AI Companies Threaten Independent Social Media Research
Ryan McGrady, Ethan Zuckerman, Kevin Zheng / Jan 30, 2025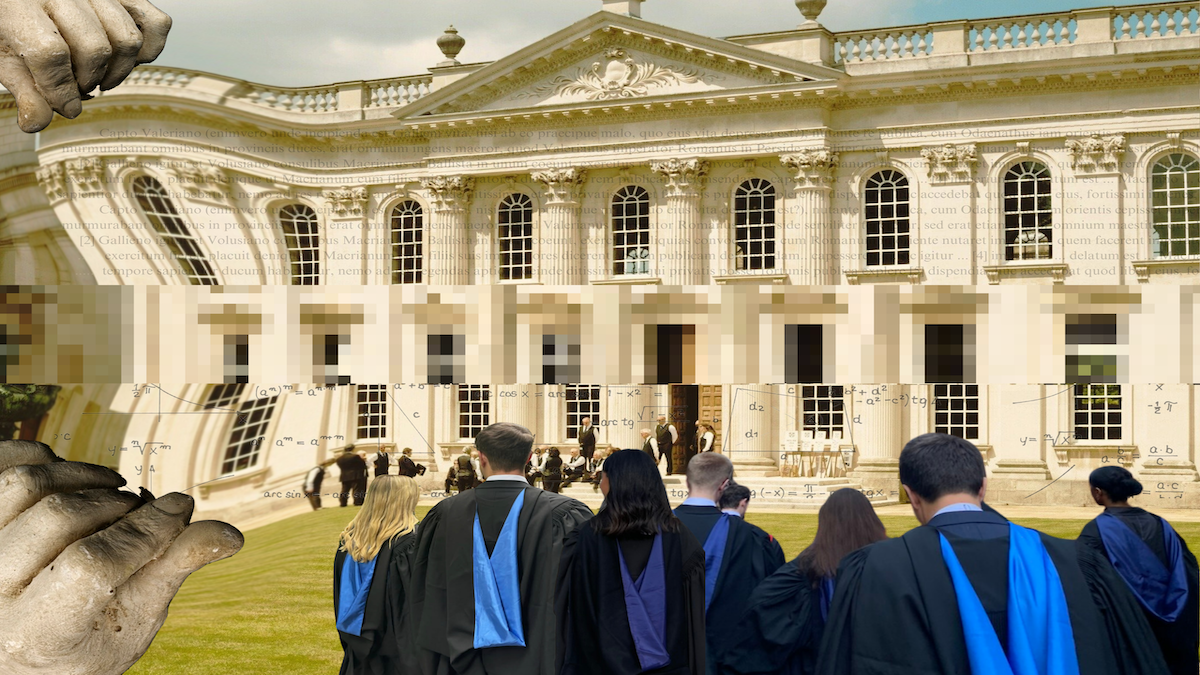
Zoya Yasmine / Better Images of AI / The Two Cultures / CC-BY 4.0
The machine learning models that produce text, images, and video based on written prompts require massive amounts of data. Popular models like ChatGPT may have started small, with public domain ebooks, Congressional transcripts, and freely licensed content like Wikipedia, but the competitive demand for more and more data to build better and better models has led these companies to look elsewhere. Their bold decisions to ingest large quantities of copyrighted books, news, photos, and other content, implicitly arguing that transforming media into an AI model is a form of fair use, has triggered many ongoing lawsuits. Given a tech culture that values speed over permission, the next inevitable tranche of media to ingest was social media—a vast treasure trove of the world's user-generated content.
From the perspective of a social media user, having one's data used to train a powerful model is troubling. In theory, because you own the copyright for any original content you post or upload, users of your content should seek your permission to use it and perhaps license it from you. In 2019, when IBM used Creative Commons-licensed photos from the photo-sharing site Flickr to train a facial recognition algorithm, there was an outcry from users and press scrutiny. Uploaders that had made their photos available for reuse but hadn’t anticipated that reuse would mean “use as training data.” This shift in usage is something philosopher Helen Nissenbaum calls a violation of “contextual integrity”—IBM took data shared in one context and used it in a different one, counter to users’ expectations.
In addition to issues of ownership and permission, using user-generated data to train AI systems raises serious privacy concerns. While it's easy to get the impression that YouTube is full of professional content creators and legacy media institutions like MrBeast, T-Series, or CNN, there are far more amateur, banal use cases—family birthdays, homework assignments, condo board meetings, and kids lipsyncing for their friends. These kinds of videos aren't meant for a wide audience, but they may find themselves used to train ubiquitous text and video models.
