A History of Data from the Age of Reason to the Age of Algorithms
Justin Hendrix / Mar 19, 2023Audio of this conversation is available via your favorite podcast service.
At Columbia University, data scientist Chris Wiggins and historian Matthew Jones teach a course called Data: Past, Present and Future. Out of this collaboration has come a book, How Data Happened: A History from the Age of Reason to the Age of Algorithms, to be published on Tuesday, March 21st by W.W. Norton. It should be required reading for anyone working with data of any sort to solve problems. The book promises a sweeping history of data and its technical, political, and ethical impact on people and power.
What follows is a lightly edited transcript of the discussion.
Justin Hendrix:
Good morning. I'm Justin Hendrix, editor of Tech Policy Press, a nonprofit media and community venture intended to provoke new ideas, debate, and discussion at the intersection of technology and democracy. At Columbia University, data scientist Chris Wiggins and historian Matthew Jones teach a course called Data: Past, Present, and Future. Out of this collaboration has come a book, How Data Happened: A History from the Age of Reason to the Age of Algorithms, to be published on Tuesday, March 21st, 2023, by W.W. Norton. This book should be required reading for people working with data to solve problems. It promises a sweeping history of data and its technical, political, and ethical impact on people and power. I had the chance to speak with the authors ahead of the book's publication.
Matthew Jones:
I am Matthew L. Jones. I'm the James R. Barker professor of Contemporary Civilization in the Department of History at Columbia University.
Chris Wiggins:
So I'm Chris Wiggins. I'm an associate professor of Applied Mathematics here at Columbia, also affiliated with the Data Science Institute and also the chief data scientist at the New York Times.
Justin Hendrix:
You're the authors, of course, of How Data Happened: A History from the Age of Reason to the Age of Algorithms. Let's start just a little bit with your perspectives because you do bring two very different perspectives to this book. Matthew, maybe we'll start with you.
Matthew Jones:
Yeah, so I'm a historian of science, and I've had a long-term interest in how is it that mathematics came to have such a central role in the way we organize our societies. I worked a lot on figures from several hundred years ago, and, more recently, I've been interested in the radical transformations we've seen in the last, say, 40 years. And so, I bring reflections upon a moment in which the obviousness of turning to math is unclear, to look at our own moment where it's a constant reflex, a central way that administrators, business people, academics, and everyone else will regularly turn to all kinds of quantitative evaluation in everything we do.
Justin Hendrix:
Chris, what about you?
Chris Wiggins:
I would say I'm a technologist, so I actually am a practicing data scientist and also an educator. In terms of the way that I think about data, a lot of that is also influenced by the fact that I'm a citizen. I'm somebody who lives with my world. My professional world, my personal world, is shaped so much by algorithms. And so, as a researcher, part of my world is actually applying algorithms and using data science and machine learning and practice both for academic research and in industry, at the New York Times. Part of that is to try to understand that. How did it come to be that way, where our world is so much shaped by algorithms?
Also, as somebody who lives in academia, I really enjoy multidisciplinary science, which means I really enjoy the sort of discipline-transgressive act of publishing with natural scientists and statisticians and computer scientists. And the ways those different fields of ideas relate to each other is really not something that we spend a lot of time thinking about in academia. We're busy publishing papers and advancing the field, but how did it get that way? How did it come to pass that we use a word like "statistics," which was really about running the state, right? It doesn't have anything to do with data, the word "statistics." Or how did it come to pass that words like "data science" and "machine learning" and "artificial intelligence" are used so interchangeably? All of those terms had their own era and their own origins. And so part of my historical curiosity was to try to understand how that all came to be.
Justin Hendrix:
So you've matched up with a historian of science, and here we are. I find the way this book starts interesting. It's a history, but you don't start so much necessarily with history but rather with a kind of comment on the stakes around understanding data and its role in human society. Can you talk a little bit about that? Talk about that decision to start with the stakes and what you think the stakes are.
Matthew Jones:
We wanted, really, to set out why is it that you would want to go back in time? Why would you want to unsettle things that seem relatively settled? We wanted to set people up for not just a tale of, say, villains or heroes or other sorts of things, but a series of really consequential decisions and discoveries and organization. And so, we wanted to start with the stakes to understand what are the sort of concrete problems that people are facing now, often which are being treated as things that we're facing in a contemporaneous moment and understand that, when you look at things in the past, you begin to see how contingent, that things could be otherwise and indeed were otherwise. We wanted to prime the reader for a different kind of approach to history, a history which is about decisions, controversies, and non-obvious choices.
