AI Snake Oil: Separating Hype from Reality
Justin Hendrix / Sep 29, 2024Audio of this conversation is available via your favorite podcast service.
Arvind Narayanan and Sayash Kapoor are the authors of AI Snake Oil: What Artificial Intelligence Can Do, What It Can’t, and How to Tell the Difference, published September 24 by Princeton University Press.
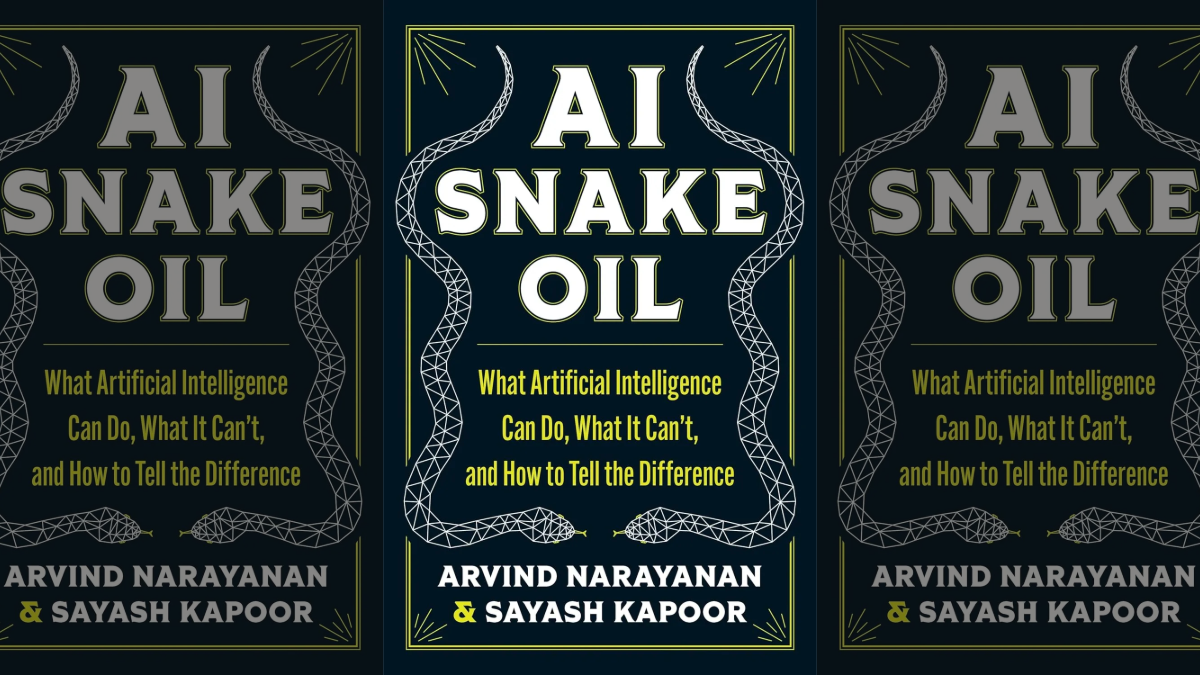
What follows is a lightly edited transcript of the discussion.
Justin Hendrix:
According to historian accounts, in 1893 at the World's Exposition in Chicago, a man named Clark Stanley took a live snake and sliced it open before a crowd of onlookers. He then put it into boiling water, skimmed off the fat, mixed it up, and told the crowd that was a cure All but the bottles of Stanley's snake oil he sold to the crowd didn't contain any snake oil at all. Eventually, the Pure Food and Drug Act of 1906 was passed to minimize the sale of untested medicines being sold around the country. Then in 1917, federal investigators found that Stanley's Miracle Snake Oil actually contained mineral oil mixed with a fatty oil that appeared to be beef, fat, red pepper and Turpentine.
Stanley was only issued a fine, but the idea of snake oil as a product that doesn't live up to its promise took off. My guests today say many of the sellers of AI systems are more or less modern day Clark Stanleys. They say AI snake oil is AI that does not and cannot work, and they set out to help their readers understand it, and distinguish it from what they see as the real promise of AI in the long run. They say AI snake oil does us a favor by shining a spotlight on underlying problems with today's AI systems, as well as many of the concerns we have about AI's role in society.