Companies that own social media platforms, like Google, Meta, and Reddit, don't like having content they host used without compensation or permission, either. They may have a sense of responsibility or obligation to protect their users' intellectual property and personal data, and they probably don't like subsidizing AI training by paying the bandwidth bills to serve AI companies millions of videos. What they want is to stop other companies from profiting from their data (or their users' data) without asking and without paying. So, it isn't surprising that many have begun employing a range of legal, procedural, and technical interventions. On the legal front, dozens of companies (including large and small media outlets and newspapers), groups, and individuals have sued AI companies, usually on intellectual property grounds. Procedurally, several platforms and publications like The New York Times have modified their terms of service to explicitly disallow the use of its content to develop software, including AI.
One of the most common technical interventions has been to modify sites' robots.txt files to disallow AI companies' crawlers and scrapers. Robots.txt is a standard from the early web through which site owners can tell a web crawler what parts of the site it can and cannot access. (Web crawlers index the internet by following links and retrieving content—they provide the information search engines need to point to useful content online.) In the spirit of the early web, it is an entirely voluntary system, punishable only through reputational damage and social sanctioning. Indeed Reuters reported that some AI companies may simply be ignoring requests to leave content unindexed. Other newer technical measures may be more effective in blocking AI companies but create collateral damage by making life difficult for social science researchers.
Permissioned and Un-permissioned Research
There are typically two broad categories of methods to get research data from a platform like YouTube: permissioned and un-permissioned. Permissioned access usually involves applying for an API key, enabling you to connect directly to the back end to retrieve the needed data by querying its database. In gaining permission, however, a researcher agrees to a set of rules. These rules can be technical, like a quota limiting the number of times you can request data each day, a requirement to delete raw data after a certain number of days, and adherence to certain data handling or privacy standards, but the rules can also take the form of research obligations (getting approval for each project, agreeing not to use data for any other projects, submitting research to the platform before publication, etc.). These kinds of requirements restrict the types of research that social scientists can conduct with permissioned data, and not all data they aim to study is accessible through permissioned methods.
Another drawback to the permissioned approach is that it's not always clear what you're getting when you access the site through the official API. For example, TikTok research staff disclosed to us that the TikTok Research API grants access not to all of TikTok but to a subset of TikTok data that its research team analyzes. It helps to explain why existing research conducted using its API exhibits some serious red flags, like a strange finding that well over half of all TikTok videos were uploaded on Saturdays. We do not believe this finding is accurate, and such a result suggests that the TikTok Research API does not represent the whole of TikTok. We would not have known why if we hadn’t directly approached members of the TikTok research team. Robust APIs facilitate high-quality research, which, in turn, enables oversight and examination of the companies that own large parts of our public sphere.
Unpermissioned access covers everything else, including a wide range of web scraping methods and tools. Companies typically rely on this approach to collect training data from platforms that are not keen to share freely, prompting many platforms to take steps to shut down such efforts. One popular, powerful tool to scrape YouTube is called yt-dlp. If you have a list of video IDs you want to study, you can use yt-dlp to download the metadata, audio, and/or video. It's immensely useful to study health communication, hate speech, linguistics, popular culture trends, political bias, and just about anything else up to and including YouTube as a whole. NVIDIA used it to scrape YouTube content for its visual models, and, as it happens, it's also a tool that our team at UMass Amherst uses for our research.
The Scraping Crackdown
We conduct research projects based on random sampling—often the only way to produce reliable estimates about a population (or an entire platform). Through random sampling, we can uncover basic information about YouTube (and TikTok) that the companies do not want to share but that the public deserves to know. For example, we’ve determined the number of videos on YouTube (about 15 billion), how much it's grown over time (it's nearly tripled in size since 2020), how many videos are categorized as "News & Politics" (2.62%), and why those categories have limited usefulness (most people just use the default category).
Random sampling isn't typically compatible with permissioned research. Even the platforms with good APIs usually don't provide a mechanism to produce a reliable random sample, so we have to use methods that involve a lot of guesswork. For this reason, and because we want to avoid seeking Google's approval for our projects, we choose the un-permissioned approach.
However, there is a risk to choosing this approach. Some months ago, YouTube blocked the cluster of computers at UMass Amherst that we use for our research. It didn't come as a complete surprise. Over the summer, shortly after news broke of OpenAI's scraping of YouTube, we met a Google insider at a conference who warned us it might happen. After we presented our findings gleaned from scraping YouTube, they explained the company would likely be cracking down on scraping soon due to the actions of AI companies. Since then, we've seen other yt-dlp users complain about similar blocks, and we've had several conversations with other researchers and developers of tools that aid researchers experiencing similar problems. A GitHub search for the error message yt-dlp users receive when it is blocked returned hundreds of hits from this year alone.
