Accelerating the Evolution of AI Export Controls
Ritwik Gupta, Andrew W. Reddie / Sep 21, 2023Ritwik Gupta is a computer vision Ph.D. student at the University of California, Berkeley, in the Berkeley AI Research Lab and a fellow at the Berkeley Risk and Security Lab and Berkeley Center for Security in Politics. He serves as the Deputy Technical Director for Autonomy at the Defense Innovation Unit. Andrew W. Reddie is an Associate Research Professor at the University of California, Berkeley’s Goldman School of Public Policy, and Founder and Faculty Director of the Berkeley Risk and Security Lab.
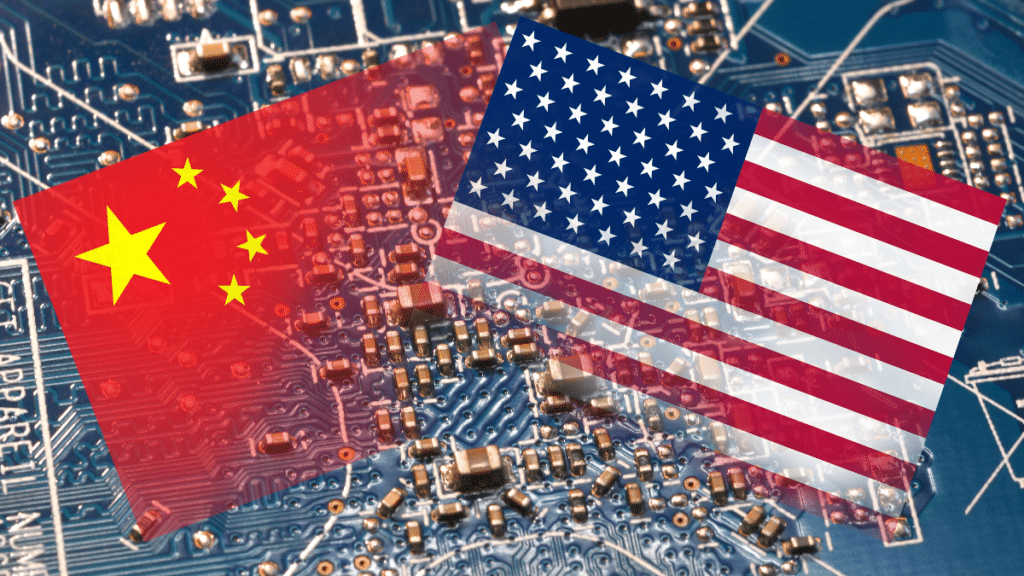
Artificial intelligence is poised to revolutionize economies, scientific research, and the power dynamics between governments and industries. As AI research expands and its capabilities grow, a nation's prowess in AI will increasingly signify its overall strength.
The United States faces a competitive geopolitical environment and must make strategic investments, regulations, and restrictions to maintain its leadership in AI R&D while countering the gains of rivals like China. The US has held a slim lead in the development of AI models according to Stanford’s 2023 AI Index report, and a large lead in the development of hardware needed to train AI models quickly and effectively. Ensuring that adversaries are unable to access the hardware “engines”' that drive AI model development is a strategy by which US policymakers believe the US can create a “moat” that provides the it with an impassable advantage. The nation's recent imposition of export controls on advanced semiconductors seeks to further limit China's access to the cutting-edge chips needed to build supercomputers and train advanced AI systems.
While broad performance thresholds capture makers of high-end GPUs and mainstream chips, gaps exist where powerful AI accelerators that contribute important AI workloads can still be acquired by China. Closing this loophole requires deep analyses of machine learning workloads, China’s evolving AI needs and partnerships, and attempts to source advanced deep learning accelerator (DLA) technologies from industry and academia.
In the following, we explain the export control performance thresholds introduced in 2022 by the CHIPS and Science Act, detail how common, powerful machine learning models work in terms of computation, and analyze how the use of DLAs might enable adversaries to make effective use of these models within export control regulations.