Justin Hendrix:
You bring up scholars like Hanna Wallach, Safiya Noble, Cathy O'Neil, talk about the extent to which algorithmic systems easily reproduce, in their automated judgements, the systemic inequalities of yore at an unprecedented rapidity and scale. Chris, anything else on this, on the stakes that you set out to address in this book?
Chris Wiggins:
There's two ways answering that. One is what are the stakes? The other is why do we start that way? Maybe I'll start with the second question, which sort of picks up on what Matt just said. There's a claim implicit in the book that a historical view is a useful view to all of us who are thinking about our present day. And so, by starting with present day, we're sort of putting a flag in the ground for where the through line is going to point. We're not claiming that we're going to take a specific time in history and go deep on that particular intellectual transition. Rather, we're starting out with the present-day stakes to say, "We're going to take an historical view that we claim will help the reader understand these particular contexts, present-day contexts, and the predicaments associated with the present-day context."
So in terms of the stakes, yeah, it's a feeling that more and more world is ruled by algorithms and data. It's sort of a loss of a feeling of control. It's, in some sense, an attempt to make sense of how it came to be that way and how these different intellectual threads tie together into present day. And those threads, we argue in the book, have centuries-long history even though it seems like, every day, there's a new headline, and that headline is somehow presented as free of context, like there's never been a chatbot before ChatGPT last month or something like that.
So part of what we're trying to do is to point out to people how useful it can be to be aware that there was a world before five headlines ago, and understanding that world can actually give you a lot of framework for understanding present day, where it's headed, similar contests of the past, and how resolution of those contests is totally relevant to the contests that are being fought right now.
Justin Hendrix:
You've already nodded to the idea that the sort of gathering of massive amounts of data was first a role for the state. You mentioned in the book that, of course, there are long traditions of collecting information, you say, about lands and peoples in China and Incan space, all over the world really. But you start the book, really, in the 18th century and focus really on the sort of enlightenment movement European states. Why do you start there?
Chris Wiggins:
Well, we're actually reading about it and teaching about it right now, so that's very much on my mind. Part of what's on my mind right now is it's true that people have gathered data to rule states for a long time. In fact, the origin of the word "statistics" sort of says to you that keeping data about a people is intimately related to ruling those people. In fact, if you look at books like James C. Scott's books, Seeing Like a State for example, there's an implicit argument there that you don't have a state unless you start enumerating people.
The reason we chose the period that we did, late 18th and mid 19th century, was this audacious idea that techniques from the scientific method and celestial mechanics and other fields that had really blossomed in the previous century or two, that those techniques could be used to understand societies and peoples. And so, what we start out with is a set of audacious attempts, mostly in the 1800s. By the end of the 19th century, people are taking techniques from the natural sciences, techniques like advanced, say, astronomy and celestial mechanics, and trying to use those same techniques for understanding people or psychology or intelligence, what even that means.
It's really a transformative time where people thought they could use that sort of scientific thinking and apply it to things that previously had been moral sciences. One of the readings we're teaching tomorrow has the word "moral" in it all over about pauperism, poverty, and crime. People started trying to take science and use it to understand these problems of society. It was really a transformative time in which scientific techniques that look completely contemporary to us today first were applied to a variety of different fields, including these fields that previously had been reserved as moral subjects.
Justin Hendrix:
You do introduce us to a Belgian astronomer. Tell us about him, Matt. Now, what was his role in doing a little bit of what Chris is talking to, getting us to a kind of social physics.
Matthew Jones:
Quetelet was a Belgian astronomer who wanted to build astronomy in Belgium. But along the way, in the sort of chaos following the French Revolution and its Napoleonic aftermath, he became really interested in how he could use all these new techniques for examining the stars that had really transformed astronomy. They were the pinnacle of French and German mathematics and astronomy of the time. And he was fascinated by what would happen if you began to use these same kind of tools to analyze all kinds of social problems and human problems.
So he took techniques, which, in some sense, were about questions of many people looking at an observation of a star, and began using them and examining individual people and then groups of people. And he began to introduce techniques that came out of the study of numbers and, in particular, of scientific concepts, and began to say that these might provide ways of gaining traction on understanding human phenomena such as crime or suicides or even characteristics like height and weight and other sorts of things.
So it's a vision that one could really apply these tools to these moral and social kinds of domains and that you could then understand them in a way distinct from individual human choice and reasoning. And then, above all, he was concerned with how is it that we might think of change that wouldn't be of a kind of destructive revolutionary character. His entire generation experienced the effects of the kind of revolutionary sort of violence, and he was envisioning how could we understand society differently and then how could we gradually transform it by transforming the characteristics of social groupings, which he often referred to in a 19th-century language of race.