Arvind Narayanan:
Arvind Narayanan, I'm a professor of computer science at Princeton University and the director of the Center for Information Technology Policy.
Sayash Kapoor:
I'm Sayash Kapoor I'm a PhD candidate at Princeton University in the Department of Computer Science and a researcher at the Center for Information Technology Policy.
Justin Hendrix:
And you are the two authors of AI Snake Oil: What Artificial Intelligence Can Do, What It Can’t, and How to Tell the Difference. You get into everything from myths about AI and why they persist. You get into questions around whether AI should be considered an existential threat, et cetera. Arvin, I saw you quoted in the Washington Post in a kind of curious article, and your response in this article I think summed up for me maybe the vibe of this book in a way.
So I'm looking at this headline, "Mayoral candidate vows to let VIC, an AI bot, run Wyoming’s capital city." So this chap, Victor Miller, has apparently set up some AI chatbot that he thinks could perhaps run for office and do a good job, and apparently he was shut down by OpenAI but went ahead and found himself another API to plug up to. You were asked about this and I loved your response. You said, "it's hard for me to talk about the risks of having an AI mayor. It's like asking about the risks of replacing a car with a big cardboard cutout of a car. Sure, it looks like a car, but the risk is that you no longer have a car." I felt like this quote summed up to me the vibe of this book.
Arvind Narayanan:
I did give that quote to the Washington Post reporter and then I regretted my snarkiness a little bit. I very much stand by the substance of that quote, though we say this in the book as well, we make a related point, which is that broken AI is appealing to broken institutions a lot of the time. What we think of as an AI problem is actually something deeper and we look at a number of examples why, for instance, automation is so appealing in hiring because companies are getting hundreds, perhaps thousands of applications per position and that points out something that's broken in the process, but then it seems appealing to try to filter through all of those candidates with AI. And even if AI in those context is not doing much, we think that a lot of these AI hiring tools are just elaborate random number generators from the perspective of an HR department that is swimming in the sea of applications. It's done the job for them. It gives them some excuse to say we've gotten it down to these 10 candidates, and so there are often underlying reasons why we think broken AI gets adopted.
Justin Hendrix:
You have a section on who this book is for. I assume the tech policy press listener is squarely in your consideration zone for who this book is for, but who else is it for Sayash?
Sayash Kapoor:
So broadly speaking, I think his book is for anyone who is curious about what AI can and cannot do in their own lives. In particular people who are looking to adopt AI in their institutions, people who are looking to see what AI can do in their day-to-day. And to be clear, despite the title AI snake oil, both Arvin and I are pretty optimistic about some types of uses for AI. We think especially when it comes to knowledge work, AI has a big role to play when it comes to the future and in some sense it is a very forward looking book. We we're trying to lay out a vision for a positive future of how people can incorporate AI into their lives and how they can avoid falling for AI snake oil.
Justin Hendrix:
Arvind, you already referenced one of the big ideas that I want to make sure to come back to in this conversation, this idea that AI snake oil is appealing to broken institutions. So I'm going to come back to that one, but I thought one way into some of the themes of this book that might really be appropriate for my listeners. In particular, your chapter six focuses on why can't AI fix social media? So that's the kind of core question that runs through this chapter and follows more or less the rubric that you put forward in other chapters as well where you consider different questions around AI and the extent to which it lives up to the hype.
You start off pointing out Mark Zuckerberg back in 2018, he's in front of Congress, he's trying to explain away all the various harms of social media and he's bullish on AI as the answer to a lot of these problems. And of course even then there would've been lots of machine learning classifiers running on Meta's platforms before it was Meta screening out hate speech or CSAM or other types of things. But it strikes me right now at this moment where folks are talking about large language models and content moderation, a lot of enthusiasm out there, a lot of vendors at the trust and safety or TrustCon conference this year hawking various LLM tools for content moderation. Why can't AI fix social media?