Fewer Tools to Understand Our Digital Infrastructure
The loss of a key research tool is part of a larger phenomenon. Over the last two years, internet research has faced a set of setbacks that reduce our options to study civic issues like hate speech, censorship, misinformation, content moderation practices, and social media’s impacts on young people. This trend started with Elon Musk buying Twitter, effectively shutting down its API for research purposes, and even suing researchers trying to study the site. Then Reddit clamped down on its API, leading to widespread (and frequently entertaining) but ultimately ineffective protests. Executives pointed to AI explicitly in their communications about the move, and the company later revealed it entered into a licensing agreement with Google. Then, Facebook shut down CrowdTangle, the primary way researchers studied Meta platforms (the unofficial funeral was in September).
In other words, we have lost many of the key tools necessary for permissioned research, and AI companies' scraping is jeopardizing un-permissioned projects. Independent technology research is crucial for ensuring a degree of transparency and understanding the platforms that are enmeshed in so much of our social, economic, entertainment, educational, and political lives. Without it, what we know about Meta, Google, or X will be limited to what they choose to disclose—which is likely to be the bare minimum they are required to share.
A Bipartisan Issue
It’s possible that some critical research about platforms will move to the EU. The Digital Services Act (DSA) is a broad package of regulations designed to increase the transparency and responsibility of large internet platforms. It includes language (Article 40) which seeks to provide researchers with the data they need to hold large platforms accountable for systemic risks. It is all but inevitable that researchers, regulators, and the large platforms covered by the law will disagree on what constitutes systemic risk and what data must be released to evaluate those risks. But at least a mechanism exists, even if it likely involves judicial rulings and applies only to the largest US-based platforms. In contrast, the US lacks similar regulatory protections, and existing APIs are too limited to allow critical research on the “systemic risks” posed by these platforms.
While we wait to see how much access the DSA gives EU researchers and whether the US will enact similar transparency legislation, we continue to find creative ways to conduct our research. We don’t know how long our new methods will remain effective, especially as many of our colleagues have had to completely halt their research projects due to hostility from X and Reddit. While we believe that careful review of technology platforms should be a bipartisan issue, the alliance between President Donald Trump and Elon Musk—who’s shown perhaps the most contempt of any technology executive for independent research—is a discouraging sign.
If you think big tech companies are too powerful but aren't sure what regulations would be most effective, independent technology research ensures you have the data you need to make such decisions. If you're worried about the influence of platforms owned by foreign adversaries, the information we have about those platforms shouldn't be limited to what they choose to share about themselves. If you're concerned about the spread of misinformation online or suspicious of certain platforms' content moderation decisions and their impact on speech, independent research can provide you with the evidence to back up your argument.
Similarly, if you think a platform has a left-wing or a right-wing bias, you probably don't want the algorithms of tomorrow opaquely trained on that biased content. If you think ChatGPT is trained to be “woke," you should support greater transparency in its training data. If you see social media as potentially harmful to young people's mental health, preserve the few ways we have to study that phenomenon without results going through social media companies first. Researcher access to social media data should be one of the rare things all political factions in the US can agree on.
A Problem of Will
Resolving issues with the ownership and transparency of training data wouldn't resolve all of the challenges researchers face, but it would relieve some of the pressure we're under. One potential solution is for one of the ongoing intellectual property lawsuits to end with a careful ruling about the limits of fair use. Such a ruling could place major restrictions on scraping, but it could also push AI companies into paying licensing fees to use the same data. If licensing becomes common, it could reduce platforms' paranoia about other, more legally justifiable forms of scraping.
Another approach is to legislate transparency in the kinds of training data the companies use. California recently moved in this direction with AB2013, which requires companies like OpenAI to publish information about the training data it uses. The EU AI Act, which entered into force in August 2024, demands transparency from developers of “high-risk AI systems” as to data used to train those systems. (As always with EU law, the forthcoming debates over what constitutes a “high-risk system” and what transparency is required will be important to watch.) It's unclear how far-reaching European and California laws will be and what legal challenges the laws will face. Still, the overwhelming bipartisan success of such legislation indicates broad public interest in creating more checks. A comprehensive transparency bill like the Platform Accountability and Transparency Act offers both permissioned and un-permissioned paths toward research data, recognizing that we may need multiple ways to study complex phenomena.