Understanding US Export Controls
Export controls are often viewed as something of a panacea among policymakers seeking to address the fact that domestic industry remains at the leading edge of innovation even while strategic competitors continue to seek access to this same innovation—using both licit and illicit means. The US semiconductor industry holds the majority of the global market share for advanced integrated circuits such as CPUs, GPUs, and more. Chips such as GPUs underpin the global AI market—the ability to create and use AI models is greatly diminished without the use of such chips.
As opposed to AI models which circulate openly on the Internet under permissive licenses, advanced integrated circuits have become the focus for export control. The expertise and manufacturing prowess required to produce such circuits are extremely limited and provide an asymmetric advantage over other nations that also hope to field AI capabilities.
Export Controls
In October 2022, the US Government instructed the Bureau of Industry and Security to place export controls on advanced semiconductors and processors that can be used by China to build supercomputers. These export controls manifested in restrictions and new licensing requirements on advanced computing integrated circuits (export control classification number (ECCN) 3A090) and computers and electronic assemblies containing those integrated circuits (ECCN 4A090). Additionally, the interim final rule controls the export of software and technologies used to manufacture items under ECCNs 3A090 and 4A090 and places end-use controls on any of the above items if the exporter knows that they will be used to build a “supercomputer”.
To cover the broad industry sector and not just companies such as NVIDIA or AMD, the interim final rule provides performance thresholds to assess chips. Any integrated circuit that surpasses these thresholds is subject to export controls.
Integrated circuits are controlled under the new ECCN 3A090 if:
- Aggregate bidirectional transfer rates are 600 Gbyte/s or more, or
- Digital processors can execute >= 4,800 TOPS (trillion operations per second), or
- Digital primitive computational units can execute >= 4,800 TOPS, or
- Analog/multi-level computational units can execute >= 38,400 TOPS
ECCN 4A090 is easier to understand: computers are controlled under this licensing regime if they contain integrated circuits exceeding the performance thresholds specified under ECCN 3A090 above.
These performance thresholds match almost exactly the performance envelope of NVIDIA’s A100 chip. The A100 is one of the highest-demand GPUs in the world today. 40GB A100s cost more than $6,000 each, and 80GB A100s cost more than $15,000. Academics, startups, and incumbent cloud providers alike are attempting to acquire these GPUs. Meta, for example, is creating a supercomputer with 16,000 A100s for internal usage only, and public-facing supercomputers like the European Union’s Leonardo and the US’ Perlmutter have ~16,000 and ~7,000 A100s, respectively.
With these performance thresholds, any company that builds comparable integrated circuits is bound to the export controls. To still compete in the Chinese market, companies such as NVIDIA and Intel are releasing “nerfed” versions of their flagship chips for sale in the Chinese market. NVIDIA’s A800 series of chips are a fraction of the power of their A100 offerings, and even Intel released a scaled-down version of their Gaudi 2 accelerator to comply with US export controls.
In the interim, companies such as NVIDIA continue innovating and releasing new GPUs. NVIDIA’s latest iteration of GPUs, the H100, offers more than 900 Gbytes/s of bidirectional transfer rates and can run upwards of $30,000 in its 80GB configuration. Major cloud providers and high-performance computing centers have already placed orders for these chips in droves, a difficult prospect in the current era of chip shortages.
Deep Learning Accelerators
Graphics processing units (GPUs) including those produced by NVIDIA, AMD, and Intel were created out of necessity. Central processing units (CPUs) were multiple instruction, single data architectures and were not able to perform complicated operations on matrices efficiently. With the advent of graphical user interfaces (GUIs) and applications that made heavy use of GUIs, co-processors that could do efficient computations relevant to graphics, i.e. linear algebra, were needed. GPUs filled that gap.
Later, as machine learning further developed, GPUs filled a general role for anything related to linear algebra. As such, GPUs found a new niche in the world of machine learning. However, the number of operations that are used on the GPU for machine learning is a fraction of all the possible operations a GPU could perform. Therefore, a lot of the power, latency, and complexity is “wasted” when using a GPU for machine learning tasks. This is the value proposition behind a new wave of companies that aim to build a new class of co-processors: deep learning accelerators.