Arvind Narayanan:
So as you pointed out, Justin, AI has been used in content moderation for a long time, and we started out by acknowledging that for well over a decade since the beginning of content moderation, companies have looked to automated tools simply because the scale is so vast and without some amount of automation, the system simply won't work. But when we started looking at the reasons why AI hasn't obviated the problem so far and looked at what might change in the future, we started to quickly realize that the limitations were not about how well the technology works, it's really about what we mean by content moderation and what we want out of it. But for me in particular, this crystallized when I was reading Tarleton Gillespie's book on content moderation where he gives this example from, I want to say maybe around 2016, 2017, there was a controversy on Facebook when a journalistic organization posted the so-called Napalm Girl Image.
It's a horrific image of the Vietnam War that everyone has seen or heard of, and Facebook took this image down and there was an outcry and people initially assumed that this was an enforcement error, perhaps an error of automated enforcement, a clumsy but or can only see this as nudity because it's a classifier that has been trained to do so but is unaware of the historical significance of this image. But what Charleston points out is that was absolutely not the case. This was an image. Not only had this type of image been discussed internally by Facebook's policymakers in terms of what they can and can't have on their platform, this specific image had been discussed and was part of the moderator training materials. And Facebook had decided that despite its historical significance for whatever other countervailing reasons, this image can't stay on the platform. And I thought this particular misunderstanding that a lot of people had, including myself back when I heard if this controversy really captures what we misunderstand about social media content moderation, it's a hard problem not because the individual instances are hard, but because as a society we can't agree about what we want out of content moderation.
And so that's really the overarching theme of the chapter and we get at it in various ways.
Justin Hendrix:
You asked the question, so why can't AI take over content moderation? Why hasn't AI already solved the problem? There are folks who are enthusiastic about especially the application of LLMs, and there now are products available from OpenAI and as I mentioned various kinds of vendors that are trying to apply these in different contexts. One of the things that they're always pointing to is that this human content moderation is such a wretched job and it has a real psychological and in some cases physical toll on the people that do the work. You end up in a place that still strikes me in this chapter as recognizing just the necessity of human intervention here. What is it that makes you so certain that at least in the near term, we're going to have to rely on real people to do this work?
Sayash Kapoor:
I think in some sense the reasons why we'll have to rely on some amount of human interventions and the same as the sort of broader argument of the book that content moderation is not a monolith. So within content moderation, we might have specific tasks, things like detecting nudity in image, things like detecting. If an image contains a certain offensive hit speed symbol, for example, that might be very easily solvable using ai. In fact, we are quite optimistic that AI will continue to play more and more of a role in doing this type of detection work, but the place where human intervention becomes necessary is in drawing the line of what constitutes acceptable speech for a platform. So in the example that Arvin just shared, I think it is really hard, it is a statement about values when it comes to what Facebook as a company wants to endorse or what Facebook as a company wants to accept on its platform rather than about detecting what's in an image. For example, the latter task detecting what's in an image is something we think AI is already very good at, but it'll continue to get better. But the former task of deciding what the values of a platform are and what constitutes the boundaries of acceptable speech, I think that's the place where humans will continue to be involved, at least for the foreseeable future.
Arvind Narayanan:
And to clarify one thing to your question, Justin, of whether a lot of the gnarly labor of tens of thousands of people, particularly in low income countries, can that be automated? We do think a lot of that can be automated. We don't think it's entirely going to go away. Sayash talked about the policymaking behind content moderation and on the enforcement side, there's always going to be a need for appeals, a completely automated process regardless of what its accuracy is measured to be in some abstract sense. It requires I think the human element of hearing someone out when they say that they have been wrongly censored. So on the enforcement side as well, I think human involvement is going to be necessary. We think it would be a mistake to completely eliminate people from the enforcement side even if it were somehow possible because another factor that we point to is whatever policies the company has come up with are going to be constantly tested by new types of speech that evolve on these platforms. And so to be able to make new policy to deal with those new situations, you need frontline workers, so to speak, who are looking at on a day-to-day basis. What are the new challenging gray areas that arose?
Justin Hendrix:
Yeah, I enjoyed that part, especially this kind of thought around human ingenuity and the extent to which people are always going to be testing how to get round content moderation systems, even if they do get more and more culturally competent or have more available context in which to make decisions, et cetera. I found myself when I was reading that part, thinking about the Shanghai lockdown protests and the extent to which despite China's kind of well known censorious approach to social media and the internet, it's much more perhaps resourced, we could argue, backbone for taking down the content they regard as violative or antisocial or whatever couldn't contain the human ingenuity. That was the real lesson to that to me, that no matter how much money and people you put into trying to take down certain human utterances, people will always find a way around it.
