AI Doesn’t Need More Energy — It Needs Less Concentration of Power
Sasha Luccioni / May 16, 2025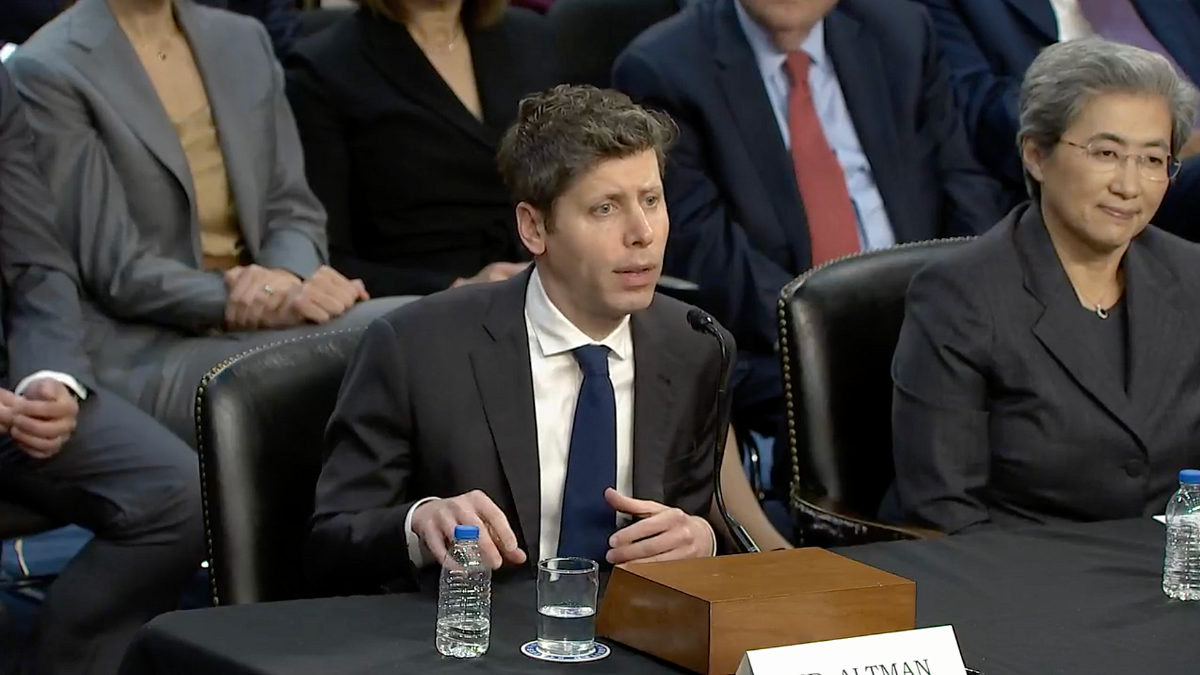
May 8, 2025—OpenAI cofounder and CEO Sam Altman testifies at a United States Senate Committee on Commerce, Science, and Transportation hearing titled “Winning the AI Race: Strengthening US Capabilities in Computing and Innovation.”
At a Senate hearing last week, OpenAI CEO Sam Altman said that "the cost of AI will converge to the cost of energy... the abundance of it will be limited by the abundance of energy." This is in line with much of the Silicon Valley vision of developing AGI (Artificial General Intelligence), or AI systems that will be able to do any task thrown at them, on par with humans. The dominant paradigm that has been adopted to pursue this is ‘bigger models, bigger datasets, more compute’… and an ever-increasing amount of energy.
If we take for granted that this paradigm is the best way to achieve this Holy Grail of techbros, then Altman’s statement makes a lot of sense. But I think both the goal of AGI and how it is currently being pursued are far from the only approaches that exist, and that we should pause and reflect upon the inevitability of this statement before throwing more coal power plants, gas turbines, or nuclear reactors at the supposed problem.
Bigger isn’t always better
The AI community’s obsession with size can largely be attributed to the 2019 blog post by Rich Sutton, in which he insists on the importance of increased computation and scale to improve the accuracy of AI models. This idea got adopted by the machine learning community as a philosophy –that when larger models are trained on more data with more compute, their performance improves. This performance comes with a cost – now the training of a single AI model can cost hundreds of millions of dollars in cloud compute (see Table below) – and use thousands of MWh of energy.
