A Modest Ox: Examining Two Approaches to Testing Crowdsourced Fact Checking
Zeve Sanderson, Joshua Tucker / Dec 10, 2021Zeve Sanderson is the Executive Director and Joshua A. Tucker is one of the co-founders and co-directors of NYU’s Center for Social Media and Politics (CSMaP).
In the prototypical example of the “wisdom of the crowds,” statistician Francis Galton asked attendees at a 1907 country fair to guess the weight of an ox. Although nobody guessed the correct answer, Galton found that the average of the crowd’s estimates was off by only one pound. More recently, research has provided evidence for the crowd’s “wisdom” in domains ranging from forecasting current and economic events to predicting sports outcomes and weather.
This body of evidence has inspired social media platforms and scholars to consider whether a crowdsourced approach to fact-checking might help them identify misinformation at its current volume and velocity. Facebook, for example, has roughly 2 billion daily active users globally, relative to only a few hundred global fact-checking organizations. This structural challenge, while present in countries with robust fact-checking organizations such as the U.S., is even more acute in contexts with under-resourced fact-checking ecosystems. An effective crowdsourced approach, then, has the potential to dramatically increase the amount of information that could be verified around the globe.
The idea of using ordinary users as fact-checkers is appealing. Using the assessments from a representative sample of a platform’s users, for example, would likely protect against claims that professional fact-checking partners are biased against particular ideological viewpoints. It’s also scalable, can be more inclusive and representative, and moves difficult decisions about what is fact a step away from powerful platforms. But does it work?
Evaluating misinformation is not the same as gauging the weight of an ox. Misinformation operates in an antagonistic environment in which false stories are deliberately designed to trick users into believing their credibility. An ox, on the other hand, does not benefit from obscuring its weight. With Twitter and Facebook both announcing programs to include user assessments of news in their fact-checking systems, the question as to whether crowds can correctly assess news is of both scientific interest and practical importance.
On Monday, a piece in Time also kicked off with the anecdote of Galton and his ox as it summarized an important recent article, referred to here as Allen et al. (2021), published in Science Advances. The results of the study were overwhelmingly positive, suggesting that small, politically balanced crowds of ordinary Americans had similar rates of agreement to those of professional fact-checkers. And unlike fact-checkers who were asked to rigorously evaluate the entire article, the crowd was able to produce similar results by simply assessing the article’s headline and lead sentence (or “lede”).
While the study offers encouraging evidence for crowdsourced fact-checking, in a recent paper published in the Journal of Online Trust and Safety, we find reasons for caution when considering a crowdsourced approach.
Before we describe our study design and results, we want to emphasize the importance of both articles, which enable technologists and policymakers to consider a breadth of evidence with which to make product and policy decisions. Our aim here is not to adjudicate the studies, but rather to explain the choices we made in our research, explore what we think our results mean, and consider how others might engage with this important new area of inquiry.
In our study, we developed a transparent, replicable method for sourcing articles for evaluation that largely removes researcher choice from the process and instead selects articles based on their popularity over a recent time period. We set up three “streams” of low quality sources that have been identified in previous research as producing false stories: one stream of left-leaning low-quality sources, one of right-leaning low-quality sources, and one of low-quality sources without a clear ideological slant. Using a news aggregator, we identified, on a daily basis, the most popular article from each stream published in the previous 24 hours.
We then sent out these three articles to be evaluated both by professional fact-checkers from major news outlets and by roughly 90 American adults recruited through a survey firm. Over the 39 days of our study, we therefore collected 12,883 evaluations from ordinary people on 135 articles. Both fact-checkers and ordinary respondents were given 48 hours to complete the task, or within 72 hours of an article’s publication. Given that information on social media platforms is likely to spread rapidly but also die out relatively quickly, our study design was structured to collect evaluations in the period during which the articles were most likely to be consumed online.
A key challenge in this design was determining whether or not an article was false, as the stories were unlikely to have been evaluated by outlets such as Snopes or PolitiFact by the time they were included in our study. And although these articles were published by low-quality domains, previous research has shown that not all articles from suspect sources are indeed false. We therefore used the modal response of the fact-checkers to determine whether an article was false or not.
