21st Century Snake Oil: The Consequences of Unregulated, Unproven AI
Sara Collins / Oct 13, 2021Imagine: you’re sitting at home, watching television, and you hear a knock on the door. When you answer the door, you see a uniformed police officer and a woman wearing a suit. The woman introduces herself as a social worker and explains that they are visiting you because an algorithm predicted that you would be “a party to violence.” The social worker gives you some brochures about assistance finding a job and seeking mental health care, while the officer explains that the police department will be “watching” you.
And they do watch you. You notice police cars following you as you walk to the local corner store, and your neighbors comment about how there always seems to be cops wherever you go. People get nervous around you and tensions start rising in your community. Some people accuse you of being a snitch. And, in your neighborhood, being known as a snitch can cause threats of violence or retaliation. So it happens, you are a “party to violence.” Someone from your neighborhood shoots toward you while you’re out running errands. In a way the algorithm was right, but only because the algorithm created the circumstances for the violence to occur.
This story might sound like a trite pitch for a Black Mirror episode, but it actually happened to one Chicago resident, according to a terrifying report by The Verge’s Matt Stroud.
I wanted to focus on this example, not because of the obvious civil rights harms or how this technology reinforces problematic policing practices, but because independent researchers ultimately found that the algorithm employed by the Chicago Police Department didn’t even work. Of course, this is not the only area where faulty algorithms are doing significant damage. From hiring algorithms to test proctoring software to predictive healthcare models, algorithms are operating without any evidence that they do what they claim to do: predict outcomes. Lawmakers and regulators need to take action to protect people from companies selling these unproven systems.
Arvind Naryanan, a Princeton professor of computer science, coined the term AI snake oil to describe artificial intelligence that works no better than a random number generator or a basic linear regression. He posits that because there have been certain areas of artificial intelligence that have made incredibly impressive gains over the past decade, there is a commercial incentive to make “AI” versions of everything. That’s how you end up with companies claiming their algorithms can predict job performance or recidivism, even though algorithms are incredibly bad at predicting social outcomes generally.
Another area where algorithmic tools perform poorly is emotion detection. Most emotion detection algorithms are based on facial expressions, and while facial expressions can be important indicators of emotion, they aren’t the whole story. Inflection and posture are also indicators of emotion. And most emotion detection algorithms don’t account for cultural differences. Even if those problems were fixed, there is little evidence to indicate that AI could ever be more accurate than humans at detecting emotions- and humans don’t always get it right, either.
The reality is that intuiting a person’s feelings or predicting job performance are both nearly impossible tasks for a computer. Algorithms cannot account for future extrinsic factors. People often employ non-linear reasoning and make decisions based on a network of past experiences and unconscious biases not easily captured by computer code. But tasking flawed algorithms with predicting human behavior has major flaws and serious consequences.
Although human decision-making skills aren’t perfect- people certainly introduce their own biases- one tangible benefit is that we can ask for an explanation. And when the explanation isn’t sufficient or forthcoming, we can attempt to challenge it. It’s not possible to “ask” an algorithm for an explanation in most circumstances. And attempts to incorporate humans into the AI decision-making process often results in the humans becoming rubber stamps, not meaningful mediators. This is because there is a perception that AI is smarter than humans. And that perception contributes to the difficulty in overruling an algorithmic system’s decision. Creating and refining algorithmic systems also become justifications for needing to collect and share more information about people, which creates its own separate privacy risks.
There is growing evidence that assigning algorithms the responsibility to make significant decisions about people is causing harm. Much has been written about the biases algorithms can codify and steps to mitigate the damage. However, detecting bias is difficult. If we could shift the burden to companies to demonstrate that their algorithms are safe and effective, we could greatly reduce the likelihood that biased algorithms enter the market at all.
For example, facial recognition’s trouble with detecting Black faces has been well documented. But facial recognition is not just used in surveillance cameras. It is also used in online hiring platforms, student proctoring software, and even for unemployment verification. Misidentification in these contexts has grave consequences. While this problem has often been framed in terms of bias, it also demonstrates a lack of effectiveness. If your software has trouble working on almost 15% of the population, your algorithm doesn’t do what you say it does. If you have an algorithm that is designed to allocate healthcare, but Black patients have to be significantly sicker than white patients in order to get the same level of care, your algorithm doesn’t work.
Unfortunately there is no requirement that companies demonstrate an algorithm’s effectiveness before putting it to use. The Algorithmic Justice and Online Transparency Act, introduced this year, has a requirement that algorithms used by online platforms be “safe and effective,” but that would only apply to sites like Facebook or Youtube, according to the proposed bill. Congress should consider expanding such protections to all products and services.
But even if Congress does not act, that doesn’t mean nothing can be done. Fortunately, the Federal Trade Commission (FTC) has a long history of prosecuting snake oil salesmen. Under Section 5 of the FTC Act, the commission has broad authority to go after companies that engage in unfair or deceptive acts or practices. This is why the FTC just sent a warning letter to purveyors of fake coronavirus cures, and how the FTC was able to fine companies that made scam weight loss products. In each instance these companies made claims about their products that weren’t backed by evidence. This same approach should be used for algorithms.
We could bolster the FTC’s enforcement capabilities with a digital regulator. The new agency’s responsibilities could include testing algorithmic and AI products to ensure that they are effective. Having an expert agency staffed with technologists, data scientists, and social scientists working on the problem of efficacy and ethics in AI would protect people from harm, while also advancing the state of the art. The coordinated approach of requiring testing before products go to market with enforcement for products that make false claims would significantly improve the current landscape.
As Congress, academics, and policy wonks continue to discuss how best to regulate the growing field of AI and automated decision-making, harmful technology cannot continue to go unaddressed. Regulators must step in and make sure consumers are protected from the worst of AI snake oil- before the next knock at the door.
Authors
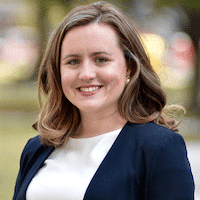