Model name | Number of parameters | Energy consumption | CO₂eq emissions |
---|---|---|---|
GPT-3 | 175B | 1,287 MWh | 502 tons |
Gopher | 280B | 1,066 MWh | 352 tons |
OPT | 175B | 324 MWh | 70 tons |
BLOOM | 176B | 433 MWh | 25 tons |
Source: Luccioni et al. (BLOOM paper)
But this brute force approach to model training isn’t the only one available to us. There are alternative ones, including smaller models (like the smolLM family) that achieve the same performance with a fraction of the compute. There are also techniques like distillation and quantization that reduce the size of models so that they can run locally on a laptop or even a phone, which not only reduces energy and emissions but also helps in terms of privacy and data protection.
Bigger is also more concentrated
Taking a step back and thinking about Altman’s testimony, it becomes clear that this reinforces the existing power dynamic in AI, in which big tech companies with access to massive supercomputers are put at an advantage, shaping the narrative. In recent years, since the launch of ChatGPT, this has been the case – AI models have become both larger in size and increasingly concentrated in a handful of organizations that can afford to train them. This also makes AI researchers dependent on grants and funding from these same organizations, which results in increased industry influence on academic integrity and freedom in the field. And as the same few companies both sponsor and shape AI research, provide the compute required to carry it out, and sell users tools and ads based on this research, the field as a whole becomes an echo chamber for the interests of Big Tech, which has consequences for society as a whole.
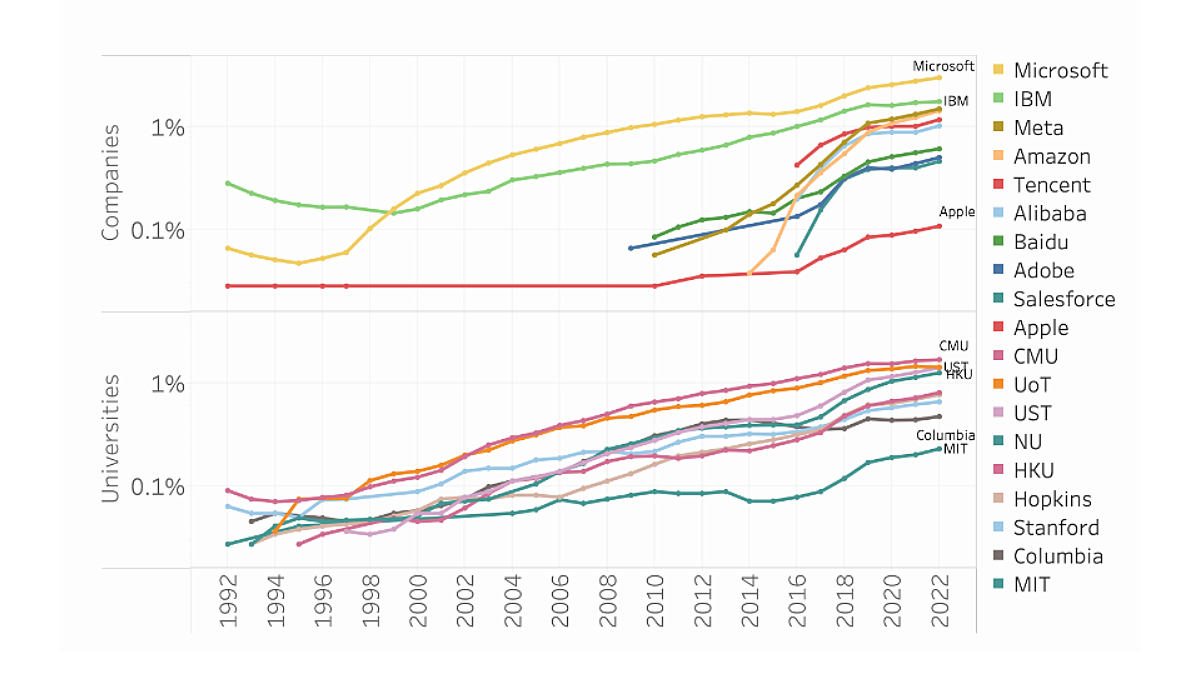
Source: Abdalla et al (2023)
For instance, nuclear has become the main source of energy that will power the AI boom – as can be seen by Microsoft’s deal to restart Three Mile Island, Altman’s investments in Helion, a startup that’s working to develop and commercialize nuclear fusion, or Google’s agreement with Kalios to purchase nuclear energy from small modular reactors (SMRs) to power Google’s datacenters. This means that in the near future, we will see what happens when the (rightfully) slow-moving nuclear energy sector meets Silicon Valley’s “move fast and break things” mentality. I’m worried that corners will be cut in the never-ending quest to generate more energy to fuel the AI’s quest for scale and compute.
