A New Contract for Artists in the Age of Generative AI
Eryk Salvaggio / Aug 25, 2023Eryk Salvaggio is a Research Advisor for Emerging Technology at the Siegel Family Endowment.
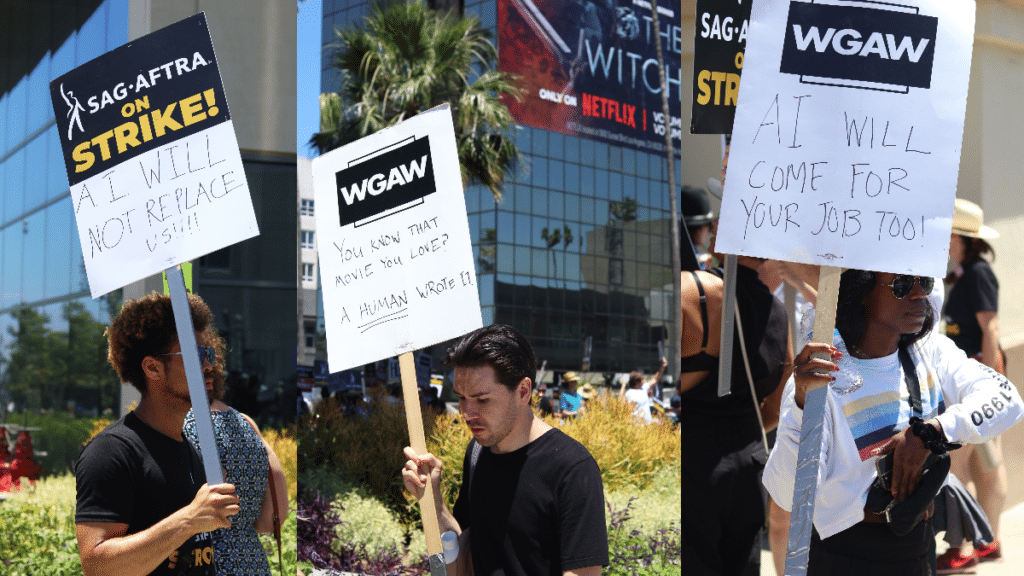
Generative AI has followed a familiar technological hype cycle. It has promised a transformation of how artists work, while threatening to end the careers of other creatives, such as those working in stock photography and copywriting. It could further disrupt, if not eliminate, creatives dependent upon commissioned illustration and concept art, video game art, and even voice-over artists.
The underlying driver of this shift is hard to grapple with. It doesn’t derive from what these models produce, but what they produce from: vast streams of creative writing, photographs, and drawings shared online. The authors of these works find themselves grappling with the reality that they have co-created a massive corpus of training data for the very AI platforms that would undermine their professions. To many, it feels as if their creative fruits have been harvested and blended by an algorithmic juicing machine without warning or consent. Where labor power is strong, such as screenwriters and actors, strikes by creative professionals are centering these concerns. But this isn't an option for independent, small-scale creatives who depend on the Internet to find clients and community.
The early promises of Creative Commons licensing made sense for the origins of the Internet. As an artist and writer, I was a stalwart advocate of Creative Commons. It was rooted in the flexibility of setting my terms for how others used my music, writing, and art. Later, I would work with foundations to write guides for students who wanted to share or use images from Wikimedia Commons. In those materials, I stressed that as artists and creators, students still maintained control over the use of their work. Commons licensing didn’t mean giving their rights away. It clarified their terms in advance and maintained flexibility to allow or refuse additional requests or uses. In return, students learned that just because something is on the internet doesn't mean it is free to use.
Share-Alike licenses were key to this control. If I made an image, I could append this license to the image (usually through adding some text to a caption) and link to the template. It made it clear that anyone who wanted to use these materials could do so for free, provided they also shared it freely and gave credit to the author. Additional degrees of licensing allowed even more specificity about the use of Commons licensed work: you could carve out a more generous allowance for non-commercial purposes, for example. You could decide if you would allow people to make alterations to that material or not. This way, you might upload photos of loved ones or personal creative work that you felt comfortable sharing, but didn’t want others to incorporate into new pieces.