Sayash Kapoor:
Absolutely. And we see this all the time even with social media platforms outside of China. So in the book we point out that we have pro ana communities where people discuss eating disorders. In some cases Facebook and other social media platforms want to clamp down on discussions of eating disorders because of a lot of pushback they received. And so these communities have found ways to circumvent them by using slangs, what are essentially slangs to discuss topics that are essentially censored by most social media platforms. In some cases these are benign. In other cases it could be argued they're harmful, but whatever the case may be, it's undeniable that when it comes to adversarial responses, when they need to, people have become very good at trying their way, finding their way around these moderation techniques.
Justin Hendrix:
Again, sticking with this chapter six, sticking with this question of why AI can't necessarily do content moderation, you actually suggest that adding regulation to the mix as we're seeing happen around the world, things like the Digital Services Act or the various online safety bills or now in the US proposals around kids online safety, et cetera, that will further complicate things. Daphne Keller, the platform regulation scholar at Stanford, said she was playing around with a custom GPT that's been trained on various platform regulations, I suppose, to make various pronouncements on how content moderation policy should work within the content of those. Why is it that you think regulation essentially complicates what AI could do in this space as well?
Sayash Kapoor:
So when it comes to platform regulation, I think there are ways to formulate these regulations that spur innovation in terms of protecting the citizens, protecting the social media users, and then there are also simplistic or naive looking solutions that might upset the balance a little bit. And so when talking about the latter, one of the things that we've written about is how child safety regulations on online platforms have not really fulfilled that promise. So one of these is copper and in particular the practice that social media platforms do not really cater to the under thirteens and preteens when they try to form these accounts. That's basically entirely because platforms do not even want to deal with trying to fulfill the premises of a safe social media environment for kids. What that has led to though is that pre-teens are on all social media like about ages and as a result, we are not really able to create this social media environment where kids can be safe online and anything. That's the risk we run when we are talking about social media regulation, that takes a simplistic view on what it means for people to be safe online.
Justin Hendrix:
One of the things I've found myself thinking about with regard to just the increased application of AI in the content moderation space is the extent to which it might not work very well and the ways that you described. So you've got this really handy chart I think on the seven shortcomings of AI for content moderation. So I would definitely recommend my readers go out and buy the book if only to acquire that chart, but just this thought that we might end up with these sanitized platforms as these things like over classify or over interpret the policies. You can imagine some LLM that's got various laws that it's looking at maybe in real time referencing various online safety laws around the world referencing some utterances from different users referencing the platforms policies all at once, and it may just decide, hey, let's err on the side of caution and end up being the kind of very paternal moderation system.
Arvind Narayanan:
Even if we take regulation out of the picture, we see a lot of that happening. Often people come at social media regulation with this view that the platforms are callous and users are clearly being harmed and the platforms are doing nothing about it. But of course we know that's not the case. It's not because platforms are intervening out of the good of their heart, but simply because it's good for business. Just the bad press around the harms of social media has been such that most platform companies have internal teams who are taking trust and safety reasonably seriously. And I think we have to look at the trade-offs of regulation in that context. We're definitely not anti-regulation, not at all what we're saying, but I think regulation shouldn't come at it with the view as if platforms are doing nothing and regulations are really setting the bar for what content moderation platforms are going to do.
I think a better approach is to look at it as shaping the incentives and we look at a case study of copyright law and how the DMCA shaped the incentives for platforms, notably YouTube. And what we find is that YouTube is much more responsive to the complaints of copyright holders than to everyday users whose non violative videos might get taken down because of the broad sweep of content id. And our worry is that other regulation is going to create a similar effect, which is exactly what you allude to of creating these sanitized platforms. We see this when AI and chatbots, even without a lot of regulation on what chatbots can and can't output, the concerns around safety has been such that companies have chosen to err on the side of caution in our view at least. And there have been many examples of I want to make a bomb recipe or whatever, someone asks a query like that. And because the bot is interpreting those questions very naively and the safety filter is overactive, it refuses that query as being too dangerous because it sees the word bomb in there, right? We see a lot of these misfires happening even without regulation, and we should be thinking about how regulation can contribute to those incentives that can nudge platforms in the side of too much sanitization.