Justin Hendrix:
Well, let's talk about that. You then moved to another fellow, Galton, who maybe takes this in a different direction, from surveying masses of people and trying to understand maybe that social physics to using the collection of massive amounts of data to understand individuals, target individuals, look for outliers and anomalies. Talk a little bit about that, the extent to which that leads us in perhaps some dark directions.
Matthew Jones:
So Galton very much picks up on Quetelet's notion of characterizing groups of people, but Galton had a particular kind of imperial concern. He was worried there weren't enough sort of great people in Britain to rule the empire and indeed to be the strongest polity on earth. So he's a cousin of the famous Charles Darwin and an exponent of a kind of process of selection of mates. So, as he looks into the question of how is it that different societies produce great people, he begins to use these new mathematical tools of people like Quetelet to how you characterize a group of people.
He comes to an insight about the question around why is it that, when parents have children, their offspring often aren't as awesome as they are, is the way we like to describe it to our students. They're lacking in awesomeness. Or things like they're not as tall, they're not as acute in sensibility. And in examining this problem, he comes to a incredible breakthrough, which is a mathematical technique called regression. Regression is an everyday technique that every scientist, almost every social scientist, we use all the time. Our children have scientific calculators that compute this. For Galton, it was initially a way to understand this phenomenon of why it is that, when you're trying to sort of manipulate a population, it's extremely hard because the outliers don't stay as outliers.
But along the way, and in pursuit of this policy, he comes to make central a fundamental way of understanding how two or more quantities relate and then a process of thinking about how they might be causally or other kinds of related. So his concern was what he branded eugenics, which was the improvement of the human species, which was to have and continues to have a very dark legacy around the world.
Chris Wiggins:
Just to draw those two subjects... So, your first question about the stakes. Part of what we're trying to do here is to say that there's historical antecedent to the desire to improve society, and you want to improve society using whatever is the latest tech of the day and often using all the data you can get your hands on.
And for Quetelet, and later for Galton, it's in large part what they were doing, was trying to take the latest technology available to them in the day and the data they could get their hands on by hook or crook -- and we talk quite a bit about how did they go about getting their hands on some data -- and then imposing on it a particular worldview and saying, "Okay, well, science tells us that this worldview is the right worldview because I've been able to take this worldview, express it in terms of whatever is the latest technology of the day," for example, the normal distribution or regression, whatever was the latest tech. And just because you take the latest tech and you impose into it your worldview doesn't mean that that somehow validates your worldview. That's what goes on in quite a bit of Quetelet and Galton, and is, I think, a good place for us to start our story.
Justin Hendrix:
And you do go on about data-driven racisms, how those intersect with the problems of modern society. I like this bit here. "Much of the history of statistics intertwines with the long, sorry tale of attempts to prove that social hierarchies rest on innate differences between people, whether differentiated by sex, race, or class. We've been duped time and again by such claims, which have persisted to our genomic age." You have an entire chapter on just the exploration of intelligence as a kind of quotient. What does our long fascination with that sort of tell us about data and its role in society?
Chris Wiggins:
I think a lot of it is the way that different fields get used as a mirror. Some new technology happens, and a lot of researchers ask, "What does that tell us about how we understand ourselves?" Intelligence is a good example. Part of what we talk about in last week's lecture in class, actually, is attempt to go about establishing various biases by just collecting numbers of things, for example, the size ofskulls of different demographic groups. This is well documented by previous authors, to be clear. A long, long history of phrenology and people just trying to measure the size of your cranium and then draw some conclusion from that.
All that failed quite miserably, and quantitative thinkers moved on to trying to figure out, "Well, let's try to get at intelligence in a more operational way," not just looking at the size of your skull, which turns out not to correlate with anything, but instead looking at some sort of summary statistic for how well you perform on various tasks. So, for Spearman, who were teaching tomorrow, actually, that was to make one summary statistic out of your performance, for example, your grades in university. So what he showed is not false, what I'm about to say, that, if you take sort of a point cloud of all of your grades and you do that for many different students, you'll see that they can be described well, as falling along one line in this cloud of different grades. And that is a thing which he made real, which is to say he reified it. And he reified it into a general number that quantifies your intelligence.
Sure enough, turns out that him and everybody else who scored well in classics had a high value on this number. And he said, "Therefore, I have captured what is true energy in your brain," so to speak, an actual physical meaning of your general intelligence factor. That went on to have all sorts of interpretations, none of which are at all borne out by the scientific process he went through. So, long before artificial intelligence, like 50 years before artificial intelligence, that's part of what we teach students is this history of people trying to take the complexity that is a human being and reduce it to one number in the form of an intelligence value.
