‘AI for Good’ Partnerships Can Make AI Better
Ruchika Joshi / Sep 21, 2024Social impact organizations have the specialized expertise needed to make AI models safer; AI for Good partnerships can do more to recognize and reward that expertise, writes Ruchika Joshi.
At this week’s UN Summit of the Future, world leaders from 193 countries are likely to sign a historic ‘Pact for the Future,’ which includes commitments to realize AI’s potential for enabling sustainable development goals. Major tech companies are expected to leverage this event to advance their partnerships with social impact organizations — especially those in the majority world — under their ‘AI for Good’ initiatives.
But the nature of these collaborations demands closer interrogation. AI for Good partnerships are often narrowly scoped as developers making this technology more affordable to social actors or funding global development projects that use AI. Their potential for impact is conflated with the growing number of proposed use cases made possible by a unilateral transfer of technology from AI developers to social organizations.
Such a one-way model of collaboration appears to be a legacy of previous generations of Tech for Good initiatives, like companies providing affordable cloud services or software for protecting human rights in conflict areas, delivering critical health care services, or increasing agricultural yield.
But AI is different from cloud storage or software. Despite the hype, the technology – as companies suggest in the fine print – is still ‘experimental.’ For the deeply fragile contexts in which social organizations operate, ‘experimental’ often translates to highly risky. This is because use cases in such contexts often relate to the delivery of essential services like healthcare and education for marginalized communities, and regulatory mechanisms to hold AI developers accountable are weak.
A large part of these risks stems from AI's value alignment problem. It is notoriously difficult to formally encode a set of values or instructions into AI systems in a way that guarantees they operate to consistently uphold socially desirable outcomes and human rights.
Part of this problem is technical. AI can fail from goal misspecification, where an AI system incorrectly interprets task definitions (i.e., by focusing on meeting the literal goal rather than the intended human objectives), leading to unintended outcomes. Even when a system’s goal is specified and interpreted correctly, AI can fail when generalizing those goals to novel situations. For instance, an AI model designed for cattle weight assessment misidentified indigenous Kenyan cow breeds as undernourished due to their naturally lean build. This is because the model, which was trained on larger Western cow breeds, failed to generalize to a new context of deployment, which in turn led to a loss of trust in AI tools among local herders.
Beyond these technical challenges, there is also the normative question of what values to encode in AI and how they should be prioritized since people often disagree about the right thing to do.
Value alignment challenges make deploying AI for development goals in majority world contexts uniquely riskier than previous technologies. AI for Good partnerships need to account for that risk differential.
For one, the idea that “AI is experimental” needs to be more than a get-out-of-jail card for model developers. Rather, this reality should be at the heart of setting up AI for Good partnerships so they are better positioned to address the risks emerging from AI’s experimental nature and codify lessons that can make AI safer for everyone.
Stronger partnerships should involve model developers taking more responsibility for jointly navigating context-specific AI risks alongside the social impact organizations who are deploying their technology. This starts with developers offering tailored technical guidance that can help social organizations better understand and assess AI risks specific to majority world contexts. Model developers could provide detailed deployment case studies, practical “how-to guides” for responsible AI use, custom risk assessment frameworks, and support to help deployers reduce risks that are identified. These resources need to address common challenges that arise in majority world contexts, such as data scarcity, cultural and linguistic sensitivities, and limited technical infrastructure.
An entire commercial industry has emerged to customize general AI models into specific real-world enterprise applications, suggesting that specialized technical expertise is necessary to safely deploy the technology. While under-resourced social organizations deploying AI for low-profit use cases have ample contextual expertise, they lack access to the expensive technical know-how required to operationalize that knowledge — a gap developers must help bridge.
Beyond offering customization support, model developers could collaborate with social organizations to define appropriate "risk thresholds" for responsible AI deployment in fragile contexts. Partnerships need to go beyond a one-time transfer of free credits for API use to instead enable social organizations to continuously test models, conduct red-teaming exercises, and provide feedback back to developers that they can incorporate into their models and products. Formal channels of feedback can also help make clear the powerful contributions that social impact organizations make to responsible model development and safety.
These recommendations to AI developers are not merely a call to “do more good.” They offer real business advantages for AI companies expanding into majority world contexts. By building partnerships that enable a two-way exchange on high-stakes, widely dispersed poverty alleviation use cases, AI developers can uncover insights applicable to enterprise use cases, particularly those in resource-constrained or scalable environments.
Such partnerships can also broaden the model risk surface for AI developers to address, uncovering valuable insights on product localization, regulatory compliance, and ethical considerations pertinent to majority world markets. Finally, emerging research suggests that aligning AI models to reduce local harms can, in fact, help mitigate global harms to improve overall model performance.
As the Pact for the Future spurs more AI for Good partnerships, social impact organizations must assert the tremendous value they hold to co-develop the terms of these partnerships such that AI developers take more responsibility for mitigating AI risks. Currently, they are one of the few stakeholders who have specialized expertise in deploying AI in high-stakes, low-profit contexts of the majority world. This body of knowledge, painstakingly built and codified over decades of serving their communities, can make AI better and safer for all deployment contexts. AI developers would do well to recognize, embrace, and reward this expertise in how they partner with social impact organizations.
Related reading
Authors
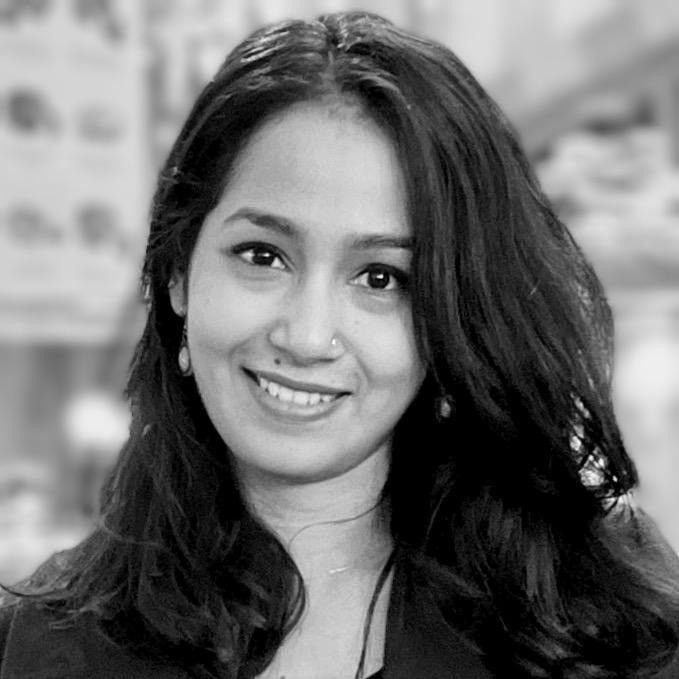