Deep learning accelerators (DLAs) are application-specific integrated circuits (ASICs) that only perform a few operations relevant to modern machine learning workflows. By limiting their scope, they are able to achieve superior performance, power consumption, and thermal output compared to GPUs for specific use cases. Industry frontrunners such as Google, Tesla, and Meta all build DLAs for their machine learning workloads, and a new wave of startups such as Cerebras, SambaNova, and Graphcore also have mature DLA offerings.
The export control as enacted by the CHIPS Act covers deep learning accelerators (and any co-processor, generally) within its language as bound by the performance thresholds set for ECCNs 3A090 and 4A090.
China’s Machine Learning Workloads
Much like its counterparts across the world, China has been a heavy adopter of GPU technologies and supporting software to accelerate its machine learning workloads. Chinese cloud providers have been building their offerings on NVIDIA and Intel GPUs which are then leveraged by their industry and government partners alike.
The Chinese DLA/ASIC market has lagged the American market by a large margin, potentially due to a lack of demand in the domestic market. There are almost no Chinese DLA companies with offerings that match those of the American market. Therefore, the Chinese machine learning environment is almost entirely dependent on GPUs for its hardware acceleration needs.
It is highly likely that China will attempt to adopt DLAs in greater quantities as its supply of American GPUs dries up. The process node required for DLAs is higher than that of GPUs, something that China can manage without access to Taiwan. However, hardware expertise required to design and manufacture DLAs must be recruited and retained for an extended period of time. Additionally, heavy investments in software toolkits that make these chips usable and performant will need to be made. Even in the best of environments, such as at the American company AMD, this is a tough prospect—AMD’s GPU software stack pales in comparison to NVIDIA’s and therefore its GPUs have drastically lower market share. In sum, it is difficult to imagine that Chinese investments in advanced AI chips will pay off on any timescale shorter than a decade.
So Why an AI Hardware Export Control?
The implementation of AI hardware export controls serves as a strategic response to concerns regarding China's expansive dissemination of AI technologies. The US has consistently utilized its dominant market position to enforce a series of export controls, and an AI hardware export control represents a bold and comprehensive step in the ongoing AI competition between the US and China. It is evident that China possesses a wealth of talent and resources to construct advanced machine learning workflows, akin to having abundant high-performance fuel at its disposal.
Nonetheless, by withholding high-performance engines from China that can maximize the utilization of this fuel, the US erects a formidable “moat” of capabilities that presents a challenging obstacle to breach, particularly in the near term.
The imperative to innovate often arises from necessity. While China's burgeoning GPU and DLA market may regain momentum as a new generation is educated in semiconductor fundamentals and entrepreneurs embark on new ventures, the “moat” may persist unless Chinese DLA advancements surpass the pace of American innovation.
The Evolution of Machine Learning Models Necessitates the Evolution of Export Controls
Machine learning models and their underlying software frameworks do not develop in isolation. Researchers and engineers craft software that inherently relies on the capabilities of their hardware infrastructure. In the machine learning community, there's an ongoing oscillation between leveraging existing hardware resources and exploring innovative architectures that harness new hardware functionalities.
As the landscape of machine learning undergoes transformation, it becomes imperative for export controls and their associated performance metrics and thresholds to evolve in order to sustain a competitive edge. These analyses aid in understanding the dependencies on GPUs versus DLAs and the ecosystems surrounding them.
Over the past decade, convolutional operations have dominated the machine learning sphere. Convolutional Neural Networks (CNNs) introduced spatially invariant deep learning, catalyzing advancements in areas like visual recognition, object detection, and even audio analysis. The success and widespread adoption of CNNs prompted the GPU market to incorporate hardware enhancements for GPU acceleration, culminating in the emergence of several DLA companies primarily focused on expediting CNN training and inference.