Justin Hendrix:
I do want to move on to that idea that we've talked about earlier, this idea of AI appealing to broken institutions as thinking about that a little bit in terms of the social media platforms, if we think of them as institutions, you talk about there being a crisis of trust at multiple layers with regard to digital communication. We don't trust platform companies. I always find that interesting giving that the social media industry has spawned this profession called trust and safety. How do you explain that idea, that part of our desire to hope that we can trust AI is about hoping we can trust institutions?
Sayash Kapoor:
Very quickly on your point about social media spawning the trust in safety industry. I think it's funny, but actually not at all surprising. If we did trust social media and social media as an institution where something people actually felt they belong to, then they wouldn't need an article trust and safety. And so just by virtue of the fact that they have this organization within social media, I think it shows how lacking they are in trust as an organization or as an institution when it comes to just the broader point about trust and safety lacking in this specific institution. I think as opposed to many of the other institutions where a lot of AI snake oil is concentrated, so things like insurance or teaching or banking. I think for social media in particular, maybe coincidentally, but perhaps not so much, these institutions are also the ones that are building a lot of the state-of-the-art AI.
So I don't think they are in any way being fooled by the false promise of AI, rather, I think they're very intentionally taking business decisions about when and where to deploy ai. One of our colleagues, Rumman Chowdhury, has said that on social media trust and safety is actually the key product because when it comes to being able to communicate openly with people, no one wants to visit a social media platform with trials or overrun with spam or whatever. And so in some sense, a lot of the AI that does work well within these organizations is because not being able to have a functioning anti-spam filter, for example, would make these social media websites useless. So that's I think the easy part of content moderation. So going back to the idea of easy versus hard, there's also the hard part where I think social media companies and executives have made a lot of tall creams which have not really stood up to scrutiny.
And I think that's where there is this false promise or over optimism about what AI can do. And so throughout a book, I think one of our main goals was to look at the nuances of different institutions and what within these institutions, what AI is currently being used for what it's been promised it's being used and how to tell them about. And I think in social media we have a clear case of some parts of it are working extremely well. Some parts of you're not working at all and executives using this blanket statement to conflate it through. Sometimes.
Justin Hendrix:
Sayash, I know you're spending more time amongst policymakers there in DC. Arvind, I believe you were one of the individuals invited to those Senate AI insight forums hosted last year. The two of you are meeting government officials who are trying to discern what to do in this space as well. I want to ask this question, but maybe just stepping back and thinking about the type of trust that government officials are putting into AI these days. I get it. If you are trying to solve the world's problems with the commanding heights of the federal government and someone comes along and tells you, I've got an abundance machine and it's going to sort the environment and it's going to sort poverty and it's going to sort access to healthcare or mental health or whatever, sounds pretty good. I can understand why folks would want to get on board, but one thing I found myself thinking, reading your book in particular is that this idea of AI diverting people away from what should be their focus is that what's going on in Washington right now when it comes to ai is it is AI helping divert people away from what should be the focus of political leaders?
Arvind Narayanan:
So there are a couple of angles to this. One is let's look at both the promise and the dangers of ai. And I think on both of these fronts, there's a little bit of a diversion. So on the promise front, it goes back to that Vic chatbot, I think Justin that you opened with, where there's someone running for mayor who thinks the chatbot is going to make these decisions. It's going to be unbiased. And so it can avoid all of these messy political disputes that we have. And part of what I said to the Washington Post reporter is that those problems are hard. The reason that politics is hard is because politics is the venue that we have chosen in our society for resolving our deepest differences and having those messy debates. That's the point. That's how we move forward as a society, no matter how unpleasant it may seem.
And despite the fact that sometimes things get too polarized to an unproductive degree, sometimes they spin out of control, we need to reign that in. But to think that we can simply eliminate politics and have this neutral arbiter is to completely misunderstand the problem that we're confronting to reduce this social problem to a technical problem. It might seem appealing in the moment, but it's ultimately not going to work. And I think a lot of the vision of tech that is being sold to policymakers is just more nuanced versions of this basic misunderstanding. In this mayor case, replacing the whole government with a chatbot, it seems obvious why that's silly, but in a lot of other cases, AI is a solution to climate or whatever. Sure, there might be some technical components to that problem. Designing a more efficient engine, a big component of that problem is a social, geopolitical, economic, all of that stuff.