Creative Commons was built on an early ideology of the internet, one which relied on the gift economy. This ethos built a strong ecosystem of content creators – first in spaces such as online forums, and later as the backbone of blogs, photo websites such as Flickr and Tumblr, and resources such as Wikipedia and Wikimedia Commons. It paved the way for a robust music-sharing scene, with hundreds of thousands of free “netlabels” rising up based on Creative Commons licensing. In short, the flexibility of these licenses allowed the early Web’s creativity and collaboration to flourish. Arguably, it contributed not only to a legal framework for protecting creative content, but also established norms of use that influenced even the gray areas of internet creativity: memes, digital collages, and musical mash-ups that could never exist through traditional licensing channels such as those used for record labels or magazines.
There would always be exceptions to Creative Commons licensing, because these licenses derived from copyright law more broadly. Some of these exceptions included fair use (or fair dealing), which allowed for satirical reappropriation and the use of data for producing research.
There are great reasons for these loopholes to exist – it makes researching hate speech in online communities possible, for example.
One of the largest datasets used in image generation is itself a research project: Common Crawl. This open dataset collects information about the World Wide Web for anyone to use, from academic researchers to businesses. The dataset includes Creative Commons licensed content, but also restricted and commercial content, with no distinction made for any of the underlying licenses: that is left to those using the dataset to dissect. In fact, the organization that manages Creative Commons licenses relies on Common Crawl to index content that uses those licenses.
How Generative AI Has Changed the Landscape
Then, something unexpected happened: Generative Artificial Intelligence systems, such as DALL-E 2, Midjourney, and Stable Diffusion, became publicly available. These technologies have blurred the line between research and commercialization for works found online in ways few could have anticipated even five years ago. Wikipedia is the backbone of large language models; Wikimedia Commons and sites like Flickr Commons are dominant in training data for image generation systems. These systems, however, strip away requirements around how material can be shared. They do not attribute the authors or sources, in violation of the share-alike clause.
What’s more, it’s not entirely clear that the images that these tools used to train these models were limited to Creative Commons licensed images. Common Crawl is the backbone of LAION 5B, a collection of metadata that stores URLs of images and their text descriptions. AI developers are quick to point out that there are no images in LAION 5B’s dataset that trained these models. But the information that these models rely on is nonetheless derived from publicly accessible images found online. And although the process for turning this metadata back into images – known as a Diffusion model – is complex, one thing is beyond dispute: without the internet’s data, sourced from thousands of people sharing their work online, these models could not exist.
As a research project, Diffusion models are remarkable. They can break images down into noise, learn how these images break apart, and then reassemble new images based on prompts. However, it’s crucial to recognize that the images and the text that drive this process originate from online contributions of creative content by human users. While the output of these AI systems might not look like specific things we would find online (with rare exceptions), they would not work without a strong open-source collection of data on which to train. Some of these are relatively harmless, such as the rough shape of a cat, or the texture of grass. Others are specifically human, as in requesting a still photo in the style of Wes Anderson or Alejandro Jodorowsky.
There is significant confusion among artists, policymakers, and even industry about how these images are used and transformed by this process. On the one hand, the output of these models can be considered transformative. On the other hand, the data that powers these models was collected under the auspices of being for non-commercial research.
These are not purely research projects; they are commercial ones. These models have been monetized by companies such as OpenAI (DALL-E 2), StabilityAI (Stable Diffusion), and Midjourney. Meanwhile, more private platforms are monetizing data-collection agreements with AI companies or asking users to agree to have their work incorporated into generative models. A consensus around “opt-out” agreements is beginning to emerge. However, these agreements place the responsibility on users that share their work online – who may not even be aware of generative AI, how it works, or that they can opt out.
The Tension Between Open and Private
Copyright is insufficient to understand these issues. We also need to consider the role of data rights: the protections we offer to people who share information and artistic expression online.
Generative AI is an opportunity to re-evaluate our relationships with the data economy, centered on a deepening tension between open access and consent.
On the one hand, those who want a vibrant commons want robust research exceptions. We want to encourage remixes, collage, sampling, reimagining. We want people to look at what exists, in new ways, and offer new insights into what they mean and how. On the other hand, those who want better data rights want people to be comfortable putting effort and care onto writing, art making, and online communication. That’s unlikely to happen if they don’t feel like they own and control the data they share, regardless of the platforms they use.