Matthew Jones:
And this very much speaks to why begin in the 18th century. There's a long history here, but a limited history, of the prestige that comes with being able to claim science validates a particular conception of humans and of human hierarchy. So, what we see in the book is, time and again, efforts to do that, as in the case of intelligence and as happening in some quarters with the use of these data technologies today.
Chris Wiggins:
That's really part one of the book, is people looking at the world and then taking the latest technology of the day in terms of some novel mathematical methods and getting your hands on some data and then arguing that those new technologies or those new data sets somehow make your prior understanding of the world science. You've scientifically proven some sort of way of looking at the world. And it's pernicious and ever-present to the present day.
And so, having it be removed at a hundred years gives us a way of understanding it sort of in the abstract. But what we constantly want to do in every chapter is to remind people some of these habits of mind are quite persistent in our society, and we need to be reflexive of it when we ourselves are practicing these sorts of logical leaps. Also, we need to be critical when people are making an argument at us that seems to be backed by numbers.
Matthew Jones:
Yeah, and one of the things we try to do is to not only be critical of, say, the use of these scientific tools but to show, in many cases, the critics at the time are not anti-science. They are anti this way that science is used to offer a justification of existing phenomena. And so, we see Du Bois, the great American Black sociologist and thinker, pushing back, on purely statistical terms, about the wrong sort of inferences made by a particular, very influential gentleman. And I think that speaks to what Chris was just saying. So we want people to have a sort of critical take on the use of the newest Chinese technology, not to just bury it, at all, but, in some sense, to be much more reflective about the way we claim authority on the basis of it.
Justin Hendrix:
If part one takes us from that early history on through what you call the mathematical baptism -- so the emergence of many of the most important, I suppose, mathematical concepts related to data -- part two, really, we're in the 20th century. The importance of World War II, in particular, to the emergence of data and data science. Talk a little bit about that. What happened around the middle of the century? I was reminded, reading this, of the movie The Fog of War and Robert McNamara and the way he described the sort of relentless use of data and statistics in World War II, in the Allies' prosecution of the war.
Chris Wiggins:
We can only talk about so much in so many chapters, so we don't spend a lot of time on operations research, which is a field that, I think, really impacted McNamara, and the idea that, if you had enough data and you just sort of made an algebraic statement about what it is you're trying to optimize, you can always come up with the best policy. So part of what we try to do, and part one, alone, of the book, is to show how hard that is and to show how difficult it is to take something as complex as our realities or society or something like that and turn it into an algebraic expression of one sort or another.
The transformative thing, though, is that today... In part one of the class, a lot of the statistical work is happening outside of academia, certainly, and with just a small amount of mathematics. And our world today is a lot of technology and mathematics put together. So part of what we get at in part two is how did data become a concern of engineering? How did data become a form of technology? A large part of that is the transformation of World War II and the creation of special-purpose computational hardware. So a story that we tell, which was really a hidden story for several decades, is the story of how much of digital computation was borne on a data science problem, on a problem trying to make sense of streams of data -- namely, cryptography -- and trying to make crypto analysis the breaking of codes.
As a technologist, I would say it's an undertold story, how much of the technology was created at Bletchley Park, which we spend a lot of time in the book, and also, to be fair, among Polish mathematicians who even before Bletchley Park had shown British and French mathematicians how to break codes using special-purpose hardware.
Post World War II, though, you have the incredible financial support of the United States government and what comes to be called the military industrial complex funding the development of digital computation through IBM, through Bell Labs, through a bunch of other players, to create computation. So it's really that computation, which... I think, for many of us, we're introduced to this computation as thinking very clearly about logic problems and things like that. But a lot of computation was built by, motivated by, funded by attempts to deal with streaming data, often in the case of breaking codes. So that's part of the story that we tell and part of what we think is a context for making clear our present day. How did it come to be the case that we have technology that allows us to store and process such abundances of data?
Matthew Jones:
Yeah, at an industrial scale. So often, when you think of Bletchley Park, people think of Alan Turing, and that's not wrong. But it was only the conjunction of people like Turing combined with staff and machines that could handle large amounts of data. And that's really the transformative moment. How does that expand, then, in the secret world, in the military world, and then in the financial world, and that computation might be about large masses of data, not about, say, numerical calculation or merely logic or something like that, but really an industrial concern that's going to require those kinds of industrial resources and a workforce that's committed to that?