The problem is not a lack of solutions. From platform APIs that provide random samples to protections for un-permissioned research in the public interest, we can imagine ways to protect the right to research these platforms that are reshaping our public sphere. Our main problem is political will. Congress will ban TikTok without publicly disclosing evidence that the Chinese government is manipulating its algorithm. Laws will seek to restrict teens' access to social media with inconclusive evidence that its harms outweigh its benefits. But thus far, political leaders and policymakers have largely been unwilling to protect our rights to answer fundamental questions about how social media is shaping our public discourse or affects individuals and society. If we want responsible policy about social media, it begins with making it possible for American researchers to find answers to these critical questions.
Authors
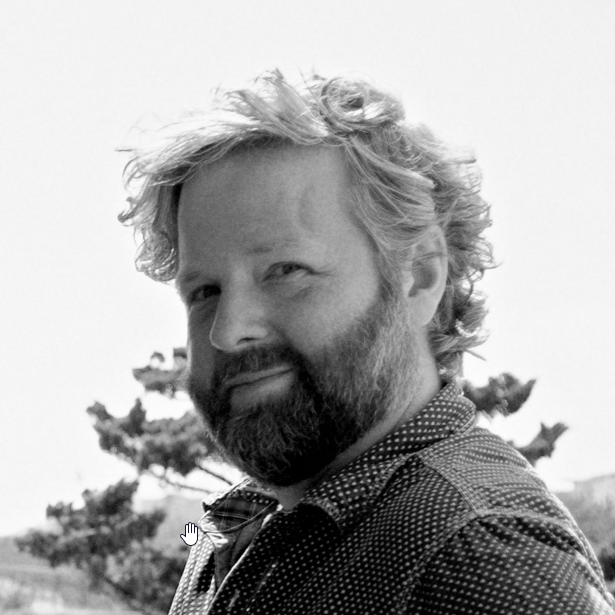
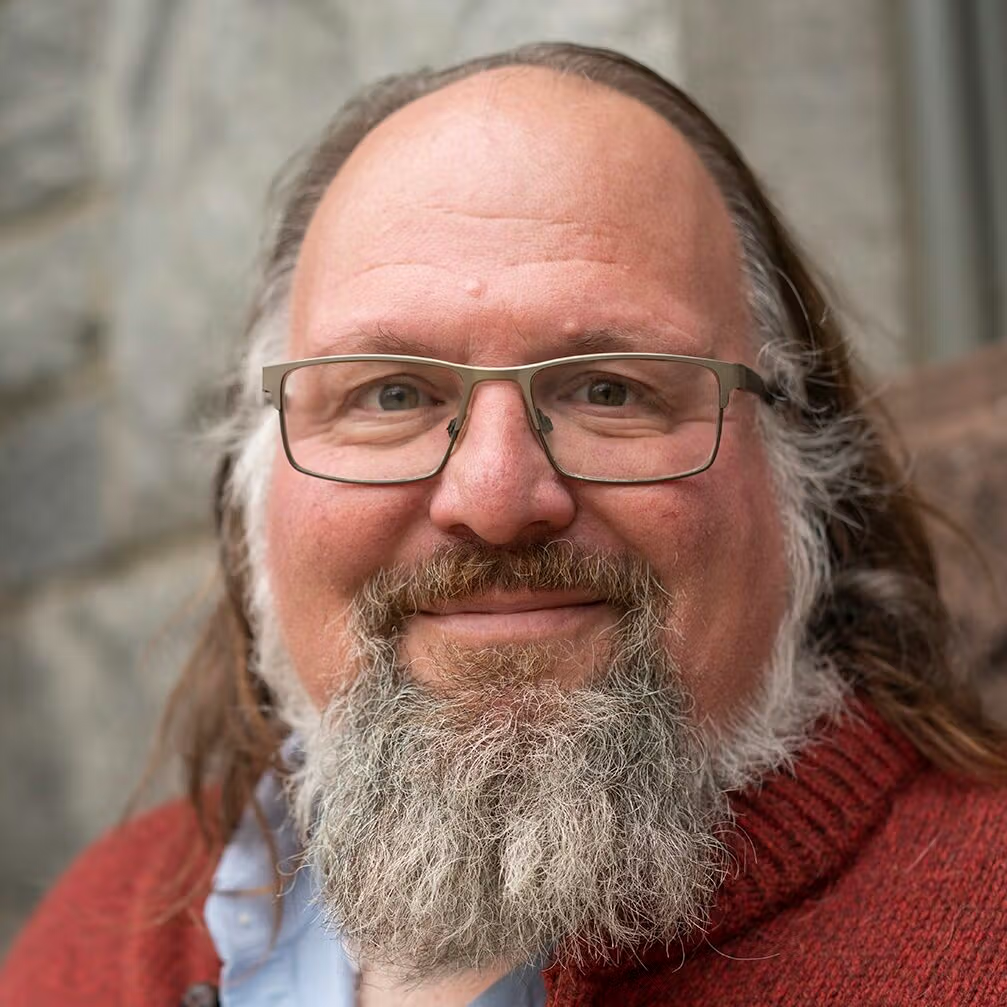
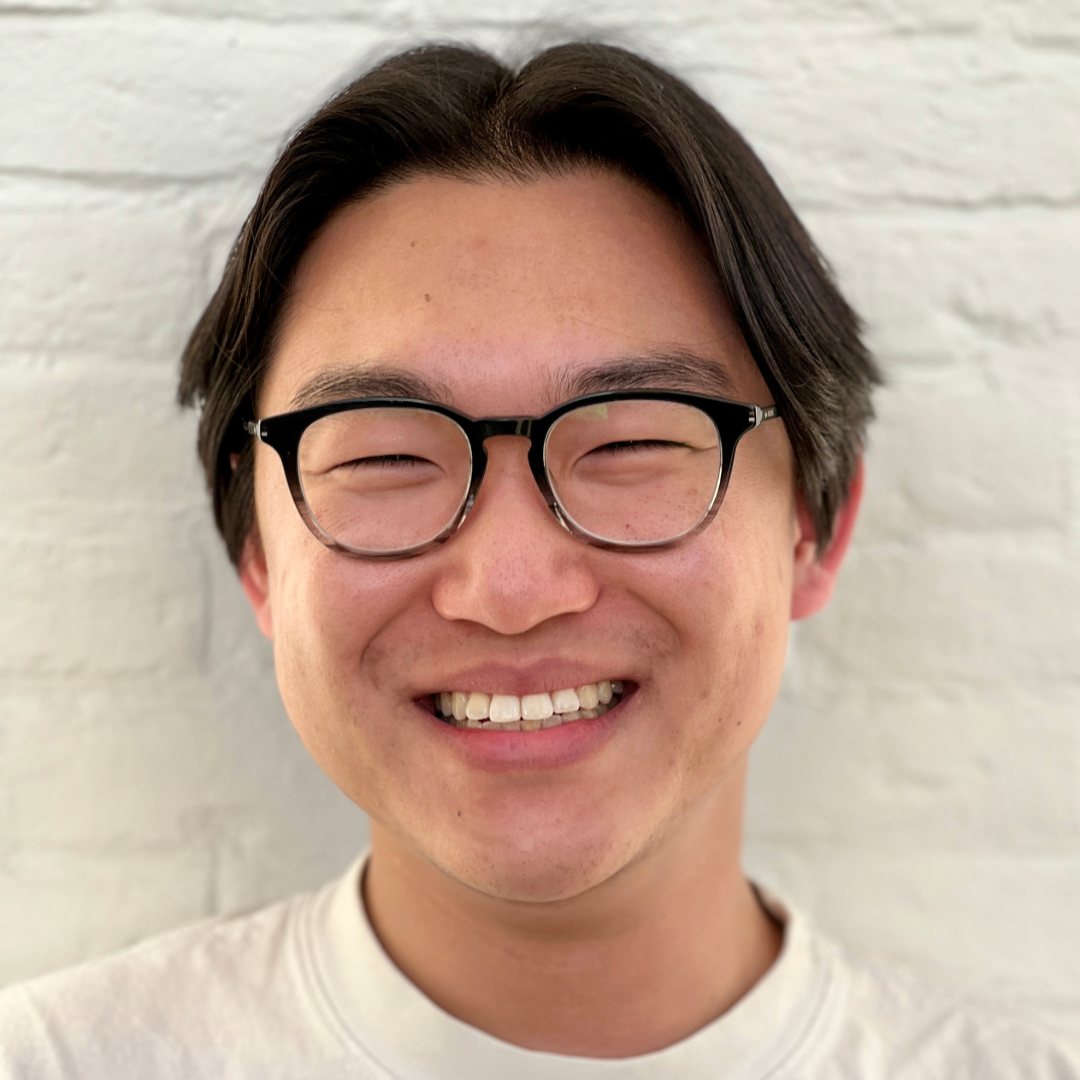