However, with the adoption and extension of the groundbreaking “Attention is All You Need” paper, the field underwent a rapid transition towards attention-based architectures, such as transformers. Transformers have revolutionized language comprehension, video-language integration, and tasks like image classification. Figure 1 presents a brief analysis of the frequency of references to "transformer" versus "CNN" in research publications, utilizing data from Semantic Scholar. As the hardware industry adapted to support CNNs, it similarly evolved to meet the computational demands of transformers.
While transformers have exhibited superior performance across various crucial tasks, CNNs continue to maintain their popularity in practical applications such as object detection and scene analysis, where transformers have yet to establish a dominant foothold in performance and user-friendly toolkits.
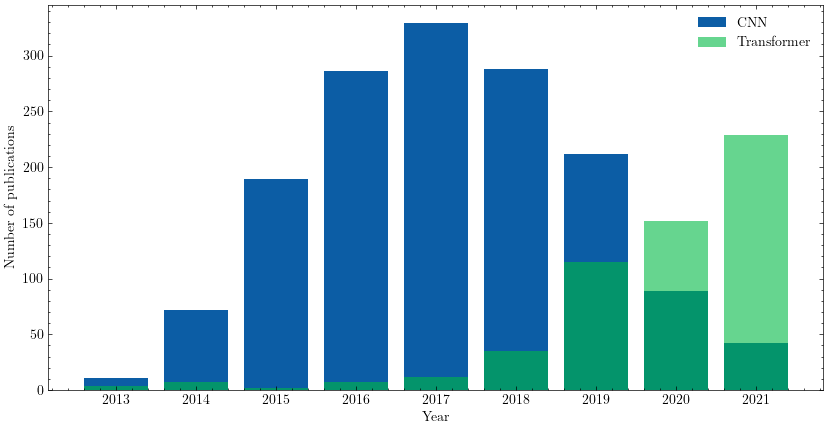
The computational foundations of transformers involve minimal FLOPs relative to the amount of data they access, resulting in low arithmetic intensity and a significant reliance on memory bandwidth. Large language models (LLMs), which are colossal transformer models, necessitate distribution across multiple chips for training and, in some cases, for inference. Consequently, to facilitate the efficient acceleration of transformers, the AI hardware market has evolved to improve intra-chip bidirectional data transfer rates.
On the other hand, CNNs exhibit much higher arithmetic intensities compared to transformers, enabling them to execute many more FLOPs per unit of data accessed from memory. For CNNs, metrics such as bidirectional transfer rates matter less since they can execute swiftly with smaller data volumes. Nevertheless, it's important to note that leading implementations of both transformers and CNNs substantially underutilize existing hardware capabilities.
The US has established performance thresholds for ECCN 3A090 based on the prevailing state of the AI hardware industry. However, the performance metrics of leading industry chips encompass characteristics required by vastly different categories of valuable machine learning architectures. Establishing thresholds based solely on such metrics creates “loopholes” where chips designed to accelerate a subset of these models can still be exported.
DLAs Bypass the Intended Effect of Export Controls
Established export control performance benchmarks have centered on metrics like intra-chip bandwidth. However, a new frontier is emerging as companies like Cerebras develop exceptionally large DLAs capable of accommodating hundreds of thousands of cores on a single silicon wafer, alongside extensive memory resources. When all essential computation and data reside on a single chip, metrics such as bidirectional transfer rates lose their relevance.
For instance, take the Cerebras WSE-2 DLA, which boasts an impressive 123 times the number of cores compared to an NVIDIA A100, even though the individual cores on the WSE-2 reportedly offer a lower FLOP count than the A100 chip. Nevertheless, the WSE-2 shines with its substantial 40GB of high-speed SRAM, in contrast to the A100's 40MB of SRAM (with an additional 40/80GB of DRAM). Most notably, the WSE-2 delivers an extraordinary level of memory bandwidth between its cores, surpassing the A100's capabilities by over 12,700 times.