Chris Wiggins:
In part one, data is powerful because it's used as an argument for what is true. In part two of the book, as data becomes a technology deployed at scale, data is the power itself. One of the things that's useful in part two of the book is a discussion of when people push back on too much data power in the form of state power. So, part of the book is debates about privacy, mostly in the 1970s when individuals were very concerned about amassing huge amounts of data about people in the hands of very few parties. Only, the very few parties were the state. And a lot of our regulation that we benefit from today was really a benefit of people being very concerned about privacy. It's just they weren't concerned about companies having all the data. They were concerned about the state having all of those data.
Justin Hendrix:
You end part two with this section, The Science of Data, and I was surprised and perhaps delighted to find you opening that section with Allen Ginsberg and, of course, a quote from his poem Howl. Tell us about why in the world Ginsberg, at this point in the book.
Matthew Jones:
Because a famous data scientist, Hammerbacher, alluded to it in a moment, I think, of deep existential angst at the misfit between the sort of amazing new kinds of technologies that data science was producing and what its most dominant use was, which is getting people to click on ads. And so, Hammerbacher reflected on what's another moment of existential angst? Well, that classic Cold War poem of Ginsberg, which really got a sense of the misfit between the aspirations of a generation and what they were, in fact, doing. And so, I think it really captured a sense of this gulf between, as it were, the extraordinary reach and capacity of these new technologies and the incredibly narrow furrows into which they were largely being pushed by various kinds of corporate, governmental intelligence and other sorts of interests.
Justin Hendrix:
Let's move to part three and talk a little bit about, well, the current day, to some extent. You start off with the battle for data ethics, which I suppose we're living through. It seems to be playing out, at least on my Twitter feed, on a daily basis. You frame up what the kind of terms of this battle really are. Just speak to that for a moment.
Chris Wiggins:
By the end of the book, we want to get to a sense for where is power going. And to really frame that debate, we need to be slightly analytic about where are the sources of power. In the headlines, you'll look at something, some act performed by a private company, and people will say, "Okay. Well, we, as individuals, should do something about that, for example, by not using that company. Or the state, which represents us, should do something to limit the power of that private company." So we get there by the end of the book, in part, by starting with one of the sort of narrative framings of what does it mean for a company not to be bad to us? And that is under the term ethics.
So the battle for ethics is partly about that fight, over the last 10 to 15 years, as power has arisen in private companies. And individual citizens -- also members of the democratic electorate, by the way -- have looked at these private companies and say, "Well, these companies should stop being so bad." And people have tried hard to be more analytic about what they mean by being "bad." And for those of us who are coming from academia, there's a particular applied ethical tradition around being bad, around rights, harms, and justice, and trying to look at the role of scientific researchers and how they make sure that they're doing ethical conduct in their research, which has been partially, but not perfectly, grafted onto the way we talk about what these private companies do with our data.
After all, these private companies are run by technologists, often ex-academics or even people who go back and forth in the now-revolving door between academia and machine learning-driven companies. So that battle to define ethics and then to demand ethical behavior by tech companies is part of the milieu we try to make sense of in that particular chapter.
Justin Hendrix:
You talk about the history of ethics in science and how science essentially evolved a sort of method to deal with ethical failures in this section, from Tuskegee to Belmont. For the listener that doesn't know anything about Belmont or IRBs or the Belmont Report, can you give just a bit of context on that and why you think that framing's important, especially as we move into the current age?
Chris Wiggins:
Yeah. So the chapter on the battle for ethics opens up with two quotes, one from a lawyer who says, "I want to strangle ethics every time I hear about ethics from tech companies," which is motivated by a reasonable place. It's coming from a place that the word itself is used in a malleable way that allows those who are in power to define what ethics even means. And if you allow people in power to define ethics, then you will often end up in ways that don't actually advance what individual people who are thinking about, say, consumer protection mean when they start talking about the word "ethics."
So we open up with that quote and contrast it with a quote from the Belmont Report. The Belmont Report says these basic principles, among those generally accepted in our culture, are particularly relevant to the ethics of research involving human subjects, principles of respect for persons, beneficence, and justice. So the Belmont Report, in 1978, was trying to make ethics not only well-defined but actually a government specification for ethics. So we bring those out as two sort of ends on the spectra. One, ethics is something fuzzy, and anybody who wants to can get up and say whatever they think ethics is. And on the other end, an attempt, nearly 50 years ago, by the US government to make a government specification for ethics.
So there's a lot to unpack in the second of those stories, alone, namely, what did they do? How did they come up with a government spec for ethics? But the other is why would the government do that? And the reason that a government would do that is because of something so deeply racist, scientifically useless, funded by taxpayers, that the government was forced to create a government definition of ethics as well as a process for informing ethics.