And there it's not going to be easy to or not going to be possible to reduce it to a technical problem. Now similarly on the dangers of AI, so many of these deep problems that we have in our society, whether it's misinformation, which really a better way of looking at the misinformation problem is a lack of trust. The press is supposed to be the institution that helps us sort truth from falsehood. And really when we say the problem of misinformation, it's not really the fact that there are some bot farms that are spewing misinformation to us. That's not the problem. The problem we should be talking about is what to do about the decline of trust in the press. Now people have lost sight of that and are treating this as an AI problem. Oh, what do we do about AI generating misinformation? Which to us is absolutely completely missing the point. Instead of dealing with this difficult problem, institutional problem that we should tackle, it's instead treating this as a technology problem and then thinking about how to put AI back in the box. It's not only going to work, but it's distracting us from the hard work that we need to do.
Sayash Kapoor:
In some sense, it is understandable why people are thinking about putting AI back in the bottle for a lot of these societal harms, right? These companies are in some cases billion dollar companies, perhaps trillion dollar companies that are spending billions of dollars of money in training these models. And so they should bear what is seen as their responsibility when it comes to these harms. And I think that's why solutions like watermarking, the outputs of AI generated text for dealing with misinformation have proven to be effective, especially in policy circles. We've seen a number of policy commitments, most notably the voluntary commitments to the White House that involve watermarking ostensibly as a way to reduce misinformation. And if you look at it technically, I think watermarking, there's no way in which watermarking works to curb misinformation even in the world where misinformation is purely a technical problem because attackers can easily circumvent most watermarking based attacks.
And so the harder problem, as long as we're avoiding the harder of problem, we're only focusing on the surface level issues of the harms that AI causes, I think it is continuing to stay counterproductive. Of course. This then brings us to the question of what responsibility do AI companies have when it comes to the externalities of the AI models, when it is clear that the harm stems from ai? And there I do think policy makers have started to come around to craft sensible and pragmatic policies around how to curb harms from deep fakes. For example, how to curb harms from non-consensual pornography and so on. And I think that part of the conversation has been pretty productive in my view.
Justin Hendrix:
One of the things that I like about this book and about your work more generally is I think you're asking us to think about the future. You're asking us to think about who gets to define the future. You've got these two kind of alternative worlds in two characters that you represent Kai and Maya, that are alternatives essentially to how we might think about AI and its role in society, or how we might think about deploying these technologies. You also talk a lot about the idea that Silicon Valley is spending so much money to essentially create a version of the future that's on sale and to define what is the kind of bounds of our technological imaginations. Just as a kind of final question to the two of you, do you think there's some hope that we can break the grip of Silicon Valley's sales pitch essentially when it comes to tech and its role in society? How do we end up with something more salient than what's on offer?
Sayash Kapoor:
So I think at some point in the book we wrote that the two of us are optimists about the future of AI, and I remember one of the peer reviews for the book criticizing us on that point, basically asking us why we are taking a position, why we are taking a stance on what we see the future to be. And I've thought about that peer review quite a bit since the time we received it. And I think the basic answer is that we feel that there is this, in this time period right now, when it's at somewhat of a societal tipping point in terms of its adoption diffusion across society, we feel that all of us have a lot of agency. We have agency in how AI is built, what we use it for, and more importantly, not just like the two of us in Princeton or the few of us in DC and policy who are thinking about AI on a day to day, but we feel as if a large number of people around the world, most people perhaps have some agency in how they use AI and what shape AI takes into the future.
And I think that is what primarily transpires at least my hope for this future. I think we've seen time and time again how people have resisted harmful applications of AI in their communities. We've also seen how creatively people have used AI models that have been available and people can run. And within the last couple of years, we've seen so many creative applications of AI across industries that just wouldn't be possible if people did not have access to AI. And so I think all of this sort of fuse this current of optimism beneath the book titled AI Snake Oil, which might be a weird juxtaposition, but if you think about it, if you want to ensure a positive vision for AI in society, then it's absolutely essential that you understand how AI snake oil gets pedaled and how to avoid it so that you can proceed to this path of optimism.