Another paradox is that generative AI models depend on a vibrant, thriving commons ecosystem to exist and improve: they need these images to be shared, to gather more data and improve their capabilities. Yet, their existence is likely to have a cooling effect on the use of Creative Commons licenses. If artists and writers feel uncertain about sharing their work with others, knowing that an AI might replicate their style. Sharing that work becomes significantly more fraught if every image an artist shares contributes to a dataset that automates their work. What, then, is the incentive to share that work online? Generated images cannot currently be copyrighted, ensuring a flood of public domain images that may quickly overwhelm the art made by the actual artist.
Today, Creative Commons licensing is falling short in ways that may have detrimental effects on the Commons as a whole. Many feel that the early promise of open culture movements to encourage creativity and collaboration has instead given rise to machines that exploit and compete with human livelihoods. Certainly, this is not what Creative Commons licenses – or copyright law – was designed to do.
There are several complicating factors at play. While it is true that no one artist makes a difference in a dataset of this size, every artist in the training data contributes to making these AI tools work. What can be done to shift this relationship back to the hands of human workers – the artists, photographers, and musicians who have built the online ecosystem by sharing their work online? Likewise, how might we preserve the flexibility of new media and digital artists who demand ethically designed generative media tools?
Rethinking the Sharing Framework
The Biden Administration’s Blueprint for an AI Bill of Rights calls for users to be “protected from abusive data practices via built-in protections” and to “have agency over how data … is used.” Our images and words, too, are “data about us,” protecting that expression is not just good policy, but supports the continued thriving of our online communities.
Yet, something seems disconnected in our conversation about generative AI when we can convert every personal interaction into “data.” Data is personal: it means things to people. The words we use to share our lives with friends on social media, the paintings and drawings we share online, the interactions we have with friends and family: all of it is data, and the public ought to have some say over how it is intercepted and transformed.
Though policymakers should consider AI-generated images under the lens of personal expression and allow the courts to determine the appropriate legal framework, the images these systems produce should not be the only area of focus. Copyright laws alone are a nebulous approach to protecting artists from the complex uses of their data for AI.
Instead, it is helpful to start at the source: how the data for these models is collected, and from whom? What mechanisms for consent are in place to ensure these contributions are informed and voluntary? This suggests a need for stronger laws regarding the use and sharing of data by the platforms that host these services. New guidelines should accommodate the following principles:
- Bring opt-out mechanisms to platforms where the data is shared. Buried in user agreements are clauses that give these platforms rights to train AI systems with users’ images (or text). If users aren’t comfortable with a platform's policy on AI, they ought to be informed and able to opt out without consequence.
- Acknowledge that people generate images – not AI. Every AI-generated artwork is a product of some human intention, and subsequent interaction, with a tool to manifest that intention. Attributing authorship to the tool, rather than attributing it to an interaction of users, tools, and data, wrongly shifts accountability to the tool instead of the humans who build and use them. Designers of these tools ought to be accountable for their decisions in building them. There are content moderation decisions already at work, such as the blurring or censorship of inappropriate images.
- Empower data consent. If users of a company’s tools or an open source model create a “dataset” of images by a particular human artist without permission, or include large numbers of an artist's work in a larger dataset, then the designers of those tools or models should be held accountable. There is an obligation to provide clear assurance that consent has been obtained for the data used in training datasets: just as one would in any ethical research endeavor.
- Affirm data rights as well as copyrights. There are limits to copyright law, which has long been flawed and over-restrictive to creative reinterpretation – and assumes that the court system is equally accessible to everyone. Placing the focus on the users who create outputs with AI tools is akin to faulting drivers when a vehicle malfunctions. Individuals who make and share AI-generated work should not be afraid of prosecution if these tools infringe another artist’s copyright: it is the responsibility of companies building these tools to ensure that consent is carried through in what their machines generate.
A new framework will enable greater control over the use of artists’ data when artists share their work online, whether it is made with pens, pencils, or keyboards. It would incentivize greater restraint on platforms that scrape, train, or sell datasets containing these works without consent. It would motivate the platforms building these tools to develop new ways to rigorously audit and defend against the nonconsensual inclusion of artists’ data, and perhaps to welcome open models that invite greater public scrutiny.