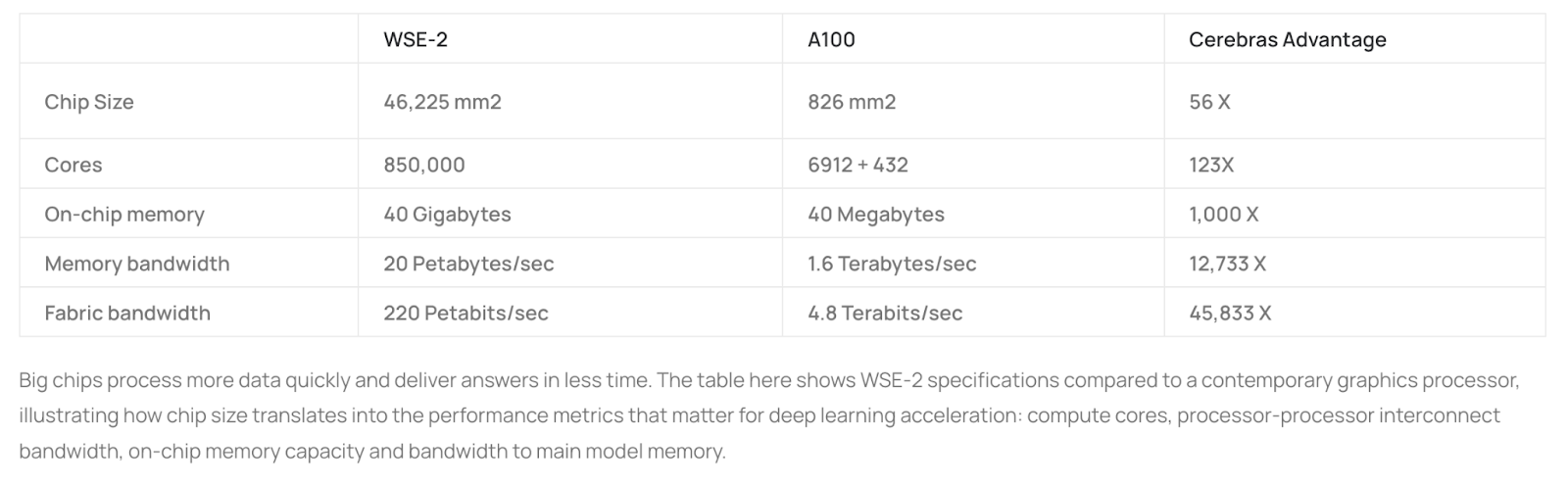
Given these performance metrics, the Cerebras WSE-2 DLA emerges as a formidable contender to an NVIDIA GPU for specific machine learning workloads. This category of workloads predominantly encompasses common types of CNNs, which stand as a prevailing family of machine learning models in use today. In fact, Cerebras’ WSE-2 chip is explicitly designed to amplify CNN performance and, crucially, falls below the performance thresholds delineated in ECCN 3A090, enabling their international export without the need for licenses.
In the ever-evolving landscape of machine learning models, export controls must remain agile to keep pace. The challenge lies in establishing export performance benchmarks that cater to a wide spectrum of machine learning models as hardware capabilities shift between architectures like CNNs and transformers. While transformers have garnered considerable attention, CNNs continue to excel in specific domains. Additionally, the emergence of DLAs such as Cerebras' WSE-2 introduces complexity to export controls, as these sizable DLAs can outperform traditional GPUs for distinct tasks, thus challenging the original regulatory intent. Consequently, U.S. export control policymakers face the intricate task of harmonizing regulations with the diverse and ever-changing requirements of the machine learning community.
Recommendations for US Export Control Bodies
The US Department of Commerce should align with the prevailing trend of software-hardware codesign, a trend in both academia and industry that shapes how AI software and hardware are created. Instead of exclusively centering export control performance thresholds on aggregate chip performance, the Department of Commerce should conduct a granular analysis, targeting specific workloads and architectures deemed potentially detrimental. This approach warrants the establishment of more precise hardware performance metrics and thresholds, casting a finer net for licensing purposes.