In addition, crowd performance cannot be assessed without a point of comparison. To this end, we constructed a professional benchmark, which was the frequency with which one professional fact-checker agreed with the modal response of the other fact-checkers. This measure is similar to the one used in Allen et al. (2021). By grouping together the respondents into hundreds of versions of crowds, we were then able to evaluate whether a crowdsourced approach could replicate the accuracy of fact-checkers.
In our analysis, we used both simple aggregation rules — the modal response from the crowd — and machine learning algorithms with information from the crowd as inputs. We also created crowds randomly sampled from all of our respondents, as well as crowds just with respondents who displayed high political knowledge. Our results?
Using simple rules, performance from crowds of randomly selected respondents and ideologically balanced respondents remained below 63% (compared to our professional benchmark of 72.8%). Crowds composed only of those who exhibited high political knowledge achieved 64%, the highest performance of these simple rules, though still far from the professional benchmark.
Using machine learning algorithms produced slightly better results. Most methods did not produce improvement over the simple rules. However, two methods (one with crowds of 25 ordinary people and one with 10 people with high political knowledge) achieved 68% performance, nearing the professional benchmark, which was 69.4% for this set of articles.
Taken together, our results suggest that crowds perform best when limited to those with high political knowledge and when using complex machine learning algorithms to aggregate responses. But no approach reaches the level of a single professional fact checker, a departure from the findings of Allen et al. (2021). And it’s important to note that we only asked professional fact-checkers to evaluate the articles using Internet research, rather than undertaking the typical laborious process that can include calling original sources. We would expect fact-checkers to have a higher benchmark if they undertook a more traditional process (which proved cost prohibitive for this project), giving even more cause for concern when interpreting our results.
When thinking about these two study designs, we identified three primary differences, though there were also many others that could have been important:
Article selection: Previous research has shown that, when asking ordinary people to evaluate the veracity of news, the sample of articles used in the study has the potential to significantly impact the results. Our sample was composed only of popular articles published by low-quality sources known to produce fake news. Allen et al (2021) used a set of articles shared by Facebook that its systems had identified as being potentially problematic, which included articles from both mainstream and low-quality sources. In preliminary unpublished research, we find that ordinary people are significantly better at identifying the veracity of news from mainstream sources as compared to low-quality sources. Therefore, the articles used across the two projects could explain much of the difference in the findings.
Temporality: We collected ratings of articles within 72 hours of their publication, often before fact-checking organizations or media outlets had the opportunity to evaluate the stories. Many of the articles used in Allen et al (2021) were months or years old and thus the veracity of the stories were well-established.
Full article vs. headline: In a separate paper that is under review, we find that people’s ability to evaluate the veracity of news is impactedby evaluating the headline / lede relative to evaluating the full article. We sent the respondents the full article, whereas the respondents in Allen et al (2021) study were only given the headline / lede.
Our purpose is not to argue for the appropriateness of one design over the other, but rather to highlight how the choices made in the study process may have impacted results. Given the potential implications of this work, we think a consideration of these differences are important for those who may use these studies to inform policy and product decisions.
This is an important new area of inquiry — one that pushes forward century-old questions in the behavioral sciences while supporting collective efforts to improve our digital information environments. Although the results of these two studies diverged, it is important to emphasize that our interpretations largely aligned: crowdsourced fact-checking, far from being a panacea to our so-called information disorder, could potentially be one tool in what certainly needs to be a much larger toolkit to discern facts in a complex ecosystem. Developing such tools will require the collaboration of scholars, technologists, civil society, and community groups.
Authors
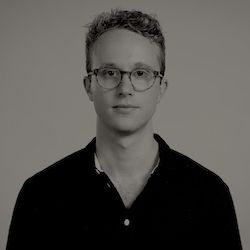
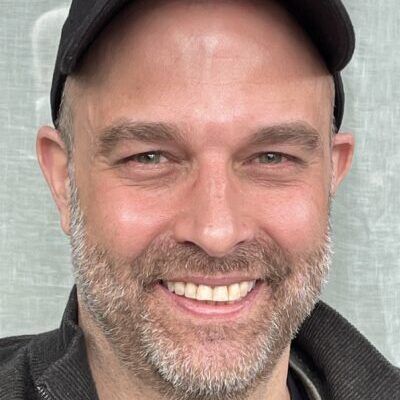