So it's a great story for thinking about applied ethics, but it also does influence the way that academic researchers who move into these tech companies are thinking about ethics. That's sort of their background for framing applied ethics. So the story is that there was a US government-funded report -- sorry, not report -- research or experiment, I should say, for 40 years, I would say with or against the men of Tuskegee, Alabama, who overwhelmingly are Black, to study the disease syphilis. And they basically studied it by denying treatment to all of these men, including working to make sure they didn't get drafted because they would get treated, working to make sure they didn't find out that there was a treatment. There was absolutely no scientific value to this experiment, to be clear. It's called the experiment, but it's scientifically useless, totally, deeply racist. And it was so bad that when it started hitting the front pages of various newspapers in 1972, not only did they shut it down immediately, but Congress was compelled to put together a commission to make sure this never, ever happened again.
So part of what's going on there is a society -- in this case, a community, a whole country -- trying to come to consensus about what even do we mean by ethics? How are we going to define it? And then, how are we going to design a process to make sure that this never happens again in our name and with our money, our taxpayer dollars? So that background took a lot of work for some really smart people, and they worked hard to define it and to design a process for it, which, I think, is useful as a backdrop for seeing how ethics can be not just philosophy but an applied field that constrains people's decisions. And also, like I said, that is the backdrop from which people in the social sciences, in particular, that move into tech companies, they have that as part of their training, to think about ethics under that applied tradition.
Matthew Jones:
To give a sense of why it's such an important case study, it shows this challenge of dealing with different ethical demands. So, for example, if someone says to you, "Oh, well, let's not be Luddites about AI. We have to think about its long-term consequences for all of society." That's an example of a kind of form of reasoning that says what we do is the best for society taken as a mass. And that is in contrast to, say, a point of view that says we always need to enshrine the respect for each person taken as a being in themselves.
So Belmont says that's got to be fundamental to any consideration of an ethical situation, that you've got to think about both of those things carefully. And then -- and Chris articulated this earlier -- it's also attached to a sense that these ethical principles can't just be for the seminar room or a conference room, that they have to be empowered in some way. And in science that's funded by the US federal government, that's relatively straightforward, though uncomfortable for many scientists, which is that all scientific experiments involving a certain participation of human beings have to go through a very arduous and annoying, to many people, bureaucratic process. That bureaucratic process is the empowering of the ethical approach. And we don't necessarily say that is the right approach, but it's a combination both of a thickness of the ethical consideration with the way that it's actually embedded into institutions such that it has purchase. In the corporate setting, neither of those is particularly clear. Hence, a battle for AI ethics that we really want to get across.
Justin Hendrix:
And that's where you go next. You take us into the corporate world, the venture capital-fueled investment in tech, and, of course, in advertising-driven internet platforms. You talk about the consequences of the attention economy, questions around how our kind of mediated reality operates in this environment. So you take us from this type of consideration, perhaps of the bureaucratic or very heavily bureaucratically-laden consideration of ethics and possible harms to humans in experimental contexts that is present in science, and then we move into a context where there appear to, for many years, have been none of those types of considerations, which is the venture capital-backed Silicon Valley approach to tech. I don't know. My listeners are well familiar with, generally, the harms that we see play out on social media, the threat to privacy that is so often in the headlines these days. I don't know. Is there anything else to say about that? Do you find yourself, in this chapter, coming to any new conclusions about this present-day scenario?
Chris Wiggins:
I think there's so many things that are just overwhelming that, when I look at them, it's useful and fun to think, "What is new here, and what is old here?" For example, I invoke ChatGPT. So I can't think about ChatGPT without thinking about ELIZA, which is a chatbot that was originally created to satirize, well, artificial intelligence. It's a very, very simple rules-based chatbot. So the idea of chatbots have been around for a while, and it's fun to take something that's kind of disorienting, like a really good chatbot, and think, well, what's old here? For example, the ideas of chatbots that are artificially intelligent. And what's new here? For example, something that hoovers up abundance of data and, in principle, could be regurgitating text that's under copyright, for example, or text that includes personally identifiable information, in the same way that the analogous technique applied to images, we have seen over the last few months, could spit out things that are uncannily resembling licensed images that were used to train those tools.
So, for example, in the present day, I think there's a lot to be said. There's a lot to be said. One of the things we wanted to do with the ethical chapter was just to get analytic about what even do we mean by "bad"? In Belmont, they worked really hard to make a little hierarchically-organized ontology of unethical. Beneficence is about harms. Are you doing good? Are you doing bad? Respect for persons includes things like the idea of autonomy of the individual, informed consent. Are you not just doing something that's good for people, but have they really consented to have their data used in a way that, even though you're sure it's good, it's not real clear that it's ethical if you're not giving people a right to consent to that thing, even though you're real sure that you're not one of the baddies?