One practical strategy involves vigilant monitoring of the outcomes arising from the MLPerf family of benchmarks, designed to assess how reference models perform across diverse software implementations and hardware backends. Both academia and industry actively engage in these benchmarks to showcase their evolving capabilities. Prior iterations of the benchmark have illuminated how Chinese cloud providers harnessed American DLAs to dominate these benchmarks. To address this, the US government can mandate that private industry submits competitive entries to these leaderboards as a prerequisite for obtaining export licenses.
Furthermore, the incorporation of diagnostic frameworks and metrics, such as the Roofline model and model FLOPS utilization, should play a pivotal role in justifying performance thresholds.
Recognizing the swift pace of innovation within the AI ecosystem, characterized by weekly software releases and semiannual hardware unveilings by companies, the Department of Commerce should adapt by refreshing performance threshold figures biennially, while performance metrics should undergo reevaluation every five years. To facilitate these assessments, the Department should convene a panel of experts drawn from academia and industry.
Additionally, the establishment of a standing technical expert committee is advisable to bolster the Bureau of Industry and Security in evaluating the eligibility of companies seeking export licenses. Such a committee would provide invaluable technical insights to inform export control decisions.
Responding to an Evolving AI Landscape
Artificial intelligence technologies are progressing at a rapid pace. Innovation in the software realm emerges daily from industry and academia around the world. Adapting models to computational restrictions is a heavily researched field. It is therefore imperative that the United States’ export controls on AI hardware evolve on a yearly basis in response to advances in AI software.
Deep learning accelerators are a maturing capability that is being widely adopted by US-based cloud service providers such as Amazon and Microsoft. By ensuring that DLAs are well controlled, the US can maintain the net effect of a dilution of adversaries’ AI capabilities by starving them of the engines required to operate these models.
Additionally, the US must proactively engage in outreach to US-based DLA companies to educate them about the national security implications of their work and the importance of due diligence on their partnerships. By adopting both a top-down and bottom-up approach to controlling advanced integrated circuits that are useful for AI, the US can maintain its strategic advantage in the AI landscape.
Authors
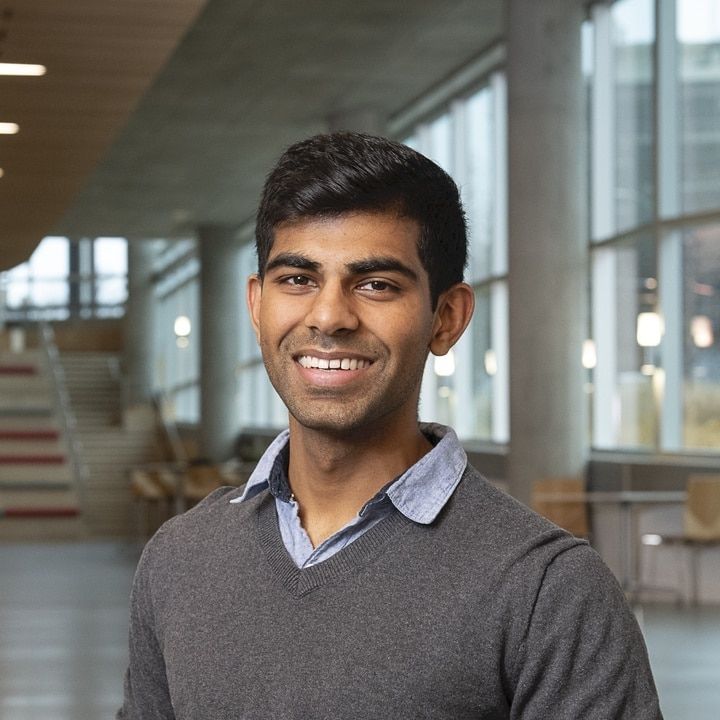
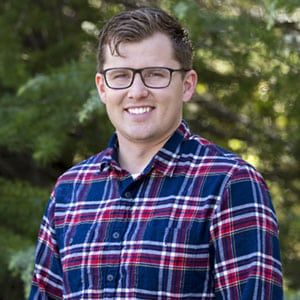