But if we push back against this narrative – by exploring alternative ways to train and deploy AI models, by requiring AI companies to measure and disclose their energy use and emissions more rigorously, and by holding them accountable if (or when) things go wrong – we can still steer AI in a different direction. As Dr. Ruha Benjamin so eloquently puts it, “Whatever happens in the future, whether it's loathsome or loving, is going to be a reflection of who we are and what we make it to be.”
So instead of believing that the future of AI is already written and that it will require infinite amounts of energy, I think that we can collectively imagine and shape an alternative one, where AI models are smaller and more efficient, used in more frugal ways, and developed by a large number of people and organizations, not only by a handful of tech companies.
Authors
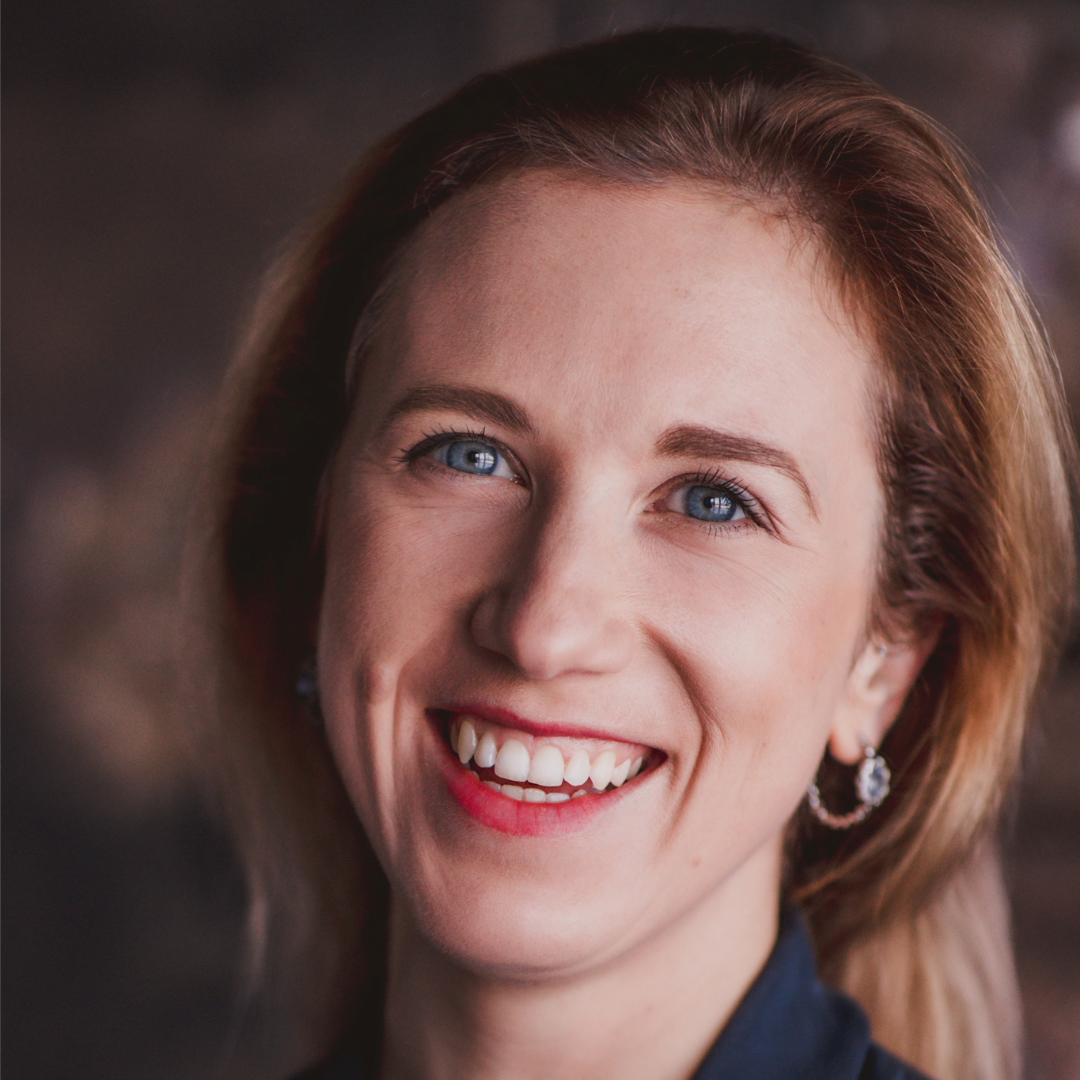