That sort of tension is part of, I think, a useful analytic provided by the framers of Belmont. And then, of course, justice, which includes fairness as one of our norms. But it's not just limited to fairness. And, by putting fairness as one of the subpoints of ethics, you can say, "Well, there's other ways to be ethical besides just making sure you're fair." So I think there's a lot to be said about ethics, and I think there's a lot to be said about the future. So, in our chapter on the future, what we try to show people -- we're writing a book, not a blog post, so want it to be useful in another three years -- is we try not to say, "Here's going to happen next week with Twitter's CEO, whatever." Instead, we try to say, "Here's a way to be analytic about power."
And we try to introduce it around state power, which, as I said earlier, we often often look to governments as though governments are the only source of restraint on private companies. The ways that private companies themselves regulate each other or effectively de-platform each other. And then individuals who perform a role, which, among legal scholars, is called private ordering, but, in the book, I prefer to just call it people power, an analogy to state power and corporate power. There's a lot to be said about all of those sources of power. And we rob ourselves of solutions if we just say, "Oh, only one of those is relevant." We have to wait for the corporations to regulate each other or de-platform each other, or we have to wait for the state to get its act together, or we have to wait for the employees at these companies to get their act together. If we lean only on one of those, we really rob ourselves of all the other solutions that come from the other two players in this unstable three-player game.
So that's part of the analytic framework that I hope our last chapter gives, in addition to pointing out things that are presently contested, Section 230 and other things that are still unresolved and don't seem likely to resolve in the near future, or GDPR and the ways that regulations are changing, led by Europe but now adopted by various states in the United States. We try to spend that chapter talking to people about how the game is still in play, right? It's way too early to give up, and it's way too early to predict how the movie's going to end. We don't know yet which powers are really going to dominate. The nice thing about the metaphor of a three-player, unstable game is there may not be one long-term solution. It may remain a chaotic contract among those three players.
Matthew Jones:
And just coming back to your prompt from the chapter on advertising and VC and things, if you think about, say, ChatGPT, what are the conditions under which a thing should come into being? There's a long list, scientific and other sorts of things. But one is a marriage of a rather lax approach to privacy and the use of other things that are just available on the web. Secondly, a certain kind of business model in which the enormous cost of building such a thing is entirely plausible within a certain kind of economic formation. It's not a sort of truth of nature that companies should be organized this way. It's really a distinctive part of US corporate law and development. You can't imagine it without that.
And then, finally, a story which has to do with that this actually depends on vast amounts of largely underpaid labor as well as sort of fancy computer programmers. The whole thing doesn't take off unless you have those kinds of combinations of certain laxity in law, certain corporate forms, and then certain kinds of corporate organization that are global in scope and that make it possible. I think you could take a lot of examples and think through the lenses that there's nothing eternal about lax privacy laws or certain corporate structures or global employment change. Those are all really distinctive things that make these things possible, both things that we really cherish and things that we find deeply problematic.
Justin Hendrix:
I was struck... In the State of the Union, the other night, Joe Biden referred to this idea of Silicon Valley's experiment on our children. So he was maybe adopting somewhat of the point of view that you've adopted in this book of thinking of it in those terms. But I do want to just maybe finish up on a set of questions I have or come back to in my own mind about what it is we're doing in fields like computational social science, the study of social media data, the study of speech on social media. It seems to be very much driven by all of those same motivations that you refer to being present in data early on, either ways to improve society, ways to better administer services, ways to control speech and argument and debate perhaps more efficiently, ways to see or predict certain harms occurring in society before they occur.
Are we still just sort of -- I don't know -- trying to do the same thing? Are we going to run into the same walls, perhaps, in the future? Or do you imagine, at some point, the study of data, the study of society, computational social science, the rest of these things will lead us to some sort of machines of loving grace? Is that where we're headed? Can you imagine that? Or has writing this book sort of led you to a different conclusion?
Chris Wiggins:
I can't help but think about Udny Yule, who we're teaching tomorrow. So it's a very interesting analogy that present debates about trying to apply science to the information ecosystem or problem du jour in the headlines and in academia has a lot of similarity to late Victorian England concerns around poverty and attempts to use science to try to make sense of that. So we're teaching tomorrow, among other things, this approach by somebody to try to predict how many people are in poverty in different regions of late Victorian England as a function of how much assistance people are getting and a handful of other variables using the best tech of the day, which was multivariate regression.