We shouldn’t be distracted by the claim that AI systems are somehow unknowable or too complex to understand. The relationship is simple: without human expression and creativity, these models wouldn’t exist. To protect the essence of open communication online, we should strive to make sharing feel safe and consensual. When that consent is violated, accountability ought to be placed where it belongs: at the level of the humans developing and deploying these systems. In the end, this framework will affirm the data and copyrights of artists, and create better conditions for training new AI systems on consensually shared data.
Authors
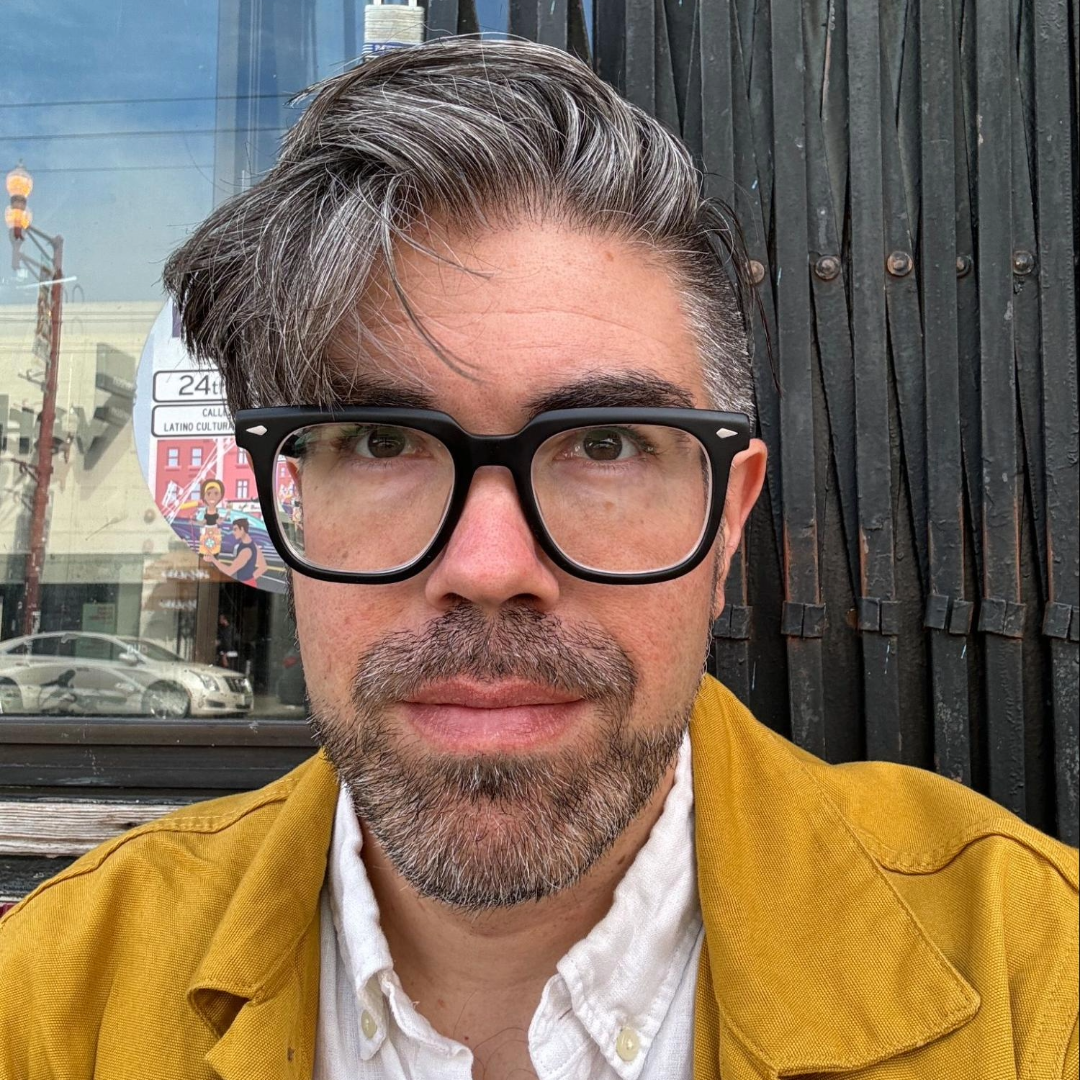