Arvind Narayanan:
Yeah, exactly what s said, and let me also add a personal perspective. I've always been profoundly, I would say techno optimistic and have been public about this on Twitter, and a lot of my long time followers were shocked. Yeah, I very much consider myself a techno optimist. I got into computer science as a major more than 20 years ago because I really believe in the power of tech to shape society for the better. And I started researching the harms of tech because I felt that the way to do that is to get ahead of the harms instead of waiting for them to materialize on a large scale. So that has really informed a lot of my research as well as our approach in this book. And now in my personal life as a parent, we've had a lot of conversations about the role of tech in our children's lives as a family, and both of my kids have had their own iPads since the age of one or two.
We're very tech forward, and we think the best way to deal with some of the harms of tech in children's lives like addiction is to introduce the tech early and for it to always be monitored and to use that as an opportunity to impart the skills around how to use devices for learning and how to avoid addiction and so forth, which we try to do pretty much on a daily basis with our children. And it's the same thing with AI. I use AI a lot with my kids when we're going on nature walks, we use AI to learn what species of trees something is or a bird or whatever, and it really nutritious this thing that seems the antithesis of tech going on nature walks. But AI has really actually enriched that. And I'm building AI-based apps for my kids to learn phonics and so forth.
It's not that I'm not teaching them phonics, I am too, but I think there are things an app can do that's actually harder to do without an app. So we've had not only this approach of optimism, but also specifically of embracing the technology of seeing AI as something that can be a very positive thing in our lives. We're not calling for resisting AI. That's not what the book is about. But through all that, recognizing that to do this requires constant vigilance about not just specific snake oil products, but also broad ways in which AI could shape society for the worse and have systemic risks and that sort of thing. So the book is primarily about the risks that we should avoid, but the perspective that led to the book is definitely one of optimism.
Justin Hendrix:
You say in your conclusion, we are not okay with leaving the future of AI up to the people currently in charge, which I appreciate. And then you invite everyone into shaping the future of AI and its role in society. I hope my listeners will go and check out this book. I do think of them as having some role in some claim, certainly in this conversation. Arvind, Sayash, I thank you for speaking to me about it today.
Sayash Kapoor:
Thank you so much for having us.
Arvind Narayanan:
Thank you. This was a wonderful conversation.
Authors
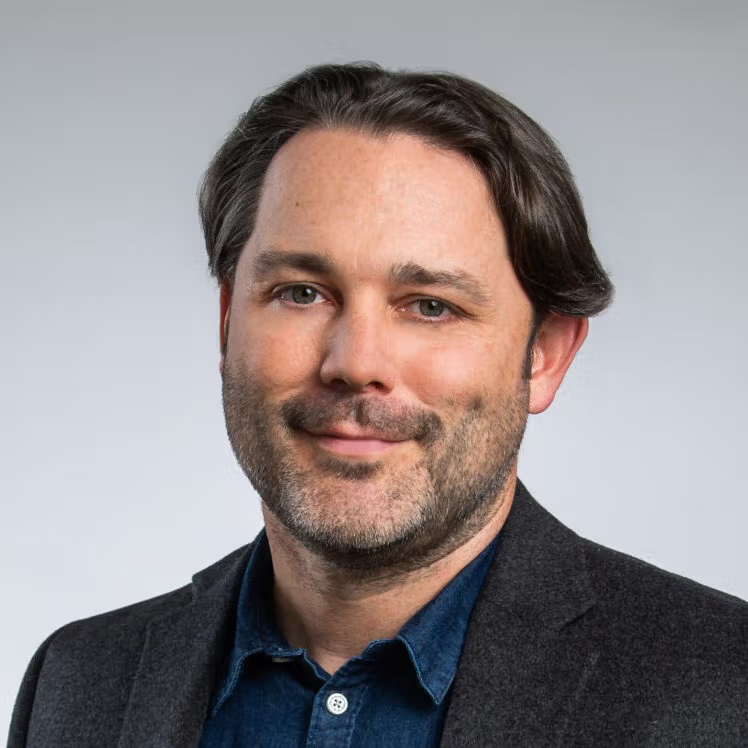