So that whole approach was hotly contested within academia. It wasn't like a scientist versus a non-scientist, as Professor Jones was saying earlier. It was really within the scientific community. There's a lot of debate on how even to make sense of that. And you definitely see that in the information ecosystem today. There are academics studying how information propagates, true and otherwise, and true and sort of in between. And there's many ways to quantify that. People are analyzing very different information platforms. The information platforms themselves have their own dynamics. The ways that you quantify true or not themselves can be contested and can lead to different results, depending on the subjective design choices.
And it is a time of great debate and, in some ways, friction and inefficiency. But I think that's what it looks like when people are trying to figure out what's true about a field that hasn't been quantified and hasn't yet sort of stabilized in terms of what is the right way, if any, to understand this complex real-world phenomenon. So, yeah, I would think there are some echoes there. I think, just because things don't look like a solved problem yet, doesn't mean that there's not room for really excellent scientific and scholarly and quantitative work in the field. Just because there's a mess now doesn't mean that the truth is not out there, so to speak. It just means that there's room for a lot of scholars to try to make these fields into stable fields of research. We're not there yet.
Matthew Jones:
Yeah. And I think one of the things that runs through the book is we don't a adopt a purely critical approach or a negative approach. Rather, we encourage, actually, a critical approach, that is, how do we reflectively use these for betterment? We begin by noting that some of the early statistical tools were used to push back on questions of social and economic division. There are, however, a lot of people who would have us use these in ways that would reinforce existing social disparity and power disparities.
And so, it's an important and crucial thing -- and we're very committed to this, both in writing this book and our teaching -- of thinking about how to teach people to use these tools in a very self-critical way. But also, part of that self-critical approach is to precisely recognize that there's very powerful interests that would have you draw conclusions that, in many cases, are not warranted. And so, the stabilization of this is going to involve, necessarily, both a negotiation of what are the scientific or technical limitations of looking at data, but also the conditions under which people are drawing those sorts of conclusions and their ability to use it in a careful way. In many cases, people are not in a position to use it in a careful way. Our collective endeavor has to both recognize the scientific limits and then to recognize the limits that are imposed by regulation, power, politics, and other sorts of things.
Justin Hendrix:
You end the book, by saying, "Technology means change, but societal change takes time. As we've seen, sometimes it takes decades for technology to get integrated into society before it comports with our values and norms, if it does at all. Many potential forces, large and small, are available to us, directly and indirectly, to shape the relationships among technology and norms, laws and markets, and data's role in it all." I think, if folks read this book, they'll have a better sense of all of those potential forces and the historical context for them. So I thank you both for writing it.
Matthew Jones:
Thank you so much, Justin.
Chris Wiggins:
Thank you. Yeah, thanks for having us, Justin.
Authors
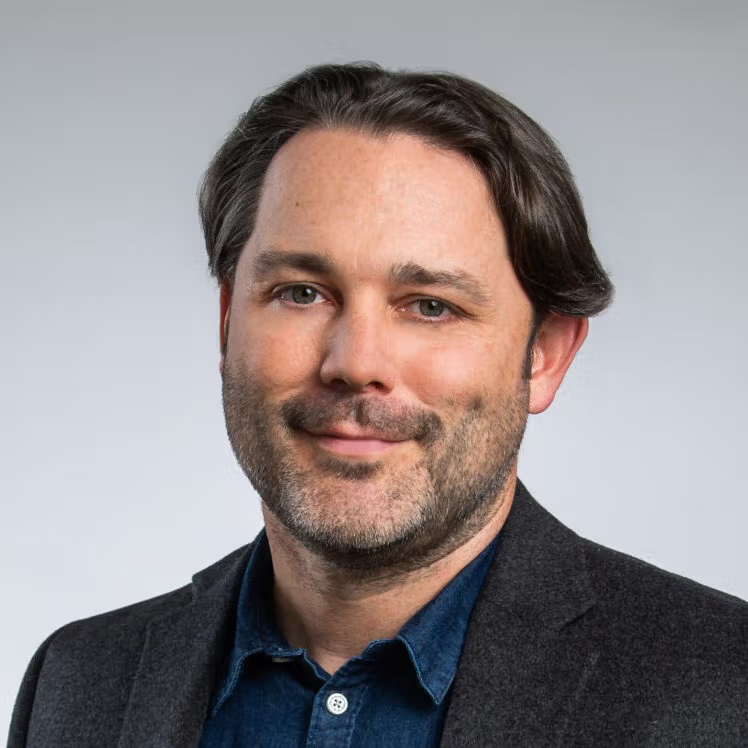