in the context of unique patient’s needs – a process that cannot be automated. Creating room for AI and humans to complement each other’s strengths enables earlier, more accurate diagnoses. Then, doctors can better understand and care for their patients.
However, before these technologies can be deployed widely, several concerns must be considered.
As AI models learn from the medical histories of previous patients, they should not compound the biases that have contributed to disparate health outcomes. A 2017 systematic review of research found that physicians have an overall preference for White patients. One study even concluded that Black Americans are less likely to be treated properly for heart attacks. Racial minorities, especially Black people, are also burdened with disproportionately high incidence and mortality rates for breast, kidney, and prostate cancers – diagnostic challenges that are seen as some the most ripe for AI’s assistance. It is crucial not to replicate existing biases.
Programs boasting impressive accuracy rates must also be auditable. For example, Stanford researchers acknowledge that AI models struggle to distinguish causal relationships. When researchers trained an algorithm to identify cancerous moles, the model noted that many photos of malignant moles contained rulers. The algorithm deduced that rulers signal cancer and used that logic to fuel its decisions. In actuality, physicians had added rulers to measure moles they suspected might be cancerous. Unlike doctors, who derive their knowledge from a repository of medical training and real-word experience, AI algorithms largely teach themselves. While AI is capable of drawing far more connections than can humans, this also raises the chances of bad associations. Algorithms with high detection rates are exciting, but researchers and AI-adopters must be able to scrutinize and verify results.
A 2019 study exposing bias in a widely used healthcare algorithm underscores how crucial this is. Researchers found that an algorithm many hospitals and insurance companies use to identify high-risk patients in need of specialized care overlooked Black patients. The model used healthcare spending as a proxy for need, not accounting that the US healthcare system has historically put less money towards caring for sick Black patients than healthy White ones.
Though the promise of AI to enhance healthcare is immense, these issues illustrate why thorough research and careful deployment of this technology is necessary. AI will continue to expand into decision making across all verticals, and healthcare will furnish use cases with some of the highest stakes. As policymakers deliberate regulation of emerging AI technologies, they should specifically consider medical applications to ensure they support the march towards responsible, accurate, and equal care.
Policymakers must act
A few regulatory bodies are attempting to do this. The European Commission’s Artificial Intelligence Act, introduced last April, seeks to categorize proposed AI uses as minimal, limited, high, or unacceptable risk. Healthcare AI applications are expected to fall under the high risk category, requiring them to pass a high bar of privacy, transparency, and oversight standards. Healthcare AI applications would also be subject to other medical sector regulatory processes; onlying after clearing both the AI-specific and sector-specific processes would a tool receive approval. The Artificial Intelligence Act is currently under legislative debate.
In the US, the Food and Drug Administration (FDA) put forward its Artificial Intelligence/Machine Learning-Based Software as a Medical Device Action Plan last September. Though not a regulatory framework itself, the plan outlines the steps the FDA intends to take toward developing such policies, using a combination of further research, patient-centered design, draft guidances, and pilots. It will likely be a few years before the outcomes of the FDA’s efforts are revealed.
Tolstoy never tells us what Ivan’s diagnosis was, but perhaps AI could have. And if Ivan’s death was inevitable, AI could have helped his doctors and family accept this reality and care for him as he wished, with comfort and relief. Leveraged responsibly, artificial intelligence can extend medicine’s reach to provide faster, better care for more people. Policymakers must urgently make way for the benefits of the technology while protecting against the risks.
Authors
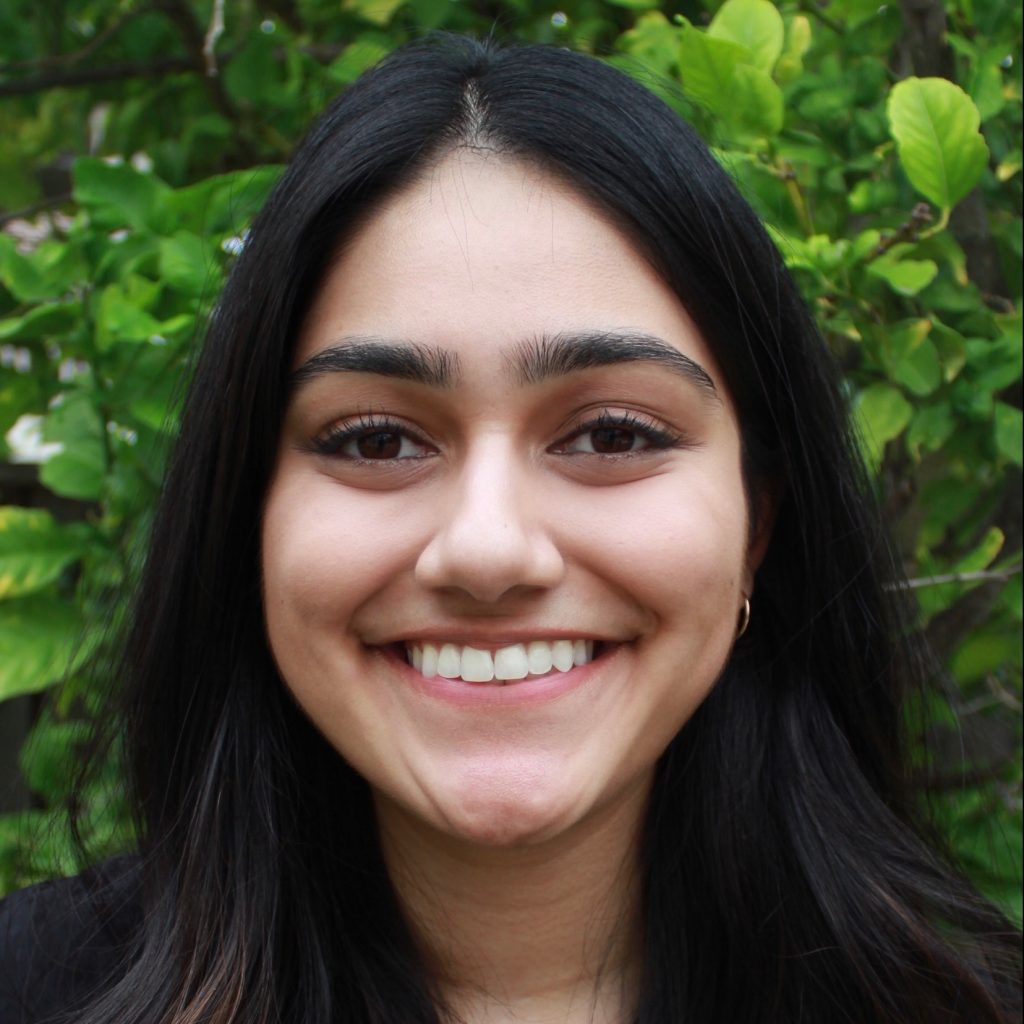